- 1Tianjin Centers for Disease Control and Prevention, Tianjin, China
- 2Huaian Center for Disease Control and Prevention, Huaian, China
Objectives: To construct an improved air health index (AHI) based on cardiovascular years of life lost (YLL) in Tianjin and assess its utility.
Methods: We derived the exposure-response coefficients from time-series models and calculated the excess YLL (EYLL) for simultaneous exposure to air pollution and non-optimum temperature. The AHI was developed using the EYLL at the WHO 2021 Air Quality Guideline annual mean values and optimum temperature as a reference. We assessed the validity of AHI by comparing the correlations and model fit between the AHI, air quality health index (AQHI), and air quality index (AQI) with cause-specific YLLs.
Results: Each inter quartile range (IQR) increase in AHI was associated with 256.31 (95%CI: 183.05, 329.57), 150.34 (95%CI: 108.23, 192.46), 90.41 (95%CI: 64.80, 116.02) and 60.80 (95%CI:33.41, 88.18) person-year increments for non-accidental, cardiovascular, ischaemic, and cerebrovascular YLL, respectively. The AHI, in contrast to the AQHI and AQI, showed the strongest correlations with the risks of cause-specific YLLs, both in the total population and subpopulations.
Conclusion: The AHI based on cardiovascular YLL has a greater predictive ability for health risks.
Introduction
In the context of global climate change, both air pollution and non-optimum ambient temperature have been recognized as serious environmental risk factors for human health, such as cardiovascular diseases and respiratory diseases [1–4]. The Global Burden of Disease Study in 2019 reported that air pollution was accountable for over 7 million deaths and tens of millions of disability-adjusted life years worldwide, with roughly 1.85 million untimely deaths and 42.51 million disability-adjusted life years resulting from air pollution exposure in China [5]. It has been estimated that non-optimum ambient temperature was responsible for 1.01 million deaths among men and 946 thousand deaths among women globally [5]. In addition, several studies have shown that air pollution and non-optimum ambient temperature could trigger comparable biological mechanisms in the induction of adverse health impacts, such as systemic inflammatory pathways, oxidative stress, and immune function [6, 7]. Therefore, simultaneously exposure to air pollution and non-optimum temperature may lead to greater health risks and burden of diseases, so that it is necessary to construct an indicator that could comprehensively assess the health risks of exposure to air pollution and non-optimum ambient temperature simultaneously.
At present, the air quality index (AQI) and air quality health index (AQHI) have been used in many countries and regions around the world to inform the public about the health risks associated with air pollution [8–10]. However, there are several limitations of these indicators. Firstly, as the AQI is a simple and easy-to-understand numeric scale ranging from 0 to 500 determined by the pollutant with the highest subindex, AQI may ignore other pollutants except the main pollutant, which means that it is unable to capture the cumulative influence of multiple air pollutants. Otherwise, the AQI fails to reflect the no-threshold exposure-response relationships between pollution and health outcomes. AQHI is an improved indicator which is constructed as the sum of the excess health risks associated with individual pollutants or mixtures of pollutants [11]. Several previous studies have constructed the AQHI and assessed its validity with the result that the AQHI is a better predictive indicator of health risks than the AQI [10, 12–15]. However, the current AQHI was established without taking the health effects of ambient non-optimum temperature into account, so it may lead to the underestimation while predicting the public health risks.
To address the limitation, the air health index (AHI) was first proposed in China by Professor Kan Haidong’s team [16]. This team, using a nationwide database, established the exposure-response relationships of major air pollutants and non-optimum ambient temperature with mortality, and constructed corresponding AHI by combining the excess health risks associated with air pollutants and non-optimum temperature on mortality. This study showed that AHI exhibited a good performance in predicting the daily mortality risks [16]. However, this study also pointed out two limitations of the AHI. Firstly, the predictability of the AHI may be less stable over a wide area, such as a country, than over a small region with homogenous atmospheric and demographic characteristics. In addition, it remains unclear whether AHI is a better indicator in predicting other health risks like cardiovascular diseases. The short-term effects of air pollution and ambient temperature on cardiovascular diseases have been studied in many regions [4, 17–19]. The statistically significant associations of air pollution and non-optimum temperature with cardiovascular diseases also were found in Tianjin [20, 21].
In addition, current AHI was constructed using mortality as a substitute for human health which giving equal weight to each death without taking into account the effects of age at death may result in a bias in forecasting air quality and health risks highlighted by the AHI. In contrast, years of life lost (YLL), which can take premature death and life expectancy into consideration and has been widely used as an indicator of health outcomes in the assessment of the effects of air pollution and ambient temperature [22, 23]. Therefore, in this study we chose cardiovascular YLL as an indicator of outcomes to establish an urban AHI in Tianjin by taking consideration of the effects of exposure to air pollution and non-optimum ambient temperature simultaneously.
Methods
Data Sources
The Tianjin Environmental Monitoring Center provided the daily average concentrations of PM2.5, PM10, SO2, NO2, CO, and O3, as well as daily AQI monitoring data, from 1st January 2014 to 31st December 2017 for six urban districts of Tianjin.
The daily average ambient temperature and relative humidity for the same period in the six urban districts of Tianjin were collected from the Tianjin Meteorological Bureau.
Data on mortality were collected from the Death Register and Report Information System of the Tianjin Centers for Disease Control and Prevention. We assessed a range of causes of mortality based on the sole primary diagnosis coded by the International Statistical Classification of Disease and Related Health Problems, 10th Revision (ICD-10), including non-accidental causes (A00-R99), cardiovascular diseases (I00-I99), ischaemic heart diseases (I20-I25), and cerebrovascular diseases (I60-I69). The cause-specific YLLs were calculated using the formula according to the WHO’s standard life table formula for YLLs. The formula has been described in detail previously [24]. The cardiovascular YLL was used as the health outcome to evaluate the exposure-response relationships of air pollution and non-optimum ambient temperature and to construct the AHI. The other cause-specific YLLs were used for the assessment of the validity of the AHI.
Estimating the Associations of Air Pollution With Cardiovascular YLL
The generalized additive models (GAM) and weighted quantile sum models (WQS) were fitted to establish the exposure-response relationship between air pollution and cardiovascular YLL.
First, single-pollutant GAMs were constructed to assess the exposure-response relationships between the six major air pollutants and cardiovascular YLL at different lag days. This aimed to identify the air pollutants that caused adverse effects and the lag days with the greatest health effects. The model is shown in Equation 1.
Where E(YLLt) represents the anticipated quantity of cardiovascular YLL on day t; α denotes the intercept; Xi,t-l is the concentration of air pollutant i on lag day l, taking into account single lags from the current day to the previous 3 days (lag0 to lag3), as well as cumulative lags from the current day to the previous 3 days (lag01 to lag03); β represents the exposure-response coefficients; ns(trend, 7*4) denotes a natural spline function with degree 7/year to control for the non-linear confounding effects of long-term and seasonal trends; templag03 and rhlag03 are the average values for average ambient temperature and average relative humidity from lags 0 to 3, s(templag03) and s(rhlag03) are penalized spline functions to control potential confounding effect of average ambient temperature and relative humidity, respectively [25]; DOW and Holiday are the dummy variables to account for the short-term cyclic fluctuations in the data.
Secondly, we determined air pollutants mixture according to the outcomes of the single-pollutant GAMs mentioned above and employed the WQS to examine the exposure-response relationship between air pollutants mixture and cardiovascular YLL. The model is shown in Equation 2.
Where β indicates the type number of air pollutants, with a maximum value of six; The
To estimate the model, the data were split into a training and a validation dataset. The former was utilized for weight estimation whilst the latter was used to test the significance of the final WQS index. The weights were assessed using a bootstrap approach and were restricted to sum to one and to be bounded between zero and one (
Estimating the Associations of Non-Optimum Ambient Temperature With Cardiovascular YLL
Our initial analysis, as presented in the Supplementary Material, using the distributed lag nonlinear model (DLNM) indicated a U-shaped relationship between ambient temperature and cardiovascular YLL in Tianjin, with possible cold and hot thresholds. Therefore, we constructed a double-threshold distributed lag linear model (DLM), which assumes that the impact of low temperature follows a linear pattern below the cold threshold, and the impact of high temperature is linear above the hot threshold. The model is shown in Equation 3.
Where TC and TH are matrices obtained by applying the double-threshold DLM to ambient temperature below the cold threshold and above the hot threshold respectively, the method for determining TC and TH has been described in detail in a previous study [28]; X are the air pollutants (the two air pollutants with the highest weight in the WQS), ns(X,3) is a non-parametric smoothing function of degree 3 to control for the confounding effect of air pollutants.
Constructing of AHI
Based on these exposure-response relationships obtained above, we calculated the daily excess YLL (EYLL) due to exposure to air pollution and non-optimum ambient temperature. The reference value of zero was utilized to calculate the excess risk associated with air pollutants. As air pollutants concentrations are standardized in the evaluation process of the WQS, it is essential that the reference value of zero is also standardized to prevent the occurrence of negative values in the calculation of the excess health risk. To calculate the excess health risk of non-optimum ambient temperature, cold or hot thresholds were used as a reference.
Then, using the annual standard values of each pollutant specified in the WHO 2021 Air Quality Guideline and optimum ambient temperature range as reference, the daily EYLL was indexed to a scale of 0–10+ to construct the AHI. Specifically, index value 3 corresponds to the EYLL derived from air pollution and ambient temperature that meet the annual standard values of the WHO 2021 Air Quality Guideline and the optimal ambient temperature range respectively. To evaluate the reliability of the AHI effectively, we also developed the AQHI, which takes into account only health risks caused by air pollution. And we categorized the AHI as low (0–3), moderate (4–6), and high (7–10+), based on prior research on air quality index grading [21].
Validity Evaluation of AHI
We assessed the validity of the AHI in predicting health risks by comparing the associations of the AHI, AQHI, and AQI with various health outcomes, including non-accidental, cardiovascular, cerebrovascular, and ischaemic YLL. The relationships between these three indexes and health outcomes in different subgroups were also compared. Because of the different numerical scales of these three indexes, AHI (0–10+), AQHI (0–10+), and AQI (0–500+), we used the inter quartile range (IQR) with increasing AHI, AQHI and AQI to assess the ability of the indices to predict health risks.
Results
Descriptive Analysis
(Supplementary Table S1) summarized the air pollution, meteorological factors, and health outcomes in Tianjin during the study period. The daily average concentrations of PM2.5, PM10, SO2, NO2, CO, and O3 were 71.97 μg/m3, 116.19 μg/m3, 28.02 μg/m3, 47.88 μg/m3, 1,606.44 μg/m3, and 58.04 μg/m3, respectively. The mean AQI was 105.45. The daily average temperature and average relative humidity were 13.94°C and 55.37%, respectively. The daily average non-accidental, cardiovascular, cerebrovascular, and ischaemic YLL were 1790.19, 1,102.27, 454.44, and 532.51 person-year, respectively.
The Associations of Air Pollution With Cardiovascular YLL
The results of the single-pollutant GAMs showed that PM10, SO2, NO2, and O3 had statistically significant effects on cardiovascular YLL in Tianjin (P < 0.05), and the maximum effect of each pollutant all occurred at the cumulative lag day (Supplementary Table S2). Specifically, the maximum health effects of PM10, NO2, and O3 occurred on lag01 with the effect values of 1.82 (95%CI: 0.01, 3.63), 6.99 (95%CI: 0.15, 13.82), and 4.34 (95%CI: 0.89, 7.81) respectively. The maximum effect of SO2 occurred on lag02 with the effect value of 12.91 (95%CI: 4.50, 21.31). Therefore, PM10, NO2, and O3 at lag01, and SO2 at lag02 were selected as the air pollutants mixtures for the subsequent analysis.
The result of the WQS was presented in Table 1, which showed the relative weights of each air pollutant in the air pollutants mixture, and the exposure-response relationship between the air pollutants mixture and cardiovascular YLL. SO2 had the largest weight of 0.447, followed by O3 and PM10 with relative weights of 0.327 and 0.179 respectively, and NO2 had the smallest of 0.046. It suggested that per unit increase in the concentrations of SO2, O3, and PM10 would result in greater health risks than NO2 in the air pollutants mixture. And the result revealed that the air pollutants mixture was also associated with an increased health risk of cardiovascular YLL. Each unit increase in the air pollutants mixture was associated with an increase of 30.41 person-year of cardiovascular YLL.
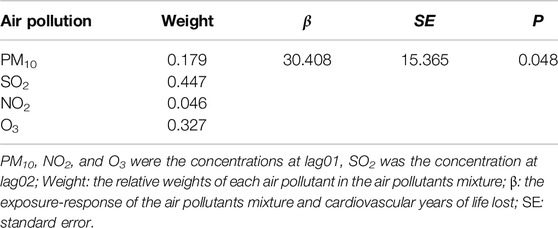
Table 1. The exposure-response relationship between air pollutants mixture and cardiovascular years of life lost in Tianjin, 2014–2017.
The Associations of Non-Optimum Ambient Temperature With Cardiovascular YLL
The DLNM showed a U-shaped relationship between average ambient temperature and cardiovascular YLL, with a large comfortable temperature range where the average ambient temperature has no statistically adverse effect on cardiovascular YLL. And the result indicated that the possible cold threshold was between −3.0°C and 3.0°C and the possible hot threshold was between 18°C and 23°C (Supplementary Figure S1). Therefore, multiple double-threshold DLMs were fitted to determine the combination of cold threshold (from −3.0°C to 3.0°C) and hot threshold (from 18.0°C to 23.0°C) with minimum residual deviation. We found that the cold and hot thresholds for cardiovascular YLL were 0°C and 19.0°C respectively (Supplementary Table S3).
Figure 1, basing a double-threshold DLM with 0°C as the cold threshold and 19°C as the hot threshold, showed the lag exposure-response relationships of low and high ambient temperature on cardiovascular YLL. Results showed that a significant cold effect on cardiovascular YLL appeared after a delay of 3 days and lasted for 18 days, whereas a significant hot effect of cardiovascular YLL occurred within 0–10 days. We calculated the overall effects of cold and hot on cardiovascular YLL along the lags (Table 2). For cold effect over lag 0–18 days, a 1°C decrease in ambient average temperature below the cold threshold was associated with a 44.68 (95%CI: 24.25, 65.11) person-year increase in cardiovascular YLL. For hot effect over lag 0–10 days, a 1°C increase in ambient average temperature above the hot threshold was associated with a 36.27 (95%CI: 22.83, 49.71) person-year increase in cardiovascular YLL.
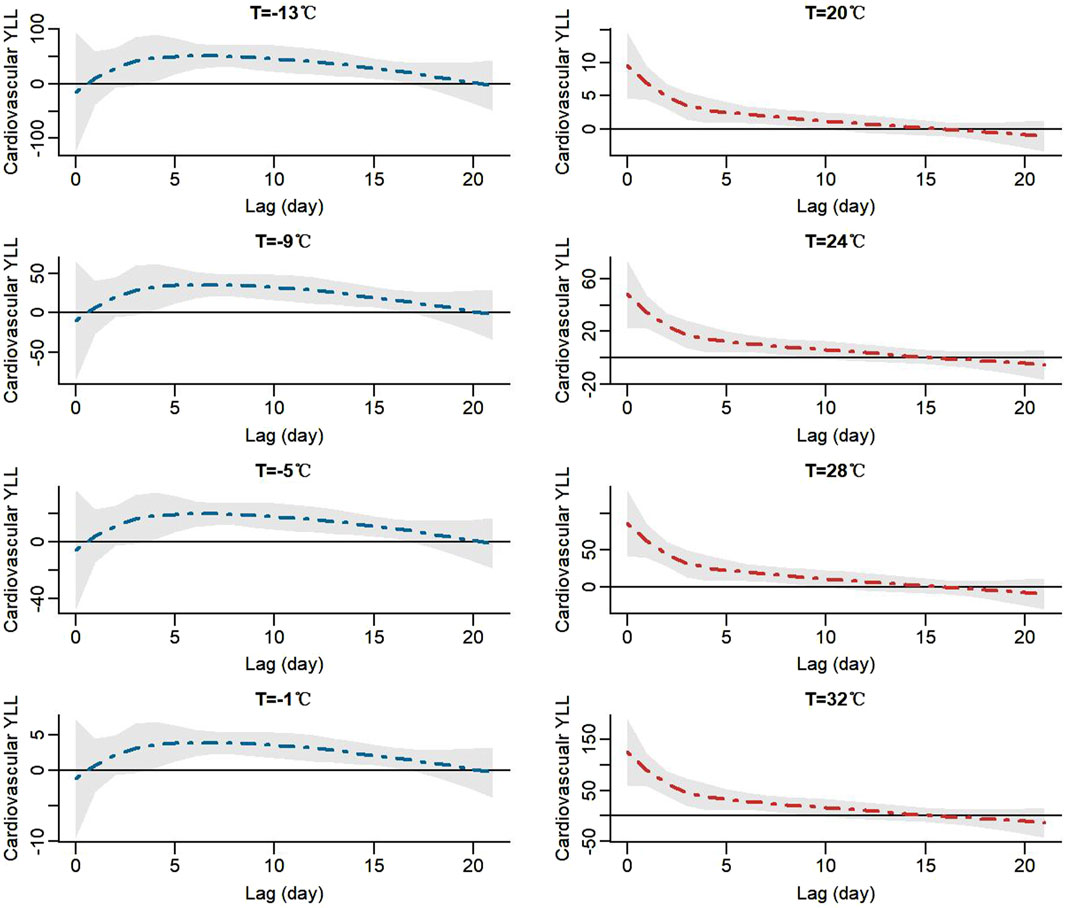
Figure 1. The lag exposure-response relationship of low and high ambient temperature with cardiovascular years of life lost in Tianjin, 2014–2017, using a double-threshold distributed lag linear model.
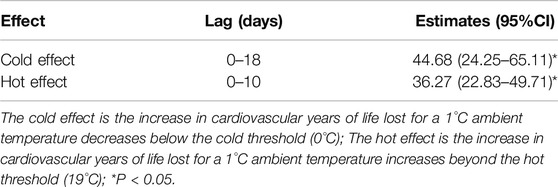
Table 2. The cumulative cold and hot effects of ambient temperature on cardiovascular years of life lost in Tianjin, 2014–2017.
The Calculation and Validation of AHI
The mean AHI was 15.60, the minimum and maximum AHIs were 2.47 and 44.61, respectively, and the IQR of AHI was 18.15. The mean AQHI was 6.12, the minimum and maximum AQHIs were 1.72 and 17.03, respectively, and the IQR of AQHI was 2.23. The mean AQI was 105.45, the minimum and maximum AQIs were 19.36 and 436.96, respectively, and the IQR of AQI was 63.09 (Supplementary Table S3).
Table 3 displayed the health risks of AHI, AQHI, and AQI associated with different health outcomes. AHI and AQHI were associated with increased risks of cause-specific YLLs, while no significant associations were observed for AQI. Each IQR increase in AHI was associated with 256.31 (95%CI: 183.05, 329.57), 150.34 (95%CI: 108.23, 192.46), 90.41 (95%CI: 64.80, 116.02), and 60.80 (95%CI: 33.41, 88.18) person-year increments for non-accidental, cardiovascular, ischaemic, and cerebrovascular YLL, respectively, whereas the increments were 45.42 (95%CI: 21.43, 69.41), 23.78 (95%CI: 9.13, 38.43), 15.93 (95%CI: 6.33, 25.52), and 4.88 (95%CI: 3.82, 13.59) for each unit increase in AQHI, respectively. And the AHI generally showed the strongest associations with the risk of cause-specific YLL, either in the total population or in subpopulations, compared with the AQHI and AQI (Figure 2).

Table 3. The relationships of air health index, air quality health index, and air quality index with daily cause-specific years of life lost in Tianjin, 2014–2017.
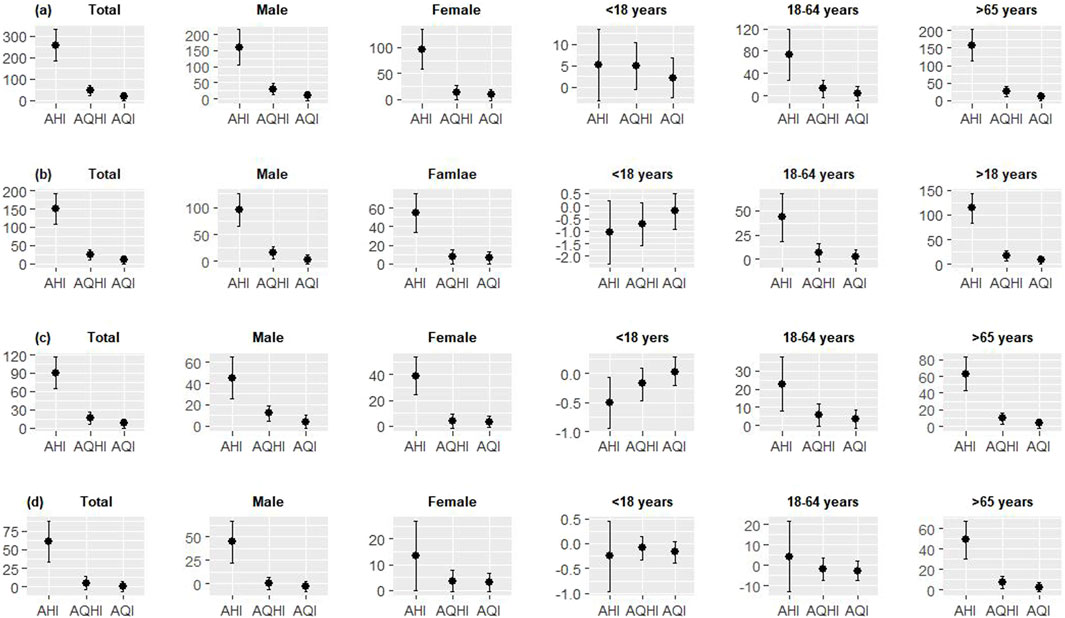
Figure 2. The relationships of per inter quartile range increase in air health index, air quality health index, and air quality index with daily non-accidental (A), cardiovascular (B), ischaemic (C), and cerebrovascular (D) years of life lost in the total population and subpopulations in Tianjin, 2014–2017.
The classification of AHI and health messages associated with each category of the index are shown in Table 4. 0–3 was classified as “low,” 4–6 as “moderate,” and 7–10+ as “high” marked with yellow, orange, and red, respectively. The primary health messages are to reduce the intensity and duration of outdoor activities, and to safeguard proper temperature conditions, for instance, by using an air conditioning system and dressing appropriately for the prevailing weather conditions.
Discussion
The AQI is a widely used index to characterize air quality and its health risks worldwide. It classifies air quality into classes based on the pollutant with the highest subindex (the top pollutant) with the disadvantage of not reflecting the additive health effects of multiple air pollutants. To address the shortcomings of the AQI, Environment Canada and Health Canada in 2008 proposed the AQHI, an index constructed based on the concentrations of multiple air pollutants and the exposure-response relationships between these air pollutants and health outcomes [11]. This provides the AQHI with a good performance in predicting the health risk of ambient air pollution.
However, within the context of global climate change, non-optimum ambient temperature is also a substantial environmental risk factor for human health. As a result, it is crucial to create an index that precisely reflects the health risks associated with non-optimum ambient temperature. Current indices measuring the health risks related to ambient temperature, such as the heat wave index and cold wave index, only account for extreme ambient temperature, neglecting the considerable health risks associated with moderate non-optimum temperature. Thus, the heat wave and cold wave indexes could significantly underestimate the health risks associated with non-optimum ambient temperature. The AHI incorporates the assessment of the exposure-response relationship between non-optimum temperature and health, as per the AQHI construction strategy [16]. This approach comprehensively reflects the health hazards linked to exposure to multiple ambient pollutants, along with effectively communicating the health risks of exposure to non-optimal temperature, which is highly significant for protecting public health.
In this study, we established the Tianjin AHI by integrating the health risks of ambient air pollution and non-optimum temperature with cardiovascular YLL and evaluated its validity. To the best of our knowledge, this study is the inaugural attempt at formulating a regional AHI whilst simultaneously utilizing cardiovascular YLL as a health outcome indicator. China is a large country with significant variations in atmospheric pollution, meteorological conditions, and population susceptibility across different regions. As a result, the predictability of AHI may be less stable in larger regions, such as the entire country, where atmospheric and population characteristics are less homogeneous. Furthermore, current health risk assessments require further differentiation of the disease based on health-risk groups [29, 30]. Short-term exposure to air pollution and non-optimum temperature could usually cause various diseases, mainly cardiovascular diseases and respiratory diseases [31–34]. Moreover, certain studies have demonstrated variations in the accuracy of air quality indices when evaluating diverse health risk groups [35]. It is therefore necessary to explore new indices that reflect the combined health risks of air pollution and non-optimal temperatures for different health risk groups, such as those with cardiovascular disease.
In addition, WQS was used to assess the exposure-response relationship between simultaneous exposure to major air pollutants and cardiovascular YLL in this study. WQS, developed explicitly for the context of environmental mixture analysis, is an increasingly widespread approach that enables the assessment of the association between a mixture and an outcome by generating a summary score of the mixture in a supervised manner [25, 26]. Because individuals are typically exposed to multiple simultaneously, and multiple air pollutants are often highly correlated with one another, WQS can more precisely evaluate the health risks of combined exposures to multiple air pollutants, compared to the traditional use of single-pollutant and two-pollutant models.
Furthermore, if Tianjin AHI is constructed according to Team Kan’s strategy, the AHI on any given day will be related to the day with the maximum pollution and non-optimal temperature observed during the 4 years in Tianjin. That arbitrary point of reference is not only irrelevant for the rest of the world but sooner or later also for the city of Tianjin. An index should have some generalizable dimension. Adebajo et al recently provided an intriguing point of reference to build a globally generalizable framework of air quality index, namely the WHO 2021 Air Quality Guideline annual mean values [21]. Therefore, we attempted to reconstruct the AHI using the WHO 2021 Air Quality Guideline annual mean values as a reference. The results showed that the majority of AHI is classified as “high risk” when using the WHO 2021 Air Quality Guideline annual mean values as a reference to construct the Tianjin AHI, with almost none falling under the “low risk” category. This indicates the necessity for further intensification of efforts to address air pollution and climate change in Tianjin, with the objective of protecting public health and reducing the associated disease burden.
Results demonstrated notable correlations between the AHI and non-accidental, cardiovascular, ischaemic, and cerebrovascular YLL, all of which surpassed those of the AQHI. No statistically significant correlations between AQI and these health outcomes were found. The GCV values for the AHI model were consistently lower than those for the AQHI and AQI models. Furthermore, the R2 values of the AHI model were uniformly higher when compared to the R2 values of the AQHI and AQI models. These findings affirmed that the AHI constructed based on cardiovascular YLL could comprehensively reflect the combined effects of air pollution and ambient non-optimum temperature in Tianjin and may be sufficient for predicting the health risks associated with air pollution and non-optimum ambient temperature in the total population and different subpopulations.
Previously, the team of Professor Haidong Kan classified the AHI index into six risk levels. In this study, we classified AHI into three risk classes based on Adebajo et al [21]. In a globalized world with people traveling it is not a good idea to use entirely different risk and color schemes to communicate the risks of air pollution and non-optimal temperature.
This study has some limitations. First, while constructing an AHI based on the exposure-response relationships of air pollution and ambient temperature with health risks has the potential to better predict daily health risks for the public, it should be noted that this construction strategy and model may only apply to the Tianjin area. Future studies should incorporate data from more areas and over a more extended time frame to validate the effectiveness of this strategy. Secondly, employing monitoring data directly from stationary monitoring sites on the ground as exposure data estimates for individuals in this study inevitably introduces measurement errors and might impact the validity of the AHI. Finally, as data from other sources have not yet been obtained, this study still employed data from 2014–2017 in the validation of the AHI, which represents a limitation of this study. In future studies, it would be beneficial to include data over longer time spans and on a wider range of health outcomes, such as rates of outpatient visits, hospitalisations, and the prevalence of various health symptoms, and so on.
Conclusion
In this work, we established an AHI based on the associations of six major air pollutants and non-optimum ambient temperature with daily cardiovascular YLL in Tianjin and evaluated the performance of the AHI in predicting daily health risks using data from 2014–2017. The AHI was found to be significantly associated with daily non-accidental and other cause-specific YLLs, and compared with the existing AQHI and AQI, the AHI had the strongest associations. And the AHI, in contrast to the AQHI and AQI, showed the strongest correlations with the risk of cause-specific YLL, both in the total population and in subpopulations. These findings highlighted that the AHI, constructed at the city level and based on cardiovascular YLL, has a greater predictive ability for health risks and has good applicability and application prospects to the public.
Author Contributions
All authors contributed to the study conception and design. Author MZ directed the implementation of the study, analyzed the data, and wrote the paper. Authors YB, JH, and YN helped to the analysis of the data and the revision of the manuscript. Author QZ designed the study and prepare and revise the manuscript. All authors contributed to the article and approved the submitted version.
Funding
The author(s) declare that financial support was received for the research, authorship, and/or publication of this article. The research was supported by grants from the Natural Science Foundation of Tianjin (grant number: 22JCQNJC00060), the Tianjin Health Research Project (grant number: TJWJ2021QN030), and Tianjin Key Medical Discipline (Specialized) Construction Project (grant number: TJYXZDXK-066B).
Conflict of Interest
The authors declare that they do not have any conflicts of interest.
Supplementary Material
The Supplementary Material for this article can be found online at: https://www.ssph-journal.org/articles/10.3389/ijph.2024.1607214/full#supplementary-material
References
1. Liu, C, Chen, R, Sera, F, Vicedo-Cabrera, AM, Guo, Y, Tong, S, et al. Ambient Particulate Air Pollution and Daily Mortality in 652 Cities. N Engl J Med (2019) 381:705–15. doi:10.1056/NEJMoa1817364
2. Arbuthnott, K, Hajat, S, Heaviside, C, and Vardoulakis, S. Years of LIFE LOST and Mortality Due to HEAT and COLD in the Three Largest English Cities. Environ Int (2020) 144:105966. doi:10.1016/j.envint.2020.105966
3. Hu, J, Hou, Z, Xu, Y, Zhou, M, Zhou, C, Xiao, Y, et al. LIFE LOSS of Cardiovascular Diseases Per Death Attributable to Ambient Temperature: A National Time Series Analysis Based on 364 Locations in China. Sci Total Environ (2021) 756:142614. doi:10.1016/j.scitotenv.2020.142614
4. Chen, R, Yin, P, Wang, L, Liu, C, Niu, Y, Wang, W, et al. Association Between Ambient Temperature and Mortality RISK and Burden: TIME Series Study in 272 MAIN Chinese Cities. BMJ (Clinical Research ed.) (2018) 363:k4306. doi:10.1136/bmj.k4306
5. GBD 2019 Risk Factors Collaborators. Global Burden of 87 RISK Factors in 204 Countries and Territories, 1990-2019: A Systematic Analysis for the Global Burden of Disease Study 2019. Lancet (2020) 396:1223–49. doi:10.1016/S0140-6736(20)30752-2
6. Wilker, EH, Yeh, G, Wellenius, GA, Davis, RB, Phillips, RS, and Mittleman, MA. Ambient Temperature and Biomarkers of Heart Failure: A Repeated Measures Analysis. Environ Health Perspect (2012) 120:1083–7. doi:10.1289/ehp.1104380
7. Xu, H, Wang, T, Liu, S, Brook, RD, Feng, B, Zhao, Q, et al. Extreme Levels of Air Pollution Associated with Changes in Biomarkers of Atherosclerotic Plaque Vulnerability and Thrombogenicity in Healthy Adults. Circ Res (2019) 124:e30–e43. doi:10.1161/CIRCRESAHA.118.313948
8. Tanday, S. India Launches Air Quality Index to Tackle Pollution Problems. The Lancet Oncol (2015) 16:e203. doi:10.1016/S1470-2045(15)70172-5
9. Mirabelli, MC, Ebelt, S, and Damon, SA. Air Quality Index and Air Quality Awareness Among Adults in the United States. Environ Res (2020) 183:109185. doi:10.1016/j.envres.2020.109185
10. Chen, MJ, Leon Guo, Y, Lin, P, Chiang, HC, Chen, PC, and Chen, YC. Air Quality Health Index (AQHI) Based on Multiple Air Pollutants and Mortality Risks in Taiwan: Construction and Validation. Environ Res (2023) 231:116214. doi:10.1016/j.envres.2023.116214
11. Stieb, DM, Burnett, RT, Smith-Doiron, M, Brion, O, Shin, HH, and Economou, V. A New Multipollutant, No-Threshold Air Quality Health Index Based on Short-Term Associations Observed in Daily Time-Series Analyses. J Air Waste Manag Assoc (2008) 58:435–50. doi:10.3155/1047-3289.58.3.435
12. Olstrup, H, Johansson, C, Forsberg, B, Tornevi, A, Ekebom, A, and Meister, K. A Multi-Pollutant Air Quality Health Index (AQHI) Based on Short-Term Respiratory Effects in Stockholm, Sweden. Int J Environ Res Public Health (2019) 16:105. doi:10.3390/ijerph16010105
13. Huang, WZ, He, WY, Knibbs, LD, Jalaludin, B, Guo, YM, Morawska, L, et al. Improved Morbidity-Based Air Quality Health Index Development Using Bayesian Multi-Pollutant Weighted Model. Environ Res (2022) 204:112397. doi:10.1016/j.envres.2021.112397
14. Xu, H, Zeng, W, Guo, B, Hopke, PK, Qiao, X, Choi, H, et al. Improved RISK Communications WITH a Bayesian Multipollutant Air Quality Health Index. Sci Total Environ (2020) 722:137892. doi:10.1016/j.scitotenv.2020.137892
15. Wong, TW, Tam, WWS, Yu, ITS, Lau, AKH, Pang, SW, and Wong, AHS. Developing a Risk-Based Air Quality Health Index. Atmos Environ (2013) 76:52–8. doi:10.1016/j.atmosenv.2012.06.071
16. Zhang, Q, Chen, R, Yin, G, Du, X, Meng, X, Qiu, Y, et al. The Establishment of a New Air Health Index Integrating the Mortality Risks Due to Ambient Air Pollution and Non-Optimum Temperature. Engineering (2022) 14:156–62. doi:10.1016/j.eng.2021.05.006
17. Orellano, P, Reynoso, J, Quaranta, N, Bardach, A, and Ciapponi, A. Short-Term Exposure to Particulate Matter (PM10 and PM2.5), Nitrogen Dioxide (NO2), and Ozone (O3) and All-Cause and Cause-specific Mortality: Systematic Review and Meta-Analysis. Environ Int (2020) 142:105876. doi:10.1016/j.envint.2020.105876
18. Bont, J, Jaganathan, S, Dahlquist, M, Persson, A, Stafoggia, M, and Ljungman, P. Ambient Air Pollution and Cardiovascular Diseases: An Umbrella Review of Systematic Reviews and Meta-Analyses. J Intern Med (2022) 291:779–800. doi:10.1111/joim.13467
19. Jin, J, Meng, X, Wang, D, Han, B, Wu, T, Xie, J, et al. Association Between Ambient Temperature and Cardiovascular Diseases Related Hospital Admissions in Lanzhou, China. Heliyon (2023) 9:e12997. doi:10.1016/j.heliyon.2023.e12997
20. Guo, Y, Barnett, AG, Zhang, Y, Tong, S, Yu, W, and Pan, X. The Short-Term Effect of Air Pollution on Cardiovascular Mortality in Tianjin, China: Comparison of TIME Series and Case-Crossover Analyses. Sci Total Environ (2010) 409:300–6. doi:10.1016/j.scitotenv.2010.10.013
21. Adebayo-Ojo, TC, Wichmann, J, Arowosegbe, OO, Probst-Hensch, N, Schindler, C, and Künzli, N. A New Global Air Quality Health Index Based on the WHO Air Quality Guideline Values WITH Application in CAPE TOWN. Int J Public Health (2023) 68:1606349. doi:10.3389/ijph.2023.1606349
22. Liu, T, Zhou, C, Zhang, H, Huang, B, Xu, Y, Lin, L, et al. Ambient Temperature and Years of LIFE LOST: A National Study in China. Innovation (Camb) (2021) 2:100072. doi:10.1016/j.xinn.2020.100072
23. Lv, LS, Zhou, CL, Jin, DH, Ma, WJ, Liu, T, Xie, YJ, et al. Impact of Ambient Temperature on LIFE LOSS Per Death FROM Cardiovascular Diseases: A Multicenter Study in Central China. Environ Sci Pollut Res Int (2022) 29:15791–9. doi:10.1007/s11356-021-16888-7
24. Zeng, Q, Fan, L, Ni, Y, Li, G, and Gu, Q. Construction of AQHI Based on the Exposure Relationship Between Air Pollution and YLL in Northern China. Sci Total Environ (2020) 710:136264. doi:10.1016/j.scitotenv.2019.136264
25. Gennings, C, Curtin, P, Bello, G, Wright, R, Arora, M, and Austin, C. Lagged WQS Regression for Mixtures WITH MANY Components. Environ Res (2020) 186:109529. doi:10.1016/j.envres.2020.109529
26. Guo, X, Li, N, Wang, H, Su, W, Song, Q, Liang, Q, et al. Combined Exposure to Multiple Metals on Cardiovascular Disease in NHANES Under FIVE Statistical Models. Environ Res (2022) 215:114435. doi:10.1016/j.envres.2022.114435
27. Adebayo-Ojo, TC, Wichmann, J, Arowosegbe, OO, Probst-Hensch, N, Schindler, C, and Künzli, N. Short-Term Effects of PM10, NO2, SO2 and O3 on Cardio-Respiratory Mortality in CAPE TOWN, South Africa, 2006-2015. Int J Environ Res Public Health (2022) 19(13):8078. doi:10.3390/ijerph19138078
28. Guo, Y, Barnett, AG, Pan, X, Yu, W, and Tong, S. The Impact of Temperature on Mortality in Tianjin, China: A Case-Crossover Design WITH a Distributed Lag Nonlinear Model. Environ Health Perspect (2011) 119(12):1719–25. doi:10.1289/ehp.1103598
29. Kousha, T, and Valacchi, G. The Air Quality Health Index and Emergency Department Visits for Urticaria in Windsor, Canada. J Toxicol Environ Health A (2015) 78:524–33. doi:10.1080/15287394.2014.991053
30. De Matteis, S, Forastiere, F, Baldacci, S, Maio, S, Tagliaferro, S, Fasola, S, et al. Issue 1 - “Update on Adverse Respiratory Effects of Outdoor Air Pollution.” PART 1: Outdoor Air Pollution and Respiratory Diseases: A General Update and an Italian Perspective: Outdoor Air Pollution and Respiratory Diseases: A General Update and an Italian Perspective. Pulmonology (2022) 28:284–96. doi:10.1016/j.pulmoe.2021.12.008
31. Irfan, H. Air Pollution and Cardiovascular Health in South Asia: A Comprehensive Review. Curr Probl Cardiol (2023) 49:102199. doi:10.1016/j.cpcardiol.2023.102199
32. Wang, H, Qian, G, Shi, J, Lu, W, Chen, Y, Fang, K, et al. Association Between Short-Term Exposure to Ambient Air Pollution and Upper Respiratory Tract Infection in Kunshan. Int J Biometeorol (2023) 68:189–97. doi:10.1007/s00484-023-02582-5
33. Zhang, Y, Luo, X, Ma, L, Ding, G, and Zhang, B. Effect of Ambient Temperature on Hospital Admissions for Respiratory Disease in Suburban Rural Villages of a Semi-Arid Region in Northwest China. J Occup Environ Med (2023) 65:1023–31. doi:10.1097/JOM.0000000000002962
34. Zhai, G, Tian, Y, Zhang, Y, and Zhou, W. The Effect of Ambient Temperature and RISK of Cardiovascular Disease Hospitalization in China: A Meta-Analysis. Int J Biometeorol (2023) 67:1423–33. doi:10.1007/s00484-023-02509-0
Keywords: air pollution, ambient temperature, air health index, years of life lost, cardiovascular
Citation: Zhang M, Bai Y, Hu J, Ni Y and Zeng Q (2024) An Improved Air Health Index Based on Short-Term Cardiovascular Effects in Tianjin, China. Int J Public Health 69:1607214. doi: 10.3389/ijph.2024.1607214
Received: 28 February 2024; Accepted: 20 August 2024;
Published: 16 September 2024.
Edited by:
Marloes Eeftens, Swiss Tropical and Public Health Institute (Swiss TPH), SwitzerlandReviewed by:
Two reviewers who chose to remain anonymousCopyright © 2024 Zhang, Bai, Hu, Ni and Zeng. This is an open-access article distributed under the terms of the Creative Commons Attribution License (CC BY). The use, distribution or reproduction in other forums is permitted, provided the original author(s) and the copyright owner(s) are credited and that the original publication in this journal is cited, in accordance with accepted academic practice. No use, distribution or reproduction is permitted which does not comply with these terms.
*Correspondence: Qiang Zeng, emVuZ3FpYW5naGFpeWFuQDEyNi5jb20=