- State Key Laboratory of Molecular Vaccinology and Molecular Diagnostics, School of Public Health, Xiamen University, Xiamen, China
Objectives: This study aims to evaluate the effects of Urban and Rural Resident Basic Medical Insurance (URRBMI) integration on healthcare utilization and explore the contribution of URRBMI to healthcare utilization inequality among middle-aged and older adults.
Methods: Using data from the China Health and Retirement Longitudinal Study (CHARLS) 2011–2018. The difference-in-difference model, concentration index (CI), and decomposition method were adopted.
Results: The results suggested that the probability of outpatient visits and the number of outpatient visits had decreased by 18.2% and 10.0% respectively, and the number of inpatient visits had increased by 3.6%. However, URRBMI had an insignificant effect on the probability of inpatient visits. A pro-poor inequality for the treatment group was observed. The decomposition revealed that the URRBMI contributed to the pro-poor inequality in healthcare utilization.
Conclusion: The findings suggest that URRBMI integration has decreased outpatient care utilization and improved the number of inpatient visits. While the URRBMI has improved healthcare utilization inequality, some challenges still exist. Comprehensive measures should be taken in the future.
Introduction
In 2005, the WHO member states committed to achieving the goal of universal health coverage (UHC), to guarantee all people have equitable access to healthcare without the risk of financial ruin (1). An increasing number of developing countries have taken different measures to achieve UHC (2). Since the late 1990’s, China has launched a series of healthcare reforms and has established three main schemes: The Urban Employee Basic Medical Insurance (UEBMI), the New Cooperative Medical Scheme (NCMS), and the Urban Resident Basic Medical Insurance (URBMI). UEBMI was established in 1998 to provide medical insurance for urban workers and retirees in the formal sector. NCMS was established in 2003, in which rural residents voluntarily choose to participate. China began piloting the URBMI system in 2007 to address the issue of medical coverage for informally employed residents, jobless residents, older adults, and children in urban areas without UEBMI. In 2011, the percentages of urban and rural residents who had health insurance protection increased to 89% and 97.5%, respectively (3, 4). A brief introduction to the health insurance schemes in China was shown in Supplementary Table S1.
Despite significant progress in the healthcare system, the gap between NCMS and URBMI was growing. First, the urban-rural segmentation of health insurance had become an important factor in healthcare utilization inequality in rural areas (5). Second, the URBMI was implemented at the city level and the NCMS was implemented at the county level. The lower administration level of NCMS undermined the risk-sharing of health insurance (6). Gradually, reducing inequalities has become a growing concern of the public, and has been widely recognized as a major objective of health policies in China (7). To provide better healthcare services and financial protection for rural residents, some provinces and municipalities (i.e., Chongqing, Tianjin, Guangdong, Shandong, and Zhejiang) made a series of attempts to merge NCMS and URBMI from 2008 to 2015. In 2016, the Chinese government officially decided to merge NCMS and URBMI nationwide and established Urban and Rural Resident Basic Medical Insurance (URRBMI) with some specific guidelines. URRBMI is intended to cover urban residents, who are not eligible under UEBMI, and all rural residents. The total premiums of URRBMI are higher than NCMS and URBMI, and the population covered is substantially larger. This suggests that the URRBMI scheme could better perform the coverage function of the healthcare system for participants, and improve risk-sharing capacity. By enrolling in URRBMI, rural residents could receive the same level of reimbursement for medicines and healthcare services as urban residents and could enjoy a wider choice of facilities (8).
One of the target groups of URRBMI is older adults, a population with higher medical needs than the overall population, which poses challenges to URRBMI (9, 10). China has the largest population in the world and is aging rapidly. By the end of 2020, individuals aged 60 and above reached 264 million in China, accounting for 18.7% of the total population (11). The tendency of aging would result in increasing demands on health services and increasing the financial risk of health insurance funds. However, it remains unclear how URRBMI integration affects healthcare utilization among middle-aged and older adults in China.
The link between health insurance and healthcare utilization has been studied in a large body of literature. For some countries and regions that have implemented universal health insurance, such as Japan, South Korea, Thailand, Taiwan, and most European countries, the expansion of health insurance coverage has significantly improved the healthcare utilization of the population (12–15). A growing body of research showed that the effects of NCMS and URBMI had been generally positive, improving healthcare utilization, promoting equity in health financing, and decreasing out-of-pocket payment (16–18). Existing evidence on the effects of URRBMI integration is mixed. Liu et al. (19) found that the healthcare options and quality for rural residents were improved and medical expenses were significantly reduced after the implementation of URRBMI. Ma (20) reported that the implementation of URRBMI significantly increased healthcare utilization for rural residents, while Zhou et al. (8) found no impact on the likelihood of hospital admission among rural residents, due to differences in the data sources and methods used. Several studies have investigated inequality in URRBMI. Li et al. (21) revealed that the integration of URRBMI could improve equity in healthcare utilization. Ren et al. (22) provided evidence of benefit equity for outpatient care with the integration of URRBMI.
Overall, research on URRBMI has gradually increased in recent years, regarding medical expenses, healthcare utilization, catastrophic health expenditure, health outcomes, and financial protection (5, 8, 19, 21, 23, 24). However, most of the above literature used data before 2016 (the pilot phase of URRBMI), and there is little evidence of the effect of URRBMI after 2016 (the formal integration phase of URRBMI). After the integration of URRBMI nationwide, it is critical to investigate the effect of this policy on healthcare utilization and its inequality. Therefore, we used more recent data (up to 2018) to assess the effect of URRBMI integration after 2016, which could update the estimates in the previous literature. In this study, we aim to evaluate the effects of URRBMI integration on healthcare utilization, calculate the concentration index (CI) and decompose the CI to learn the contribution of URRBMI to the total inequality. The findings will provide references for the development of the URRBMI scheme, and lessons for other countries to achieve UHC.
Methods
Data Sources
The data for this study were derived from the China Health and Retirement Longitudinal Study (CHARLS), which is conducted by the National Development Research Institute of Peking University. The CHARLS was a nationally representative longitudinal survey targeting Chinese community-dwelling individuals aged 45 years and older along with their spouses. The CHARLS national baseline survey used multistage stratified probability-proportionate-to-size sampling to cover 28 provinces, 150 countries/districts, and 450 villages/urban communities, involving 17,708 individuals in 10,257 households (25). Using a four-stage and well-established sample design, CHARLS provides comprehensive information about demographics, health, insurance status, work and retirement, income and consumption, assets, as well as community-level information. In this paper, the panel data comprising from CHARLS 2011, 2013, 2015, and 2018 are selected as the research samples.
The CHARLS data allows us to identify whether sample individuals live in a province/municipality implementing the URRBMI. In this study, the treatment and control groups were determined according to whether the URRBMI was implemented in each province. We defined treatment provinces as those provinces which adopted the integration from January 2016 to December 2017 (Supplementary Table S2 presents the timeline of URRBMI integration in China). Five provinces and municipalities had an early integration of the URRBMI before 2016, which may lead to an underestimation of the URRBMI effects (26). Thus, we excluded these provinces and municipalities from the treatment group. The control provinces are those provinces that were integrated after 2017. The pre-integration period is assigned to the years 2011–2017 whereas the post-integration period is 2018.
We used 2011 survey data as the baseline, with 17,708 participants included in the study. 5,774 participants dropped out in the three waves from 2013 to 2018. We excluded participants if they lived in the five provinces and municipalities which implemented the URRBMI before 2016. Participants who were insured by other medical insurance (e.g., UEBMI or supplemental insurance) were excluded. In addition, participants under 45 years old and with abnormal or missing variables (e.g., gender, age, marital status, and self-reported health) were also excluded. The final sample of 6,204 participants with 5,438 in the treatment group and 766 in the control group (Supplementary Figure S1).
Variables
The implementation of URRBMI is involved as an essential independent variable. The implementation of URRBMI = 1 means that the group is a treatment group, and the year is the post-integration period; otherwise, the implementation of URRBMI = 0.
The main dependent variables pertain to individuals’ healthcare utilization. Following the extant literature on the determinants of healthcare utilization in China using the CHARLS survey (27, 28), we constructed four dependent variables: 1) whether the individual had any outpatient visit last month; 2) whether the individual received any inpatient care last year; 3) the number of outpatient visits during last month; and 4) the number of inpatient visits during last year.
In light of existing literature (29–31), we used an adjusted Anderson Health Services Utilization Model as our starting point to select controlled variables, which might influence healthcare utilization. Three types of variables were controlled in the empirical analysis: the predisposing characteristics, including age, gender, marital status, and education status; the enabling resources, including residence and annual personal income; and the need factors, including chronic disease and self-reported health status. Supplementary Table S3 offers a list of summary statistics and a brief description of our variables.
Statistical Analysis
Difference-in-difference
The difference-in-difference (DID) approach is key to evaluating interventions to inform health policymakers and future policies. We employed the DID model to identify the impact of URRBMI on healthcare utilization.
In Eq 1,
Concentration Index and Decomposition
The concentration index quantifies the degree of socioeconomic-related inequality in healthcare utilization (32). The index is bounded between −1 and 1, 0 denotes perfect equality, whereas a positive (negative) value indicates that a healthcare variable is more concentrated among the rich (the poor) middle-aged and older adults (7). The formula for computing the CI is:
h represents the healthcare utilization indicators, r represents the fractional rank of personal income,
The CI can be decomposed into the contributions of individual factors to income-related inequality, in which each contribution is the product of the sensitivity concerning that factor and the degree of income-related inequality in that factor (33). The decomposition of the concentration index is applied to the OLS regression. The regression model is as follows:
Where y is the variable of healthcare utilization,
Where
All statistical analyses were performed using Excel V.2019 and Stata V.14.
Results
Descriptive Analysis
Table 1 shows the variables and the descriptive statistics of the sample. Overall, most participants were female, married, rural residents, and educated to below primary school. In 2011, most of the participants were 45–59 years old. Most participants had fair self-reported health status but one or more chronic health issues. The average annual personal income in the treatment and control groups were 1,773.37 yuan and 2,673.53 yuan respectively. In 2018, most of the participants were aged 60 years or above and had fair self-reported health status. The descriptive statistics of the dependent variables were shown in Supplementary Tables S4, S5.
Difference-in-difference Estimates
The effects of URRBMI on healthcare utilization are presented in Table 2. For outpatient care utilization, the probability of outpatient visits decreased by 18.2% (OR = 0.818, p < 0.05), and the number of inpatient visits decreased by 10.0% (coefficient = −0.100, p < 0.01). These results indicated that the URRBMI integration had negative and significant effects on the outpatient care utilization of middle-aged and older adults. In terms of inpatient care utilization, we found that the probability of inpatient visits was slightly increased, but the effect was statistically insignificant (OR = 1.003, p > 0.1). In contrast, the number of inpatient visits increased by 3.6% (coefficient = 0.036, p < 0.05), which meant that the integration of URRBMI had positive effects on the number of inpatient visits.
Heterogeneity Analysis
To further explore the heterogeneous effects of URRBMI integration on different subgroups, we performed two additional analyses. As shown in Supplementary Table S6, for urban residents, the integration of URRBMI had a negative and significant effect on the probability of outpatient visits (OR = 0.752, p < 0.05). For rural residents, the URRBMI integration was associated with a 12.2% (coefficient = −0.122, p < 0.01) decline in the number of outpatient visits. In addition, the introduction of URRBMI had insignificant effects on the inpatient care utilization for rural residents. We also test this implication by running a subgroup analysis across the age group. The results showed that the implementation of URRBMI not only had significant negative impacts on outpatient care utilization (p < 0.01) but also had positive effects on the number of inpatient visits (p < 0.05) for the older group. There was no significant effect of URRBMI on healthcare utilization for the middle-aged group.
Measurement of Inequality in Healthcare Utilization
Table 3 shows the change in the concentration index in healthcare utilization. In the treatment group, the CI of outpatient visits probability and number respectively decreased from −0.0124 to −0.0335 and from −0.0235 to −0.0539, indicating that the pro-poor inequality and lower-income people utilized more outpatient services. The CI of the inpatient visits probability increased from −0.0449 to −0.0203. The CI of inpatient visits number rose slightly but remained negative. This indicates that lower-income people also utilized more inpatient services. Regarding the control group, the CI of outpatient and inpatient visits probability remained positive from 2011 to 2018, implying that there is a pro-rich inequality. The CI values of the number of outpatient and inpatient visits in 2018 were negative, implying that there is a pro-poor inequality.
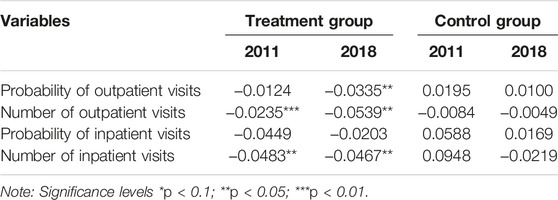
TABLE 3. The change of concentration index in healthcare utilization among treatment and control groups (China, 2011–2018).
Table 4 presents the decomposition results of CI for healthcare utilization in 2018. The CI of URRBMI implementation was negative (CI = −0.0215), meaning that lower-income people were more likely to be covered by it. The contribution rates of URRBMI implementation to the concentration index in the number of outpatient and inpatient visits were 9.96% and 6.59% respectively. The results indicated that URRBMI implementation contributed to the pro-poor inequality in healthcare utilization. In addition, the CI of having chronic diseases, and poor health status were negative, which reveals that lower-income people were more likely to be unhealthy. The factors that contributed most to the inequality were poor health status, annual personal income, and the age of 60 years and above.
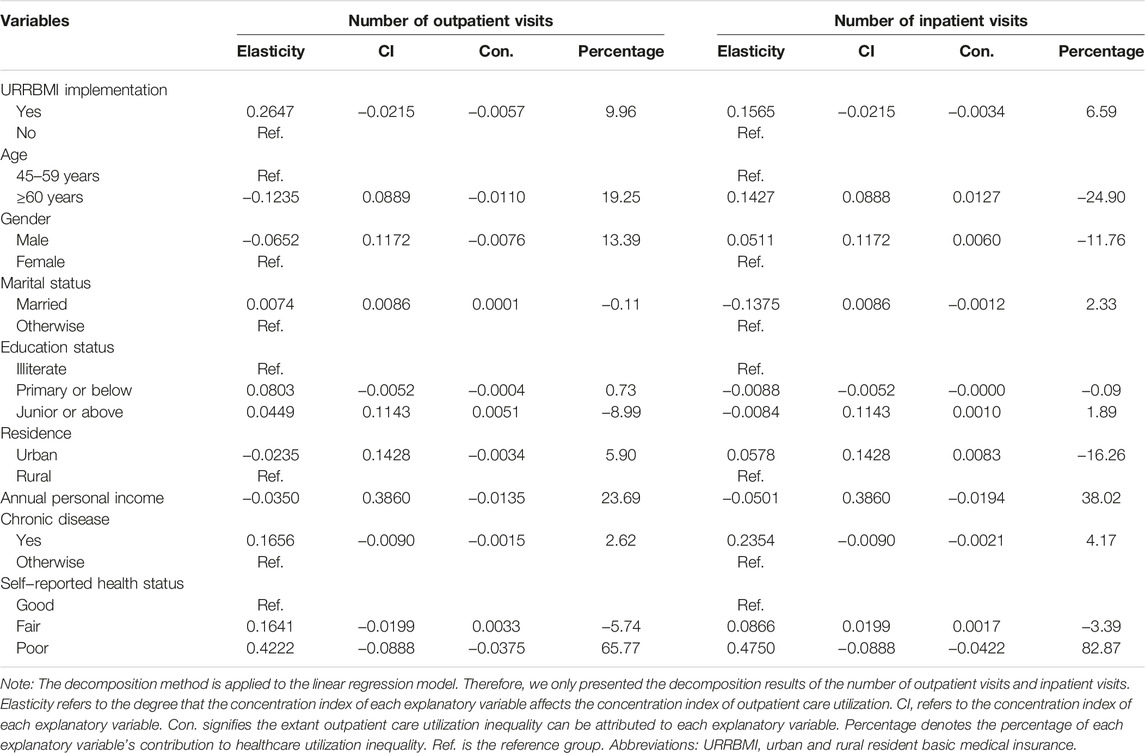
TABLE 4. Decomposition of Concentration Index for healthcare utilization in 2018 (China, 2011–2018).
Common Trend and Robustness Analysis
The most important premise of the DID model is the common trend assumption. The assumption states that changes in healthcare utilization would be the same in both treatment and control provinces in the pre-integration periods (34). Supplementary Figure S2 indicated that there were no differences in the pre-integration trends for healthcare utilization, and the assumption of parallel trends was generally satisfied.
To demonstrate the robustness of the results, we combined the propensity score matching and difference-in-difference (PSM-DID) methods. The combination of two methods to counter biases and the confounding of different sources, and the comparison of results, are encouraged in the literature (23). The results of the estimation of the PSM-DID method are shown in Supplementary Table S7, which are consistent with the DID results reported in Table 2, indicating that the results of the DID model were robust.
In addition, we conducted a placebo test by constructing a false treatment group. Supplementary Figure S3 reports the kernel density of estimated coefficients for the 500 randomly generated treatment groups and the distribution of the associated p-values. These results indicated that the empirical results are not severely biased due to randomness and any omitted variables.
Discussion
In this study, we estimated the effect of the URRBMI integration on healthcare utilization among middle-aged and elderly people in China from 2011 to 2018 with DID model. We also explored the changes in the healthcare utilization inequality before and after URRBMI integration, and further estimated the contribution of the URRBMI scheme to the inequality degree. Therefore, there are three aspects of our study that should be discussed.
Firstly, the empirical findings show that the URRBMI integration reduced the probability of outpatient visits and the number of outpatient visits, which is different from the evidence of earlier studies (36, 37). Meanwhile, the URRBMI integration significantly increased the number of inpatient visits, which is consistent with the previous study. This might have something to do with the fact that inpatient care has higher reimbursement rates and more medical services than outpatient care (38). Existing research has shown that health insurance has little association with the out-of-pocket cost of outpatient services, and patients can only receive greater reimbursement through inpatient care (36, 39, 40). This suggests that patients would reduce their use of outpatient care in favor of more inpatient care (41). Huang (6) proved that increased reimbursement rates after URRBMI integration could be a primary mechanism driving higher utilization of inpatient care. On the other hand, increased reimbursement for inpatient care might persuade some patients who would have previously sought outpatient care to choose the more expensive option, possibly encouraged by hospitals seeking to increase revenues. However, such behavior would lead to excessive medical treatment and increase the cost of healthcare utilization. Therefore, improving the benefits structure for outpatient care is imperative and guiding residents to use medical service resources rationally.
Second, the heterogeneous effects of URRBMI integration on each subgroup were observed. Compared with urban residents, the impact of URRBMI integration on rural residents was only reflected in outpatient care utilization and had an insignificant impact on inpatient care utilization, which differs from the expected results. URRBMI has a higher reimbursement rate in inpatient care than NCMS, which should improve the inpatient care utilization rate of rural middle-aged and older people, as confirmed by previous research results (20). The possible explanation is that there still be a gap in the distribution of medical resources between rural and urban areas, especially high-quality medical resources and inpatient services. Thus, the URRBMI impact on rural residents is only reflected in outpatient care utilization. Meanwhile, the differences in data collection, study samples, and populations would also lead to different findings, so further research is needed to validate these findings (42). In addition, the URRBMI significantly impacted healthcare utilization in older adults, but not in the middle-aged population, which implied that healthcare utilization was closely related to age. There may be two reasons: one is that as the age increases, the diseases appear and health gets worse, and the other is that older residents are more sensitive to the change in reimbursement rates. The higher reimbursement rates of the URRBMI scheme help to release the demand for healthcare among older adults.
Third, this study also finds that all of the CI values in the treatment group were negative after the URRBMI integration, indicating that the lower-economic participants had more healthcare utilization than the higher-economic participants. The findings are inconsistent with the previous study. Wang et al. (43) found that the richest utilize more outpatient care in URRBMI. Besides the differences in data and methods used, the time scopes of the study may also account for these different findings (36). By decomposing the concentration index, we found that URRBMI integration contributed to the pro-poor inequality in healthcare utilization. The effect is in alignment with the original intention of the URRBMI scheme. Furthermore, it is worth noting that poor health status has the greatest contribution to the inequalities of outpatient and inpatient care, which is consistent with earlier findings (44). This further proves that personal health status is the first driving force for healthcare utilization (44). Therefore, the government should play the role of URRBMI for middle-aged and older residents with poor health status, adopting better means to guide medical choices and promoting health.
There are several limitations to this study. First, since the decision to URRBMI integration in each province was affected by many unobservable factors (such as the willingness of local leaders), it is hard to avoid the problem of endogeneity (43). Second, the information on healthcare utilization was self-reported, which may have led to a reporting bias, especially for older respondents. Third, the post-integration period is only available for 1 year in 2018. The URRBMI integration effects may have a time lag effect that is not significant in the short term, which requires further evaluation with more observation time.
Conclusion
This study shows that URRBMI integration has decreased outpatient care utilization and has improved the number of inpatient visits. Furthermore, the integration affected healthcare utilization for older adults. The URRBMI contributed to the pro-poor inequality in healthcare utilization. However, our findings also shed light on several areas where challenges remain. Therefore, comprehensive measures, such as improving the benefits structure of URRBMI to cover more outpatient diseases, optimizing the allocation of medical resources, and aiding vulnerable groups, should be taken during the post-integration period. The government should play the role of URRBMI for residents and adopt better means to guide medical choices. Improving the URRBMI scheme would be an important part of healthcare reform in China. Meanwhile, the experience in China may have broad implications for other low and middle-income countries that aim to reduce healthcare utilization inequality and achieve the goal of UHC.
Author Contributions
LZ contributed to the study conception and design. RC performed data analysis and interpretation, and drafting of the article. YF reviewed and supervised the paper. All authors have read and agreed to the published version of the manuscript.
Funding
This study was supported by the National Natural Science Foundation of China (No. 81973144), the Science and Technology Innovation Think Tank Youth Program (No. 2021ZZZLFZB1207146), and the Social Science Foundation of the Fujian Province, China (FJ2022C047).
Conflict of Interest
The authors declare that they do not have any conflicts of interest.
Supplementary Material
The Supplementary Material for this article can be found online at: https://www.ssph-journal.org/articles/10.3389/ijph.2023.1605521/full#supplementary-material
References
1.Who. The World Health Report: Health Systems Financing: The Path to Universal Coverage. World Health Organization (2010).
2. Lagomarsino, G, Garabrant, A, Adyas, A, Muga, R, and Otoo, N. Moving towards Universal Health Coverage: Health Insurance Reforms in Nine Developing Countries in Africa and Asia. Lancet (2012) 380:933–43. doi:10.1016/s0140-6736(12)61147-7
4. Cheng, TM. Early Results of China's Historic Health Reforms: the View from Minister Chen Zhu. Interview by Tsung-Mei Cheng. Health Aff (2012) 31:2536–44. doi:10.1377/hlthaff.2012.1017
5. Li, C, Dou, L, Wang, H, Jing, S, and Yin, A. Horizontal Inequity in Health Care Utilization Among the Middle-Aged and Elderly in China. Int J Environ Res Public Health (2017) 14:842. doi:10.3390/ijerph14080842
6. Huang, X, and Wu, BX. Impact of Urban-Rural Health Insurance Integration on Health Care: Evidence from Rural China. China Econ Rev (2020) 64:101543. doi:10.1016/j.chieco.2020.101543
7. Li, D, Zhang, J, Yang, J, Xu, Y, Lyu, R, Zhong, L, et al. Socio-economic Inequalities in Health Service Utilization Among Chinese Rural Migrant Workers with New Cooperative Medical Scheme: a Multilevel Regression Approach. Bmc Public Health (2022) 22:1110. doi:10.1186/s12889-022-13486-1
8. Zhou, Q, He, Q, Eggleston, K, and Liu, GG. Urban-rural Health Insurance Integration in china: Impact on Health Care Utilization, Financial Risk protection, and Health Status. Appl Econ (2022) 54:2491–509. doi:10.1080/00036846.2021.1998323
9. Mao, WH, Zhang, YG, Xu, L, Miao, ZW, Dong, D, and Tang, SL. Does Health Insurance Impact Health Service Utilization Among Older Adults in Urban China? A Nationwide Cross-Sectional Study. Bmc Health Serv Res (2020) 20:630. doi:10.1186/s12913-020-05489-8
10. Pan, J, Tian, S, Zhou, Q, and Han, W. Benefit Distribution of Social Health Insurance: Evidence from china's Urban Resident Basic Medical Insurance. Health Policy Plan (2016) 31:853–9. doi:10.1093/heapol/czv141
11.Bulletin of the Seventh National Census. Bulletin of the Seventh National Census (2021). Available at: http://www.gov.cn/guoqing/2021-05/13/content_5606149.htm (Accessed August 20, 2022).
12. Kondo, A, and Shigeoka, H. Effects of Universal Health Insurance on Health Care Utilization, and Supply-Side Responses: Evidence from Japan. J Public Econ (2013) 99:1–23. doi:10.1016/j.jpubeco.2012.12.004
13. Somkotra, T. Measurement and Explanation of Horizontal (In)Equity in Health Care Utilization Among Thais after Universal Coverage Policy Implementation. Asia-Pacific J Public Health (2011) 23:980–95. doi:10.1177/1010539509360674
14. Cheng, SH, and Chiang, TL. The Effect of Universal Health Insurance on Health Care Utilization in Taiwan - Results from a Natural experiment. Jama-Journal Am Med Assoc (1997) 278:89–93. doi:10.1001/jama.278.2.89
15. Keane, C, Regan, M, and Walsh, B. Failure to Take-Up Public Healthcare Entitlements: Evidence from the Medical Card System in Ireland. Soc Sci Med (2021) 281:114069. doi:10.1016/j.socscimed.2021.114069
16. Yan, XL, Liu, YL, Cai, M, Liu, QQ, Xie, XQ, and Rao, KQ. Trends in Disparities in Healthcare Utilisation between and within Health Insurances in China between 2008 and 2018: a Repeated Cross-Sectional Study. Int J Equity Health (2022) 21:30. doi:10.1186/s12939-022-01633-4
17. Zhang, LY, Cheng, XM, Tolhurst, R, Tang, SL, and Liu, XY. How Effectively Can the New Cooperative Medical Scheme Reduce Catastrophic Health Expenditure for the Poor and Non-poor in Rural China? Trop Med Int Health (2010) 15:468–75. doi:10.1111/j.1365-3156.2010.02469.x
18. Ma, JD, Xu, J, Zhang, ZG, and Wang, J. New Cooperative Medical Scheme Decreased Financial burden but Expanded the gap of Income-Related Inequity: Evidence from Three Provinces in Rural China. Int J Equity Health (2016) 15:72. doi:10.1186/s12939-016-0361-5
19. Liu, P, Guo, W, Liu, H, Hua, W, and Xiong, LP. The Integration of Urban and Rural Medical Insurance to Reduce the Rural Medical burden in China: a Case Study of a County in Baoji City. Bmc Health Serv Res (2018) 18:796. doi:10.1186/s12913-018-3611-y
20. Ma, WC, From New Rural Cooperative Medical Insurance to Basic Medical Insurance for Urban and Rural Residents: An Analysis of Policy Effects of the UrbanRural Medical Insurance Coordination System [in Chinese]. China Econ Stud (2021) 04:146–57. doi:10.19365/j.issn1000-4181.2021.04.11
21. Li, CF, Tang, CX, and Wang, HP. Effects of Health Insurance Integration on Health Care Utilization and its Equity Among the Mid-aged and Elderly: Evidence from China. Int J Equity Health (2019) 18:166. doi:10.1186/s12939-019-1068-1
22. Ren, Y, Zhou, Z, Cao, D, Ma, BH, Shen, C, Lai, S, et al. Did the Integrated Urban and Rural Resident Basic Medical Insurance Improve Benefit Equity in China? Value Health : J Int Soc Pharmacoeconomics Outcomes Res (2022) 25:1548–58. doi:10.1016/j.jval.2022.03.007
23. Su, D, Chen, Y-C, Gao, H-X, Li, HM, Chang, JJ, Jiang, D, et al. Effect of Integrated Urban and Rural Residents Medical Insurance on the Utilisation of Medical Services by Residents in China: a Propensity Score Matching with Difference-In-Differences Regression Approach. Bmj Open (2019) 9:e026408. doi:10.1136/bmjopen-2018-026408
24. Wang, JH, Zhu, H, Liu, H, Wu, K, Zhang, X, Zhao, M, et al. Can the Reform of Integrating Health Insurance Reduce Inequity in Catastrophic Health Expenditure? Evidence from China. Int J Equity Health (2020) 19:49. doi:10.1186/s12939-020-1145-5
25. Zhao, Y, Hu, Y, Smith, JP, Strauss, J, and Yang, G. Cohort Profile: The China Health and Retirement Longitudinal Study (CHARLS). Int J Epidemiol (2014) 43:61–8. doi:10.1093/ije/dys203
26. Ye, W, and Rodriguez, JM. Highly Vulnerable Communities and the Affordable Care Act: Health Insurance Coverage Effects, 2010-2018. Soc Sci Med (2021) 270:113670. doi:10.1016/j.socscimed.2021.113670
27. Gong, CH, Kendig, H, and He, XJ. Factors Predicting Health Services Use Among Older People in China: An Analysis of the China Health and Retirement Longitudinal Study 2013. Bmc Health Serv Res (2016) 16:63. doi:10.1186/s12913-016-1307-8
28. Zhang, CC, Lei, XY, Strauss, J, and Zhao, YH. Health Insurance and Health Care Among the Mid-aged and Older Chinese: Evidence from the National Baseline Survey of CHARLS. Health Econ (2017) 26:431–49. doi:10.1002/hec.3322
29. Zhou, ZL, Zhao, YX, Shen, C, Lai, S, Nawaz, R, and Gao, JM. Evaluating the Effect of Hierarchical Medical System on Health Seeking Behavior: A Difference-In-Differences Analysis in China. Soc Sci Med (2021) 268:113372. doi:10.1016/j.socscimed.2020.113372
30. Zeng, Y, Wan, Y, Yuan, Z, and Fang, Y. Healthcare-Seeking Behavior Among Chinese Older Adults: Patterns and Predictive Factors. Int J Environ Res Public Health (2021) 18:2969. doi:10.3390/ijerph18062969
31. Andersen, RM. Revisiting the Behavioral-Model and Access to Medical-Care - Does it Matter. J Health Soc Behav (1995) 36:1–10. doi:10.2307/2137284
32. Xu, K. Analysing Health Equity Using Household Survey Data: a Guide to Techniques and Their Implementation. Bull World Health Organ Int J Public Health (2008) 86(10):816. doi:10.2471/blt.08.052357
33. Wagstaff, A, van Doorslaer, E, and Watanabe, N. On Decomposing the Causes of Health Sector Inequalities with an Application to Malnutrition Inequalities in Vietnam. J Econom (2003) 112:207–23. doi:10.1016/s0304-4076(02)00161-6
34. Kaestner, R, Garrett, B, Chen, JJ, Gangopadhyaya, A, and Fleming, C. Effects of ACA Medicaid Expansions on Health Insurance Coverage and Labor Supply. J Policy Anal Manage (2017) 36:608–42. doi:10.1002/pam.21993
35. Cai, XQ, Lu, Y, Wu, MQ, and Yu, LH. Does Environmental Regulation Drive Away Inbound Foreign Direct Investment? Evidence from a Quasi-Natural experiment in China. J Develop Econ (2016) 123:73–85. doi:10.1016/j.jdeveco.2016.08.003
36. Zhang, AW, Nikoloski, Z, and Mossialos, E. Does Health Insurance Reduce Out-Of-Pocket Expenditure? Heterogeneity Among China's Middle-Aged and Elderly. Soc Sci Med (2017) 190:11–9. doi:10.1016/j.socscimed.2017.08.005
37. Han, JQ, Zhang, XD, and Meng, YY. Out-Patient Service and In-Patient Service: The Impact of Health Insurance on the Healthcare Utilization of Mid-aged and Older Residents in Urban China. Risk Manage Healthc Pol (2020) 13:2199–212. doi:10.2147/rmhp.S273098
38. Chen, S, Lin, Z, Fan, X, Li, J, Xie, Y-J, and Hao, C. The Comparison of Various Types of Health Insurance in the Healthcare Utilization, Costs and Catastrophic Health Expenditures Among Middle-Aged and Older Chinese Adults. Int J Environ Res Public Health (2022) 19:5956. doi:10.3390/ijerph19105956
39. Cheng, L, Liu, H, Zhang, Y, Shen, K, and Zeng, Y. The Impact of Health Insurance on Health Outcomes and Spending of the Elderly: Evidence From China's New Cooperative Medical Scheme. Health Econ (2015) 24:672–91. doi:10.1002/hec.3053
40. Tian, WX, Wu, B, Yang, YH, Lai, Y, Miao, W, Zhang, X, et al. Degree of protection provided by Poverty Alleviation Policies for the Middle-Aged and Older in China: Evaluation of Effectiveness of Medical Insurance System Tools and Vulnerable Target Recognition. Health Res Pol Syst (2022) 20(1):129. doi:10.1186/s12961-022-00929-9
41. Lili, Z. Has Health Insurance System Changed Health Care Seeking Behavior? Evidence from Chinese CHNS [in Chinese]. Public Finance Res (2018) 10:100–13. doi:10.13497/j.cnki.is.2018.10.009
42. Fan, XJ, Su, M, Si, YF, Zhao, YX, and Zhou, ZL. The Benefits of an Integrated Social Medical Insurance for Health Services Utilization in Rural China: Evidence from the China Health and Retirement Longitudinal Study. Int J Equity Health (2021) 20:126. doi:10.1186/s12939-021-01457-8
43. Wang, ZW, Chen, YC, Pan, TY, Liu, XD, and Hu, HW. The Comparison of Healthcare Utilization Inequity between URRBMI and NCMS in Rural China. Int J Equity Health (2019) 18:90. doi:10.1186/s12939-019-0987-1
Keywords: China, health insurance, difference-in-difference, healthcare utilization, inequality
Citation: Zhang L, Chen R and Fang Y (2023) Effects of Urban and Rural Resident Basic Medical Insurance on Healthcare Utilization Inequality in China. Int J Public Health 68:1605521. doi: 10.3389/ijph.2023.1605521
Received: 24 October 2022; Accepted: 03 February 2023;
Published: 16 February 2023.
Edited by:
Calogero Guccio, University of Catania, ItalyReviewed by:
Henry Lucas, University of Sussex, United KingdomHaijing Hao, Bentley University, United States
Copyright © 2023 Zhang, Chen and Fang. This is an open-access article distributed under the terms of the Creative Commons Attribution License (CC BY). The use, distribution or reproduction in other forums is permitted, provided the original author(s) and the copyright owner(s) are credited and that the original publication in this journal is cited, in accordance with accepted academic practice. No use, distribution or reproduction is permitted which does not comply with these terms.
*Correspondence: Ya Fang, fangya@xmu.edu.cn