- 1Institute of Medical Sociology, Center for Psychosocial Medicine, University Medical Center Hamburg-Eppendorf, Hamburg, Germany
- 2Heidelberg Institute of Global Health, Heidelberg University Hospital, Heidelberg, Baden-Württemberg, Germany
- 3NAKO e.V., Heidelberg University, Heidelberg, Baden-Württemberg, Germany
- 4Leibniz Institute for Prevention Research and Epidemiology (LG), Bremen, Germany
- 5Department of Epidemiology, Helmholtz Center for Infection Research, Helmholtz Association of German Research Centers (HZ), Braunschweig, Niedersachsen, Germany
- 6Institute for Medical Sociology, University Hospital of Düsseldorf, Düsseldorf, Germany
- 7Institute for Prevention and Cancer Epidemiology, University of Freiburg Medical Center, Freiburg, Baden-Württemberg, Germany
- 8Institute for Medical Epidemiology, Biometrics and Informatics (IMEBI), University Hospital in Halle, Halle, Saxony-Anhalt, Germany
- 9NAKO Study Center, German Institute of Human Nutrition Potsdam-Rehbruecke (DIfE), Potsdam, Brandenburg, Germany
- 10Division of Cancer Epidemiology, German Cancer Research Center (DKFZ), Heidelberg, Baden-Württemberg, Germany
- 11Institute of Social Medicine, Epidemiology and Health Economics, Charité University Medicine Berlin, Berlin, Germany
- 12Institute of Clinical Epidemiology and Biometry, Faculty of Medicine, University of Würzburg, Würzburg, Bavaria, Germany
- 13State Institute of Health I, Bavarian Health and Food Safety Authority, Erlangen, Germany
- 14Department of Clinical Epidemiology and Aging Research, German Cancer Research Center (DKFZ), Heidelberg, Baden-Württemberg, Germany
- 15Deptartment of Epidemiology and Preventive Medicine, University Medical Center Regensburg, Regensburg, Bavaria, Germany
- 16Institute for Community Medicine, University Medical Center Greifswald, Greifswald, Mecklenburg-Vorpommern, Germany
- 17Molecular Epidemiology Research Group, Max Delbrück Center for Molecular Medicine, Helmholtz Association of German Research Centers (HZ), Berlin, Baden-Wurttemberg, Germany
- 18Institute for Occupational and Maritime Medicine Hamburg, University Medical Center Hamburg-Eppendorf, Hamburg, Hamburg, Germany
- 19Institute of Epidemiology, Helmholtz Center München, Helmholtz Association of German Research Centres (HZ), Neuherberg, Germany
- 20Berlin Institute of Health (BIH), Charité University Medicine Berlin, Berlin, Germany
- 21Subject Sociology, University of Bamberg, Bamberg, Bavaria, Germany
- 22Leibniz-Institut für Umweltmedizinische Forschung (IUF), Dusseldorf, Germany
- 23Institute for Medical Informatics, Biometry and Epidemiology, Essen University Hospital, Essen, North Rhine-Westphalia, Germany
- 24German Institute of Human Nutrition Potsdam-Rehbruecke (DIfE), Potsdam, Brandenburg, Germany
- 25Institute of Nutrition Science, Faculty of Mathematics and Natural Sciences, University of Potsdam, Potsdam, Brandenburg, Germany
- 26Institute of Medical Biometry and Epidemiology, University Medical Center Hamburg-Eppendorf, Hamburg, Hamburg, Germany
Objectives: We analyze whether the prevalence of depressive symptoms differs among various migrant and non-migrant populations in Germany and to what extent these differences can be attributed to socioeconomic position (SEP) and social relations.
Methods: The German National Cohort health study (NAKO) is a prospective multicenter cohort study (N = 204,878). Migration background (assessed based on citizenship and country of birth of both participant and parents) was used as independent variable, age, sex, Social Network Index, the availability of emotional support, SEP (relative income position and educational status) and employment status were introduced as covariates and depressive symptoms (PHQ-9) as dependent variable in logistic regression models.
Results: Increased odds ratios of depressive symptoms were found in all migrant subgroups compared to non-migrants and varied regarding regions of origins. Elevated odds ratios decreased when SEP and social relations were included. Attenuations varied across migrant subgroups.
Conclusion: The gap in depressive symptoms can partly be attributed to SEP and social relations, with variations between migrant subgroups. The integration paradox is likely to contribute to the explanation of the results. Future studies need to consider heterogeneity among migrant subgroups whenever possible.
Introduction
The prevalence of depressive symptoms and adverse mental health is affected by recognized risk factors, including female gender, advanced age, lower socioeconomic status, and weak social connections [1, 2]. However, these factors may not act on their own but often in combination [3]. There is compelling evidence for a socioeconomic gradient in depression. It has been shown that lower levels of education, income or occupational status are generally associated with higher risks of depression and adverse mental health [4–6]. This association was found in different age groups [7–9]. There is also evidence for poorer mental health of populations in countries with higher income inequality [7–9] and for socioeconomic inequalities in treatment outcomes of depression [10].
Furthermore, social relations have been linked to both mental health and depressive symptoms. In their systematic review, Santini et al. identified different aspects of social relations to be associated with depressive symptoms. Highest risks for depressive symptoms were found for those who perceived their social support as inadequate [11]. Being socially isolated has also been identified as risk factor for depressive symptoms and adverse mental health [12].
Persons with migration background often carry higher mental health risks [13–15]. Possible reasons include socioeconomic disadvantage, harmful working and living conditions, experience of racism and discrimination and traumatic experiences in countries of origin, during migration or after arrival in host-countries [3, 16]. Various studies have shown that migrants and their descendants often report lower social integration and a lack of social support [14, 16, 17]. Social integration and social support enhance subjective wellbeing and can serve as a buffer against acute stress and adverse mental health effects [18–20]. Earlier studies found that social relations contribute to reduced mental health risks of migrants [11, 14, 21, 22]. In a Swedish study, Brydsten et al. [14] identified lack of social activity and low social support, among others, as key factors explaining higher mental health risks of migrants compared to non-migrants. Lecerof et al. [22] found that trust in others and social participation had a protective effect on mental health when migrant populations under study faced financial difficulties or experienced discrimination. The heterogeneity within migrants has often been neglected or not been analyzed, mostly due to reasons of data availability and the lack of including migrants in research.
People with migration background are not a homogenous group but instead consist of a broad range of nationalities, cultures, origins, languages, experiences and socioeconomic determinants [23, 24]. The concept and definition of migration background in Germany has been described in detail by Will [25]. Various historical, social and political peculiarities need to be considered in the history of migration in Germany. A general comparison of migrant versus non-migrant populations neglects these differences [23, 24, 26]. Most of the studies mentioned above regarding mental health gaps and the social disadvantage of migrant populations have rarely addressed the heterogeneity of persons with migration background [26–28]. In 2019, 21.2 million people in Germany (about 26% of the total population) can be classified as 1st generation migrants with own migration experience (about 13.7 million people) or as descendants of first-generation migrants who were born in Germany, so called 2nd generation migrants (about 7.6 million people; [29]). The largest groups are 1st generation migrant workers who came to Germany under political agreements between 1950 and the 1970s, especially from Turkey and countries in Southern Europe (Italy, Spain, Portugal, and Greece). Together with their descendants, they account for about 6.8 million people. Another frequent region of origin of migrants in Germany is Eastern Europe, especially from countries such as Poland and Romania. Among those with origins in Eastern Europe and the former Soviet Union are the so-called German resettlers, who automatically received German citizenship upon federal recognition as resettlers. About 4.6 million resettlers migrated to Germany since 1950 [29].
The aim of this study is to consider this heterogeneity in migrants and their descendants and to go into more detail regarding findings on mental health and their possible explanations among different groups of people with migration background in Germany. Our analyzes are based on data from the German National Cohort (NAKO), which, due to its size of more than 200,000 study participants, allows a differentiated analysis of the health situation of migrant populations in Germany [28].
Based on the aforementioned background, the following research questions are analyzed: (I) Are there differences in depressive symptoms between non-migrant and different migrant populations? (II) Can these migration-related differences in depressive symptoms be attributed to social integration, emotional support and socio-economic position (SEP)?
Methods
The NAKO is a prospective multicenter cohort study funded by the Federal Ministry of Education and Research, the federal states and the Helmholtz Association [30]. Main goal of the NAKO is to investigate risk factors and causes of common chronic diseases. Data collection and assessment is organized by 18 study centers across Germany, covering rural and urban areas with both high and low population density [28, 31, 32]. The NAKO included 204,878 participants aged 20–69 at baseline, based on random samples from data of the population registration authorities of the respective study centers, stratified by sex (1:1) and age (10.0% each 10-year group between 20 and 39 years, and 26.7% in each 10-year group between 40 and 69 years). The overall response at baseline was 17%, but varied between 9% and 32% across the 18 different study centers [33]. Baseline assessments took place from March 2014 until September 2019. These included face-to-face interviews, self-administered questionnaires, various physical examinations and the collection of biospecimens in all study centers, including whole-body 3T magnetic resonance imaging of about 30,000 participants. The wide range of assessments and the average data collection time of up to 5.6 h contributed to the 5 years time span of the baseline examination [33]. Further details on sampling and data assessment can be found in earlier publications [31, 32]. The sample used in the present analyses consists of 204,878 respondents, of which 34,108 can be classified as migrants. All participants provided written consent for study participation and all study centers’ local ethical committees gave their approval. The complete study was carried out in accordance with the Declaration of Helsinki.
Measures
Dependent Variable
Depressive symptoms were assessed using the Patient Health Questionnaire PHQ-9 [34]. The scale is well established and has been used in many other studies, including former research using the NAKO data [35, 36]. The PHQ-9 consists of nine items that relate to depressive symptoms during the past 2 weeks. Each item has a four-point-scale, ranging from 0 (“not at all”) to 3 (“almost every day”) resulting in a sum score with a range from 0 to 27. Cronbach’s Alpha for the PHQ-9 scale was 0.84, showing a high internal consistency of the scale. A validated cut-off score of 10 points or higher indicates depressive symptoms in terms of a moderate to severe depressive episode [37]. We used the dichotomized PHQ-9 variable as indicator for high (PHQ-9-score ≥10) or low (PHQ-9-score <10) depressive symptoms.
Independent Variables
Age and sex of respondents were recorded, where age was divided into three age groups (39 years and younger, 40–59 years, 60 years and older), as depressive symptoms are likely to vary in different age groups and to make results comparable across different risk factors included in the models [38]. Education and income were considered as indicators for socio-economic position (SEP). Education was assessed based on the ISCED-97 classification and recoded into three levels (“low” = ISCED-level 1/2, “intermediate” = ISCED-level 3/4 and “high” = ISCED-level 5/6) [39]. Equivalent household income was calculated based on information of the size of household and income, originally assessed with 16 income categories. Based on this information, relative income position was calculated on a 5-point scale and ranged from 1 (“below 60% of average equivalent income”) to 5 (“above 150% of average equivalent income”). This classification was based on the net equivalent household income from the European Union Statistics on Income and Living Conditions (EU-SILC). For this study, income position was recoded into three categories (“below 60% of average equivalent income,” indicating risk of poverty as defined by EU-SILC; “60%–150% of average equivalent income”; and “above 150% of average equivalent income,” indicating high income groups). As unemployment has been associated with effects both on social relations (e.g., in the work context) and income, we added employment status to our models. This variable indicated whether a respondent was employed, unemployed or non-employed. Pensioners fall into the latter category while self-employed persons fall into the employed status category.
Quantitative and qualitative dimensions of social relations were represented by the social network index measures (SNI, [40]) and emotional support. The SNI contains information on partnership (living with a partner), number of close social contacts (close friends and relatives) and membership in voluntary organizations. The SNI sums this information in a levelled score from 1 to 4, with lowest level 1 indicating social isolation [40]. The four-level SNI was dichotomized for the analyses (score of 1 coded as 1 and all others coded 0), with 1 indicating “social isolation” and 0 “not being isolated”. Emotional support was assessed by asking “Can you count on someone to support you emotionally (e.g., talk to you about problems, help you make a decision)?”. Based on the response categories, it was possible to distinguish to what extent help was needed at all and, if so, to what extent it was perceived as sufficient or insufficient. This variable was also dichotomized: The answers “no” and “yes, but would have needed more support” were recoded into “insufficient emotional support,” while “yes, but had no need” and “yes, and support was sufficient” were recoded into “sufficient emotional support”.
Migration was assessed according to a set of basic indicators for mapping migrant status in Germany [41]. We followed the proposal by Wiessner et al. for the NAKO data enabling more specific analyses and comparisons with non-migrant populations [28]. Migration status was recorded based on participants’ nationality and country of birth and their parents’ nationality and country of birth. This enabled a distinction between non-migrant populations and so-called 1st - and 2nd generation migrants. Based on this information, migrants were divided into two subgroups of 1st generation migrants, resettlers and one subgroup of 2nd generation migrants: Those who have another than a German citizenship; those who were born in another country than Germany but obtained German citizenship; those who can be defined as German resettlers, and those who were born in Germany and have at least one parent with non-German country of birth (2nd generation migrants) [28]. Information on countries of birth of study participants and on countries of birth of their parents were used to generate a variable on regions of origin, based on the 15 regions defined by the United Nations [42], in order to enable more specific analyses and to identify possible heterogeneity within migrant populations. In 1st generation migrants their own country of birth was used for generating the region of origin variable, in 2nd generation migrants the country of birth of the parents was used. When both parents had different countries of birth from different regions, country of birth of the father was used for generating the region of origin variable. The three most common regions across the four different migrant subgroups and their descendants were selected, while the 12 remaining regions were coded as “other” category (see Table 1).
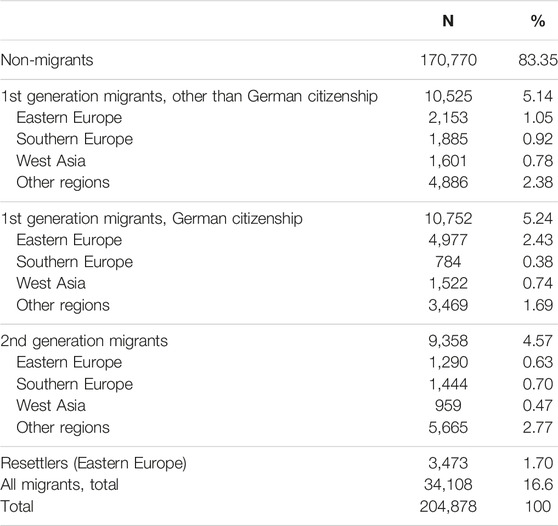
TABLE 1. Sample description according to migration status and largest regions of origin (German National Cohort (NAKO), Germany, 2014–2019).
Missing Data
On average, about 6.5% of individual items across all variables were missing. The variables sex and age had no missing values, while the highest proportion of missing values were found in the variables emotional support (16.3%) and SNI (16.6%). The missing data pattern was analyzed, and missing data was imputed using the multivariate imputation by chained equations method [43]. The method for imputing missing values depends on the variable’s nature. For continuous variables, predictive mean matching was applied, while logistic regressions were used for binary variables and polytomous logistic regression for categorical variables with more than two levels.
Statistical Analysis
Sample description is reported in terms of means and standard deviations for continuous data and proportion for categorical variables. Results are shown for each migrant group separately and for the total sample. Two logistic regression models with PHQ-9 (dichotomized) as dependent variable and migration as independent variable were calculated, each with a different set of further covariates. In the first model, we included age and sex as covariates. The second model introduced all variables simultaneously and thus included migration, age, sex, SEP indicators (education, and income positions), employment status and social relations indicators (emotional support, SNI). Analysis of collinearity revealed no problems, neither for education and income nor for other control variables used in our models.
We then calculated two more logistic regression models that included the specific regions of origin for different migrant subgroups, which generally replicated the initial models, in order to investigate differences between them and their association with depressive symptoms. For all models, Nagelkerke’s pseudo-r-squared is reported. All analyses were carried out using the R statistical package [44].
Results
16.6% (n = 34,108) of the NAKO sample can be categorized as migrants or their descendants (see Table 1). Further specification revealed that 5.1% (n = 10,525) are 1st generation migrants with other than German citizenship, 5.2% (n = 10,752) became German citizens after birth, 4.6% (n = 9,358) are 2nd generation migrants (German-born with at least one parent of other than German origin) and 1.7% (n = 3,473) are German resettlers. When looking at regions of origin, Eastern Europe (e.g., Poland and Rumania), Southern Europe (e.g., Italy) and West Asia (e.g., Turkey) were the three regions of origin named most often across all different migrant categories.
14.3% of 1st generation migrants with non-German citizenship reported 10 years or less of education, while in the non-migrant population only 1.7% do so (see Table 2). A similar trend was found in relative income position. For example, 37.1% of 1st generation migrants with non-German citizenship were attributed to the lowest income position, thus being at risk of relative poverty, while 12.7% of non-migrants were at risk of relative poverty. The proportion of respondents reporting unemployment was highest in migrants and their descendants, with 14.3% of 1st generation migrants with non-German citizenship reporting unemployment. When looking at the two indicators of social relationships, three out of four migrant groups under study showed higher risks of being socially isolated (with the exception of resettlers) and a higher percentage of inadequate emotional support compared to non-migrants. Lack of emotional support was reported by almost every fifth 1st generation migrants with non-German citizenship, social isolation was reported by 17.7% of 1st generation migrants with non-German citizenship. An increased burden of depressive symptoms was detected in all migrants and their descendants compared to non-migrants in Germany. This was true for mean scores of the PHQ-9 questionnaire as well as a score of 10 or higher, indicating the presence of moderate to severe depressive symptoms.
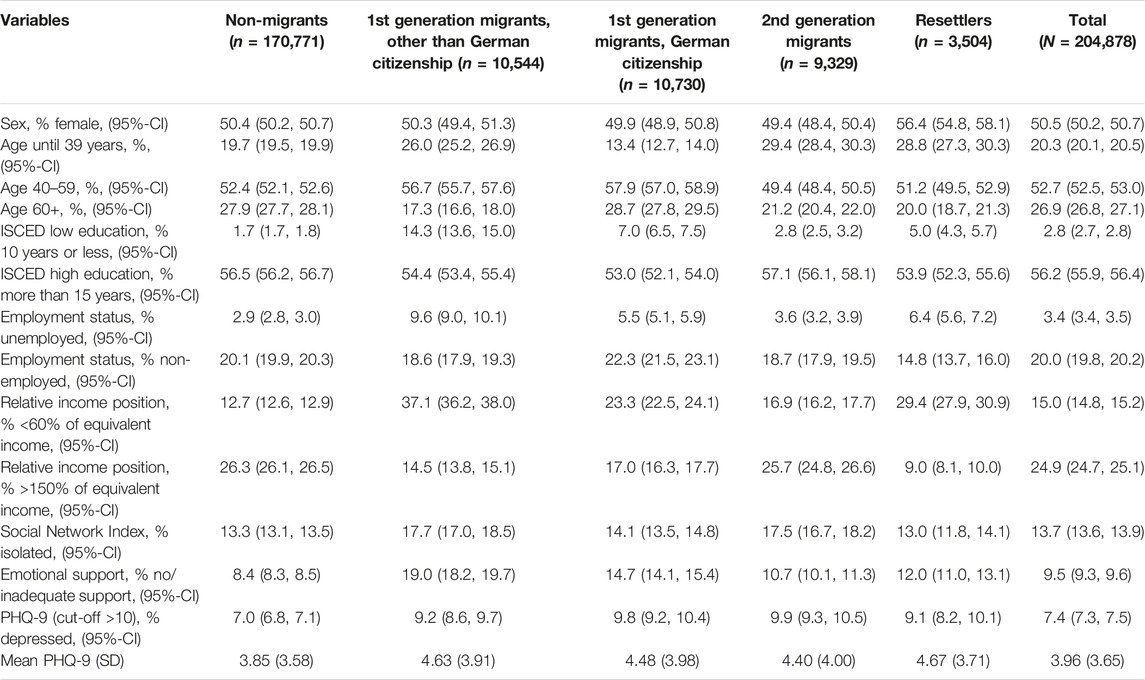
TABLE 2. Descriptive statistics of indicators used in the analysis; baseline data (German National Cohort (NAKO), Germany, 2014–2019).
In terms of research question one, results showed significantly increased risks of reporting depressive symptoms for all migrants compared to non-migrants (see Table 3, Model 1). Highest risks were found for both groups of 1st generation migrants (German citizenship OR 1.57; other than German citizenship OR 1.51). Regarding research question two, we found that after controlling for social relations and SEP, in two out of four migrant groups significantly higher ORs remained compared to non-migrants. In non-German citizens and resettlers, the increased risks of reporting depressive symptoms decreased or reversed while this was not the case for those who obtained German citizenship after birth (OR 1.18) and for descendants of migrants (OR 1.28).
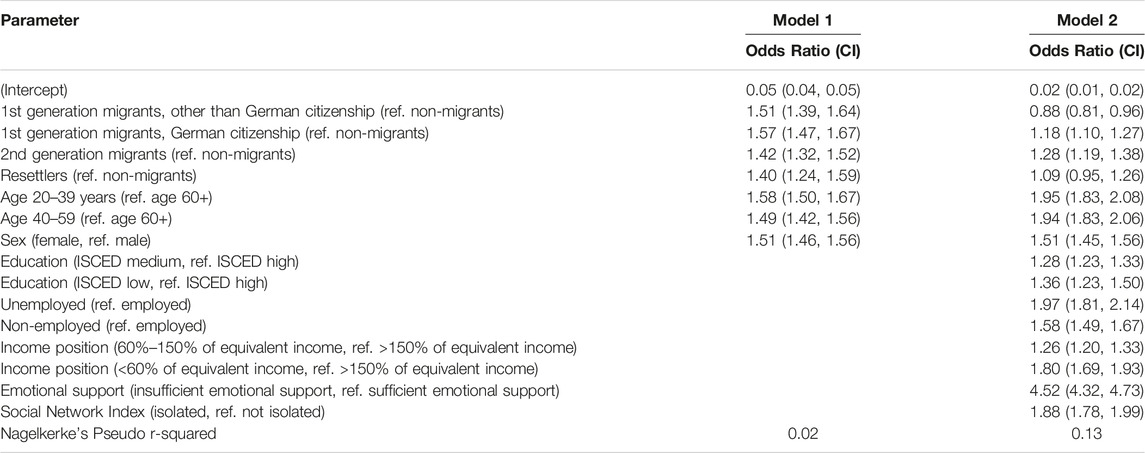
TABLE 3. Logistic regression models to examine associations of different migration characteristics with depressive symptoms (dichotomized PHQ-9, 1 = score ≥10 indicating depressive symptoms); N = 204,878 (German National Cohort (NAKO), Germany, 2014–2019).
In Table 4, migrants and their descendants were further differentiated by region of origin. Resettlers were not included (see results in Table 3) as their region of origin is mostly Eastern Europe, with little exceptions. Compared to non-migrants, migrants and their descendants with origin in West Asia showed highest odds of reporting depressive symptoms with odds ratios ranging from 2.17 to 2.70. Subpopulations with origin in Eastern Europe and Southern Europe showed comparably less elevated odds. While for those 1st generation migrants without German citizenship the introduction of SEP and social relations led to a reduction and even reversal of risks compared to non-migrants, especially with origin in West Asia, this was not the case for 2nd generation migrants with origins in West Asia. After introducing both SEP and the two indicators of social relations, odds for depressive symptoms in 2nd generation migrants with origin in West Asia remained more than two-fold compared to non-migrant populations (OR 2.15; Table 4, Model 2). Similar results were found for 1st generation migrants with German citizenship with origin West Asia, even though the odds ratio after controlling for both social relations and SEP was comparably lower (OR 1.42).
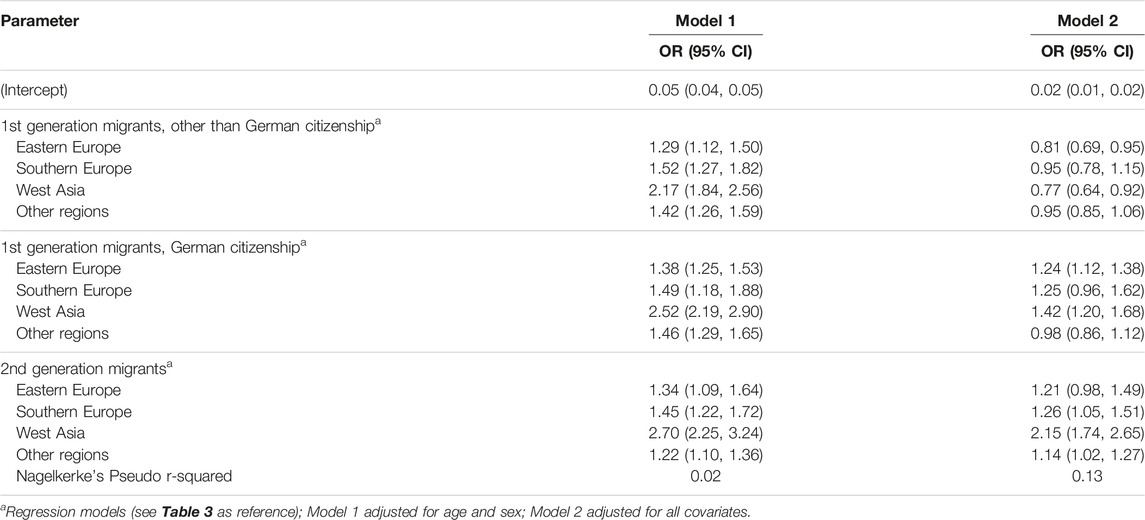
TABLE 4. Logistic regression models to examine associations of different migration characteristics with depressive symptoms (dichotomized PHQ-9, 1 = score ≥10 indicating depressive symptoms), specified by region of origin; N = 190,731 (German National Cohort (NAKO), Germany, 2014–2019).
Discussion
Summary and Interpretation
Based on data of the German NAKO, we found higher prevalence of depressive symptoms in all migrant groups under study compared to non-migrant populations. These findings are in line with previous larger population studies [14, 22, 45–47]. For example, Brydsten et al. showed that risks of mental distress was higher among various migrant groups compared to natives, based on the Swedish Health on Equal Terms survey [14]. Similarly, Aichberger et al. found higher risks for depression in various migrant groups from different regions in Europe compared to non-migrants based on the SHARE data [45].
Furthermore, it was investigated to what extent these differences can be attributed to socio-economic indicators and social relations. The confounding effects of SEP and social relations varied in different populations: The higher odds ratios of depressive symptoms in 1st generation migrants without German citizenship disappeared or even reversed when SEP and both aspects of social relations were controlled for. This was not the case in 1st generation migrants with German citizenship and in 2nd generation migrants. A differentiation by region of origin detected further variations in associations of migration background and depressive symptoms. Moreover, variations in the role of SEP and social relations for these associations were identified. Highest odds ratios of reporting depressive symptoms compared to non-migrants were found especially in migrants with origin in West Asia, independent of migrant generation or citizenship and not attributable to SEP or social relations. This is in line with previous findings that revealed higher degrees of somatization and stronger symptom severity in Turkish migrants in Germany [26].
The introduction of social relations and SEP into regression models showed varying effects on the associations of migration and depressive symptoms. A possible explanation for the persistence of higher risks for depressive symptoms in 1st generation migrants with German citizenship and in 2nd generation migrants after the introduction of SEP and social relations is that being born in Germany or having German citizenship may be associated with a stronger sense of belonging and a stronger desire for social acceptance [48]. Their continuous refusal, the feelings of not belonging and a higher likelihood of experiencing discrimination may translate into higher risks of poorer mental health [17, 49, 50]. This has been labelled as a form of social defeat, which is associated with higher health risks due to increased stress levels [51]. Earlier studies detected highest degrees of perceived discrimination in Turkish migrants, especially in second-generation migrants and in women [48, 49]. Perceived discrimination and the awareness of relative deprivation are reasons for the integration paradox which states that this process is even more likely to occur in the higher educated and structurally integrated immigrants [52]. It describes that economically more integrated and often higher educated 2nd generation migrants are more likely to turn away from the host society, psychologically. The perception of not belonging might lead to a stronger orientation towards acculturation styles that can be labelled as separation [48]. In contrast, denying or not acquiring German citizenship may be associated with lower hopes for long-term integration, possibly including greater involvement in migrant communities. Differences in risks for poorer mental health are more likely to be explained primarily by socioeconomic disadvantage and lower social integration in these migrant populations, while in 2nd generation migrants, in 1st generation migrant with German citizenship and to a certain degree in German resettlers the effects of SEP and social integration on the mental health gap are considerably lower due to the above-described integration paradox and its consequences.
A healthy migrant effect might play a role in explaining the lower risks of 1st generation migrants without German citizenship. Selection effects such as in-selection, which leads to more homogenous and often healthier groups of migrants leaving their countries of origin, and out-selection, which might favour those economically better off to stay in host-countries are likely. In-selection only affects those with own migration experience and out-selection is potentially more likely for those without German citizenship, which has been labelled as “unhealthy re-migration effect” [53]. These effects might contribute to the differences observed in the likelihood of depressive symptoms in different migrant populations [54, 55].
When looking at social integration in migrant subgroups, it might be valuable for future studies to differentiate between bonding (within one’s own community) and bridging (with other local communities) social capital. For example, the ability to share common cultural practices for refugees within their own community (binding capital) was positively associated with quality of life and mental health [56]. Nonetheless, active commitment within communities and especially across different communities and successful integration in host societies is severely hampered when relevant resources (language, economic, cultural etc.) are lacking and when other needs regularly outweigh the need to socially meet and connect. These effects potentially vary between different migrant subgroups.
Furthermore, future studies need to take a more nuanced look at the migration aspect. Our results vary depending on which specific population of migrants is considered. The more detailed analyses show differences with regard to mental health risks within different migrant populations, and they also indicate that different aspects are relevant for different migrant populations in terms of reducing the risks of depressive symptoms compared to non-migrant populations. Analyses that fail to consider subgroups within migrant populations would fail to detect these differences [57].
Strengths and Limitations
These analyses of the NAKO data are limited by various aspects. The four items of emotional support and SNI, included in a computer-assisted personal interview at the end of the NAKO assessment, have a comparably high proportion of missing data [31, 33]. Analyses of missing data showed that older and less educated persons as well as those a with migration background were more likely to not participate in the computer-assisted interview. Thus, data were not missing completely at random. Applying multiple imputation can reduce bias and improve efficiency of statistical results [58, 59]. Moreover, we additionally calculated all regression models with original (per-protocol) data that included missing values. We found no patterns that indicate any introduced bias due to imputation of missing data. The average assessment time per participant of up to 5.6 h leads to high quality data and a rich amount of information, but on the other hand also makes baseline assessment overall very time-consuming. Such a high average assessment time makes an early termination more likely for older-aged persons, persons with impaired health or with lower language skills, as mentioned above. Moreover, baseline data collection took more than 5 years (from March 2014 until September 2019). It cannot be ruled out that changes and events during this time period affected the outcome examined here.
Another limiting aspect is the survey language of the NAKO, which is German, without options for translation or the provision of other foreign languages [28]. This systematic underrepresentation of non-German speaking groups may lead to an underestimation of differences in depressive symptoms, as these groups are more likely to face greater challenges in social and socioeconomic integration (e.g., labour market) due to language barriers (see for example, [60, 61]). Data on language proficiency was collected at the end of the interviews, but was not available for the analyses conducted here.
Furthermore, as discussed above, experiences of discrimination and racism were not assessed in the NAKO study. Both aspects and their subjective perception are important determinants of (mental) health and of (successful) social integration, especially in migrant populations [62, 63]. Brydsten et al. [14] found that experiences of discrimination were an explanatory factor regarding the mental health gap especially for non-European migrants. Additional relevant migration-related indicators have recently been proposed for future research on migration and health in Germany, including reasons for migration, self-reported discrimination, social support and sense of belonging to society [24]. Therefore, the neglect of subjective experiences of discrimination and racism remains a blind spot in the NAKO study. These experiences should be addressed and integrated in future studies.
Conceptual overlap has to be considered when interpreting the association between social relations and depressive symptoms. Social isolation is an aspect that is also included in the PHQ-9 [34]. Thus, in a way, social relations are included in the independent and in the dependent variable. Finally, although the NAKO is designed as a prospective cohort-study, only data from the first wave was available for analysis. This cross-sectional design impedes causal interpretations. Reverse causation cannot be ruled out.
Major strength of the NAKO is the sample size. With over 200,000 participants and a wide range of indicators of health, SEP, social relations and many other it offers large potential for social epidemiology. Especially regarding migrant populations, the NAKO allows a differentiation within the same dataset which is still rare. Regions of origin, citizenship, country of birth and information on parents’ country of birth offer the possibility to specify research questions regarding certain migrant populations and overcome the more general comparison of one migrant and one non-migrant population.
Conclusion
Based on data from the NAKO study in Germany, higher risks of depressive symptoms were found in migrants and their descendants than in non-migrant groups. After the inclusion of SEP, SNI and emotional support in the regression models, the effect of migration status on depressive symptoms remained visible but was reduced, indicating the important role of SEP and social relations for this mental health gap. Both the risks of depressive symptoms and the effects of SEP and social relations varied considerably, when different migrant subgroups and regions of origin were taken into account. This is an important message for future studies in social epidemiology concerning comparisons of migrant and non-migrant populations, as this heterogeneity within migrants and their descendants should be taken into account, whenever possible. The NAKO data offer the potential of differentiated analysis. In the near future, data of the NAKO follow-up will be available and the cross-sectional analysis of the present study should be replicated in a longitudinal-design.
Author Contributions
NV, DL, and OK developed the research questions. NV and DL prepared the data for analyses, DL conducted the data analyses. CW advised on data analysis and data structure. All authors except NV, DL and OK were involved in data collection of the NAKO. NV, DL and OK drafted the manuscript. NV, DL and OK contributed to the interpretation of the data. All authors contributed to the article and approved the submitted version.
Funding
This project was conducted with data from the German National Cohort (NAKO, www.nako.de). The NAKO is funded by the Federal Ministry of Education and Research (BMBF) (project funding reference numbers: 01ER1301A/B/C and 01ER1511D), federal states and the Helmholtz Association with additional financial support by the participating universities and the institutes of the Leibniz Association.
Conflict of Interest
The authors declare that they do not have any conflicts of interest.
Acknowledgments
We thank all participants who took part in the NAKO study and the staff in this research program.
References
1. Glaesmer, H, Riedel-Heller, S, Braehler, E, Spangenberg, L, and Luppa, M Age- and Gender-specific Prevalence and Risk Factors for Depressive Symptoms in the Elderly: a Population-Based Study. Int Psychogeriatr (2011) 23(8):1294–300. doi:10.1017/S1041610211000780
2. Hölzel, L, Härter, M, Reese, C, and Kriston, L. Risk Factors for Chronic Depression — A Systematic Review. J Affect Disord (2011) 129(1):1–13. doi:10.1016/j.jad.2010.03.025
3. Gkiouleka, A, and Huijts, T. Intersectional Migration-Related Health Inequalities in Europe: Exploring the Role of Migrant Generation, Occupational Status & Gender. Soc Sci Med (2020) 267:113218. doi:10.1016/j.socscimed.2020.113218
4. Lorant, V, Deliège, D, Eaton, W, Robert, A, Philippot, P, and Ansseau, M. Socioeconomic Inequalities in Depression: a Meta-Analysis. Am J Epidemiol (2003) 157(2):98–112. doi:10.1093/aje/kwf182
5. Jacquet, E, Robert, S, Chauvin, P, Menvielle, G, Melchior, M, and Ibanez, G. Social Inequalities in Health and Mental Health in France. The Results of a 2010 Population-Based Survey in Paris Metropolitan Area. PLoS One (2018) 13(9):e0203676. doi:10.1371/journal.pone.0203676
6. Amiri, S. Unemployment Associated with Major Depression Disorder and Depressive Symptoms: a Systematic Review and Meta-Analysis. Int J Occup Saf Ergon (2022) 28(4):2080–92. doi:10.1080/10803548.2021.1954793
7. Ladin, K, Daniels, N, and Kawachi, I. Exploring the Relationship between Absolute and Relative Position and Late-Life Depression: Evidence from 10 European Countries. Gerontologist (2010) 50(1):48–59. doi:10.1093/geront/gnp065
8. Ribeiro, WS, Bauer, A, Andrade, MCR, York-Smith, M, Pan, PM, Pingani, L, et al. Income Inequality and Mental Illness-Related Morbidity and Resilience: a Systematic Review and Meta-Analysis. Lancet Psychiatry (2017) 4(7):554–62. doi:10.1016/S2215-0366(17)30159-1
9. Patel, V, Burns, JK, Dhingra, M, Tarver, L, Kohrt, BA, and Lund, C. Income Inequality and Depression: a Systematic Review and Meta-Analysis of the Association and a Scoping Review of Mechanisms. World Psychiatry (2018) 17(1):76–89. doi:10.1002/wps.20492
10. Elwadhi, D, and Cohen, A. Social Inequalities in Antidepressant Treatment Outcomes: a Systematic Review. Soc Psychiatry Psychiatr Epidemiol (2020) 55(10):1241–59. doi:10.1007/s00127-020-01918-5
11. Santini, ZI, Koyanagi, A, Tyrovolas, S, Mason, C, and Haro, JM. The Association between Social Relationships and Depression: a Systematic Review. J Affect Disord (2015) 175:53–65. doi:10.1016/j.jad.2014.12.049
12. Gariépy, G, Honkaniemi, H, and Quesnel-Vallée, A. Social Support and protection from Depression: Systematic Review of Current Findings in Western Countries. Br J Psychiatry (2016) 209(4):284–93. doi:10.1192/bjp.bp.115.169094
13. Aichberger, MC, Neuner, B, Hapke, U, Rapp, MA, Schouler-Ocak, M, and Busch, MA. Association between Migrant Status and Depressive Symptoms in the Older Population in Germany. Psychiatr Prax (2012) 39(03):116–21. doi:10.1055/s-0031-1276936
14. Brydsten, A, Rostila, M, and Dunlavy, A. Social Integration and Mental Health - a Decomposition Approach to Mental Health Inequalities between the Foreign-Born and Native-Born in Sweden. Int J Equity Health (2019) 18(1):48. doi:10.1186/s12939-019-0950-1
15. Close, C, Kouvonen, A, Bosqui, T, Patel, K, O’Reilly, D, and Donnelly, M. The Mental Health and Wellbeing of First Generation Migrants: a Systematic-Narrative Review of Reviews. Glob Health (2016) 12(1):47. doi:10.1186/s12992-016-0187-3
16. Williams, DR, Lawrence, JA, and Davis, BA. Racism and Health: Evidence and Needed Research. Annu Rev Public Health (2019) 40:105–25. doi:10.1146/annurev-publhealth-040218-043750
17. Bilecen, B, and Vacca, R. The Isolation Paradox: A Comparative Study of Social Support and Health across Migrant Generations in the U.S. U.S Soc Sci Med (2021) 283:114204. doi:10.1016/j.socscimed.2021.114204
18. Butler, M, Warfa, N, Khatib, Y, and Bhui, K. Migration and Common Mental Disorder: an Improvement in Mental Health over Time? Int Rev Psychiatry (2015) 27(1):51–63. doi:10.3109/09540261.2014.996858
19. Lin, Y, Zhang, Q, Chen, W, and Ling, L. The Social Income Inequality, Social Integration and Health Status of Internal Migrants in China. Int J Equity Health (2017) 16:139. doi:10.1186/s12939-017-0640-9
20. Umberson, D, and Karas Montez, J. Social Relationships and Health: A Flashpoint for Health Policy. J Health Soc Behav (2010) 51(1):S54–66. doi:10.1177/0022146510383501
21. Hallgren, M, Lundin, A, Tee, FY, Burström, B, and Forsell, Y. Somebody to Lean on: Social Relationships Predict post-treatment Depression Severity in Adults. Psychiatry Res (2017) 249:261–7. doi:10.1016/j.psychres.2016.12.060
22. Lecerof, SS, Stafström, M, Westerling, R, and Östergren, PO. Does Social Capital Protect Mental Health Among Migrants in Sweden? Health Promot Int (2016) 31(3):644–52. doi:10.1093/heapro/dav048
23. Alegría, M, Álvarez, K, and DiMarzio, K. Immigration and Mental Health. Curr Epidemiol Rep (2017) 4(2):145–55. doi:10.1007/s40471-017-0111-2
24. Kajikhina, K, Koschollek, C, Sarma, N, Bug, M, Wengler, A, Bozorgmehr, K, et al. Recommendations for Collecting and Analysing Migration-Related Determinants in Public Health Research. J Health Monit (2023) 1:52–72. doi:10.25646/11144
25. Will, AK. The German Statistical Category “Migration Background”: Historical Roots, Revisions and Shortcomings. Ethnicities (2019) 19(3):535–57. doi:10.1177/1468796819833437
26. Morawa, E, Dragano, N, Jöckel, KH, Moebus, S, Brand, T, and Erim, Y. Somatization Among Persons with Turkish Origin: Results of the Pretest of the German National Cohort Study. J Psychosom Res (2017) 96:1–9. doi:10.1016/j.jpsychores.2017.02.014
27. Bermejo, I, Mayninger, E, Kriston, L, and Härter, M. Mental Disorders in People with Migration Background Compared with German General Population. Psychiatr Prax (2010) 37(05):225–32. doi:10.1055/s-0029-1223513
28. Wiessner, C, Keil, T, Krist, L, Zeeb, H, Dragano, N, Schmidt, B, et al. Persons with Migration Background in the German National Cohort (NAKO)-sociodemographic Characteristics and Comparisons with the German Autochthonous Population. Bundesgesundheitsbl (2020) 63(3):279–89. doi:10.1007/s00103-020-03097-9
29.German Federal Statistics Office. Migration and Integration [Internet] (2023). Available from: https://www.destatis.de/EN/Themes/Society-Environment/Population/Migration-Integration/_node.html (Accessed July 10, 2023).
30.German National Cohort (GNC) Consortium. The German National Cohort: Aims, Study Design and Organization. Eur J Epidemiol (2014) 29(5):371–82. doi:10.1007/s10654-014-9890-7
31. Schipf, S, Schöne, G, Schmidt, B, Günther, K, Stübs, G, Greiser, KH, et al. The Baseline Assessment of the German National Cohort (NAKO Gesundheitsstudie): Participation in the Examination Modules, Quality Assurance, and the Use of Secondary Data. Bundesgesundheitsbl (2020) 63(3):254–66. doi:10.1007/s00103-020-03093-z
32. Kuss, O, Becher, H, Wienke, A, Ittermann, T, Ostrzinski, S, Schipf, S, et al. Statistical Analysis in the German National Cohort (NAKO) - Specific Aspects and General Recommendations. Eur J Epidemiol (2022) 37(4):429–36. doi:10.1007/s10654-022-00880-7
33. Peters, A, Peters, A, Greiser, KH, Göttlicher, S, Ahrens, W, Albrecht, M, et al. Framework and Baseline Examination of the German National Cohort (NAKO). Eur J Epidemiol (2022) 37(10):1107–24. doi:10.1007/s10654-022-00890-5
34. Kroenke, K, Spitzer, RL, and Williams, JBW. The PHQ-9: Validity of a Brief Depression Severity Measure. J Gen Intern Med (2001) 16(9):606–13. doi:10.1046/j.1525-1497.2001.016009606.x
35. Streit, F, Zillich, L, Frank, J, Kleineidam, L, Wagner, M, Baune, BT, et al. Lifetime and Current Depression in the German National Cohort (NAKO). World J Biol Psychiatry (2022) 2022:1–16. doi:10.1080/15622975.2021.2014152
36. Dragano, N, Reuter, M, Berger, K, Engels, M, Schmidt, B, Greiser, KH, et al. Increase in Mental Disorders during the COVID-19 Pandemic-The Role of Occupational and Financial Strains. Dtsch Arztebl Int (2022) 119(11):179–87. doi:10.3238/arztebl.m2022.0133
37. Manea, L, Gilbody, S, and McMillan, D. A Diagnostic Meta-Analysis of the Patient Health Questionnaire-9 (PHQ-9) Algorithm Scoring Method as a Screen for Depression. Gen Hosp Psychiatry (2015) 37(1):67–75. doi:10.1016/j.genhosppsych.2014.09.009
38. Stordal, E, Mykletun, A, and Dahl, AA. The Association between Age and Depression in the General Population: a Multivariate Examination. Acta Psychiatr Scand (2003) 107(2):132–41. doi:10.1034/j.1600-0447.2003.02056.x
40. Brissette, I, Cohen, S, and Seeman, TE. Measuring Social Integration and Social Networks. In: S Cohen, LG Underwood, and BH Gottlieb, editors. Social Support Measurement and Intervention: A Guide for Health and Social Scientists [Internet]. New York: Oxford University Press (2000). Available from. doi:10.1093/med:psych/9780195126709.003.0003
41. Schenk, L, Bau, AM, Borde, T, Butler, J, Lampert, T, Neuhauser, H, et al. A Basic Set of Indicators for Mapping Migrant Status. Recommendations for Epidemiological Practice. Bundesgesundheitsbl (2006) 49(9):853–60. doi:10.1007/s00103-006-0018-4
42.United Nations Statistics Divison (UNSD). Methodology - Standard Country or Area Codes for Statistical Use (M49) [Internet] (2023). cited 2023 Feb 13. Available from: https://unstats.un.org/unsd/methodology/m49/ (Accessed July 10, 2023).
43. Buuren, SV, and Groothuis-Oudshoorn, K. Mice: Multivariate Imputation by Chained Equations in R. J Stat Softw (2011) 45:1–67. doi:10.18637/jss.v045.i03
44.R Core Team. R: A Language and Environment for Statistical Computing. Vienna, Austria: R Foundation for Statistical Computing (2022).
45. Aichberger, M, Schouler-Ocak, M, Mundt, A, Busch, M, Nickels, E, Heimann, H, et al. Depression in Middle-Aged and Older First Generation Migrants in Europe: Results from the Survey of Health, Ageing and Retirement in Europe (SHARE). Eur Psychiatry (2010) 25:468–75. doi:10.1016/j.eurpsy.2009.11.009
46. Morawa, E, Brand, T, Dragano, N, Jöckel, KH, Moebus, S, and Erim, Y. Associations between Acculturation, Depressive Symptoms, and Life Satisfaction Among Migrants of Turkish Origin in Germany: Gender- and Generation-Related Aspects. Front Psychiatry (2020) 11:715. doi:10.3389/fpsyt.2020.00715
47. Nesterko, Y, Friedrich, M, Brähler, E, Hinz, A, and Glaesmer, H. Mental Health Among Immigrants in Germany – the Impact of Self-Attribution and Attribution by Others as an Immigrant. BMC Public Health (2019) 19(1):1697. doi:10.1186/s12889-019-8060-y
48. Morawa, E, and Erim, Y. Acculturation and Depressive Symptoms Among Turkish Immigrants in Germany. Int J Environ Res Public Health (2014) 11(9):9503–21. doi:10.3390/ijerph110909503
49. Aichberger, MC, Bromand, Z, Rapp, MA, Yesil, R, Montesinos, AH, Temur-Erman, S, et al. Perceived Ethnic Discrimination, Acculturation, and Psychological Distress in Women of Turkish Origin in Germany. Soc Psychiatry Psychiatr Epidemiol (2015) 50:1691–700. doi:10.1007/s00127-015-1105-3
50. Gkiouleka, A, Avrami, L, Kostaki, A, Huijts, T, Eikemo, TA, and Stathopoulou, T. Depressive Symptoms Among Migrants and Non-migrants in Europe: Documenting and Explaining Inequalities in Times of Socio-Economic Instability. Eur J Public Health (2018) 28(5):54–60. doi:10.1093/eurpub/cky202
51. Björkqvist, K. Social Defeat as a Stressor in Humans. Physiol Behav (2001) 73(3):435–42. doi:10.1016/s0031-9384(01)00490-5
52. Verkuyten, M. The Integration Paradox: Empiric Evidence from the Netherlands. Am Behav Sci (2016) 60(5–6):583–96. doi:10.1177/0002764216632838
53. Razum, O, Zeeb, H, Akgün, HS, and Yilmaz, S. Low Overall Mortality of Turkish Residents in Germany Persists and Extends into a Second Generation: Merely a Healthy Migrant Effect? Trop Med Int Health (1998) 3(4):297–303. doi:10.1046/j.1365-3156.1998.00233.x
54. Zufferey, J. Investigating the Migrant Mortality Advantage at the Intersections of Social Stratification in Switzerland: The Role of Vulnerability. Demographic Res (2016) 34(32):899–926. doi:10.4054/demres.2016.34.32
55. Reus-Pons, M, Kibele, EUB, and Janssen, F. Differences in Healthy Life Expectancy between Older Migrants and Non-migrants in Three European Countries over Time. Int J Public Health (2017) 62(5):531–40. doi:10.1007/s00038-017-0949-6
56. Ager, A, and Strang, A. Understanding Integration: A Conceptual Framework. J Ref Stud (2008) 21(2):166–91. doi:10.1093/jrs/fen016
57. James, R, Blanchet, K, Orcutt, M, and Kumar, B. Migration Health Research in the European Region: Sustainable Synergies to Bridge the Research, Policy and Practice gap. Lancet Reg Health Eur (2021) 5:100124. doi:10.1016/j.lanepe.2021.100124
58. Lee, KJ, Tilling, KM, Cornish, RP, Little, RJA, Bell, ML, Goetghebeur, E, et al. Framework for the Treatment and Reporting of Missing Data in Observational Studies: The Treatment and Reporting of Missing Data in Observational Studies Framework. J Clin Epidemiol (2021) 134:79–88. doi:10.1016/j.jclinepi.2021.01.008
59. Tsiampalis, T, and Panagiotakos, DB. Missing-data Analysis: Socio-Demographic, Clinical and Lifestyle Determinants of Low Response Rate on Self-Reported Psychological and Nutrition Related Multi-Item Instruments in the Context of the ATTICA Epidemiological Study. BMC Med Res Methodol (2020) 20(1):148. doi:10.1186/s12874-020-01038-3
60. Woodall, A, Morgan, C, Sloan, C, and Howard, L. Barriers to Participation in Mental Health Research: Are There Specific Gender, Ethnicity and Age Related Barriers? BMC Psychiatry (2010) 10:103. doi:10.1186/1471-244X-10-103
61. Bonevski, B, Randell, M, Paul, C, Chapman, K, Twyman, L, Bryant, J, et al. Reaching the Hard-To-Reach: a Systematic Review of Strategies for Improving Health and Medical Research with Socially Disadvantaged Groups. BMC Med Res Methodol (2014) 14(1):42. doi:10.1186/1471-2288-14-42
62. Hynie, M. The Social Determinants of Refugee Mental Health in the Post-Migration Context: A Critical Review. Can J Psychiatry (2018) 63(5):297–303. doi:10.1177/0706743717746666
Keywords: German National Cohort, NAKO, migrant health, migration, social relations, depressive symptoms, socioeconomic position, social integration
Citation: Vonneilich N, Becher H, Bohn B, Brandes B, Castell S, Deckert A, Dragano N, Franzke C-W, Führer A, Gastell S, Greiser H, Keil T, Klett-Tammen C, Koch-Gallenkamp L, Krist L, Leitzmann M, Meinke-Franze C, Mikolajczyk R, Moreno Velasquez I, Obi N, Peters A, Pischon T, Reuter M, Schikowski T, Schmidt B, Schulze M, Sergeev D, Stang A, Völzke H, Wiessner C, Zeeb H, Lüdecke D and von dem Knesebeck O (2023) Associations of Migration, Socioeconomic Position and Social Relations With Depressive Symptoms – Analyses of the German National Cohort Baseline Data. Int J Public Health 68:1606097. doi: 10.3389/ijph.2023.1606097
Received: 18 April 2023; Accepted: 05 July 2023;
Published: 18 July 2023.
Edited by:
Jaroslava Kopcakova, University of Pavol Jozef Šafárik, SlovakiaReviewed by:
Michel Oris, University of Geneva, SwitzerlandCopyright © 2023 Vonneilich, Becher, Bohn, Brandes, Castell, Deckert, Dragano, Franzke, Führer, Gastell, Greiser, Keil, Klett-Tammen, Koch-Gallenkamp, Krist, Leitzmann, Meinke-Franze, Mikolajczyk, Moreno Velasquez, Obi, Peters, Pischon, Reuter, Schikowski, Schmidt, Schulze, Sergeev, Stang, Völzke, Wiessner, Zeeb, Lüdecke and von dem Knesebeck. This is an open-access article distributed under the terms of the Creative Commons Attribution License (CC BY). The use, distribution or reproduction in other forums is permitted, provided the original author(s) and the copyright owner(s) are credited and that the original publication in this journal is cited, in accordance with accepted academic practice. No use, distribution or reproduction is permitted which does not comply with these terms.
*Correspondence: Nico Vonneilich, n.vonneilich@uke.de