- 1Guangdong Provincial Key Laboratory of Tropical Disease Research, Department of Epidemiology, School of Public Health, Southern Medical University, Guangzhou, China
- 2School of Medicine, Southern University of Science and Technology, Shenzhen, China
- 3Public Health Division, Hospital of Zhongluotan, Guangzhou, China
- 4Department of Anaesthesiology, Nanfang Hospital, Southern Medical University, Guangzhou, China
- 5Bioscience and Biomedical Engineering Thrust, Systems Hub, The Hong Kong University of Science and Technology (Guangzhou), Guangzhou, China
- 6Bioscience and Biomedical Engineering Thrust, Systems Hub, The Hong Kong University of Science and Technology, Hong kong, Hong Kong SAR, China
- 7Department of Endocrinology and Metabolism, Nanfang Hospital, Southern Medical University, Guangzhou, China
Objectives: To examine the association between visit-to-visit blood pressure variability (BPV) and incident diabetes mellitus (DM) risk in a Chinese population.
Methods: Data comes from China Health and Nutrition Survey (n = 15,084). BPV was estimated as the average real variability (ARV) using at least three BP measurements from the year preceding the event and was divided into quartiles. Participants were also categorized into 9 groups on the basis of combinations of systolic BPV (SBPV) and diastolic BPV (DBPV) tertiles. Cox proportional hazards regression models were used.
Results: During a median follow-up of 16.8 years, 1,030 (6.8%) participants developed diabetes (incidence rate: 4.65/1,000 person-years). The HRs (95% CIs) for the highest quartile (vs. the lowest quartile) of SBPV and DBPV were 1.60 (1.30–1.97) and 1.37 (1.13–1.67), respectively. Participants with both highest SBPV and DBPV tertile had an ≈89% higher risk of DM (HR, 1.89; 95% CI, 1.47–2.42) compared with those in the both SBPV and DBPV tertile 1 group.
Conclusion: Higher SBP ARV and DBP ARV were independently associated with increased risk of incident DM, which was augmented when both presented together.
Introduction
Diabetes mellitus (DM) is a worldwide pandemic, altering the disease profile around the globe due to a higher incidence of diabetes-specific complications, such as kidney failure and peripheral arterial disease (1). Indeed, DM and its complications have contributed tremendously to the burden of mortality and disability worldwide (2). There were estimated 537 million people with DM worldwide in 2021, and this number is expected to increase to 783 million by 2045 (3). In particular, China has the world’s largest diabetic population, with a prevalence of 12.8% in 2017, which represents more than an estimated 168 million people in China with DM, resulting in a major public health challenge (4). Thus, early detection of patients at high risk for DM is urgently needed.
Elevated blood pressure (BP), which is well known to be associated with cardiovascular disease, is also emerging as a risk factor for DM (5, 6). However, BP fluctuates continually, and these fluctuations tend to remain consistent within patients (7, 8). In the past, BP fluctuations have often been dismissed as random fluctuations and considered to be a limitation of measuring BP in clinical settings (9). In this context, visit-to-visit variability (VVV) of BP, an index that reflects the variability in BP between visits, is being increasingly used as a newer method to evaluate intraindividual BP fluctuations, which has the potential to avoid underestimation of the true risk of elevated BP in the traditional correlation between baseline BP and outcomes of interest, such as cardiovascular disease, mortality, etc (10, 11).
Existing evidence has linked an increased BPV to an increased risk of DM through impaired glucose tolerance following sympathetic overactivity and autonomic imbalance (12). Several observational studies have also demonstrated that high BPV is significantly associated with pre-diabetes and DM (13, 14). However, those studies focused on short-term BPV, and the impact of long-term BPV on DM is less clear. Furthermore, BPV shows differences among different ethnic populations (15), and relevant studies among Asian populations are rare. Although a prior study using data from a nationwide Japanese population demonstrated that visit-to-visit systolic blood pressure variability (SBPV) is higher in subjects with new-onset DM (16), the association of diastolic blood pressure variability (DBPV) on the incidence of DM was less well understood. Therefore, to comprehensively examine the impact of long-term BPV on the risk of DM, the present study aimed to respectively evaluate the association between visit-to-visit SBPV and DBPV and the incidence of DM, as well as the association of different combinations of SBPV/DBPV tertiles on DM risk using a 17-year longitudinal follow-up study of Chinese men and women.
Methods
The Strengthening the Reporting of Observational Studies in Epidemiology (STROBE) checklist for research reporting of observational studies was followed in this study (Supplementary Table S1).
Study Population
The China Health and Nutrition Survey (CHNS) is an ongoing large-scale, longitudinal, prospective cohort designed to examine the effects of health, nutrition, and family-planning policies and programs in China. Individuals of multiple ages were selected from rural, urban and suburban areas across 15 provinces and municipal cities, using a multistage and random cluster design. A range of demographic, socioeconomic, lifestyle, and health information, including BP, were collected in each wave. The details of the CHNS study have been reported elsewhere (17). This retrospective secondary data analysis was based on 10 waves (from 15 January, 1989 to 13 December 2015) of CHNS survey data. As of 13 December 2015, a total of 39,674 individuals (aged 0–92 years) covering 9,552 households were enrolled. In our study, the following individuals were excluded: participants who had prevalent DM at baseline (n = 450) and participants who had fewer than 3 visits with BP measurements (n = 24,140). Ultimately, the study sample consisted of 15,084 participants (Figure 1).
The study was approved by the institutional review committees of the Chinese Center for Disease Control and Prevention, and written informed consent was obtained from all participants.
Baseline BP and Long-Term BPV Measurements
At each wave since 1989, BP was measured according to standard procedure (18), and the average interval of BP measurements across waves was 2.9 years. After at least a 5-min rest, BP was measured by trained health workers or nurses using a standard mercury sphygmomanometer on the right arm in the sitting position with the cuff maintained at heart level. BP was measured three times on one visit, and the three measurements were separated by at least a 30-s interval, during which the right arm was raised up for 5–6 s. BP values measured three times on one visit were averaged and reported as the BP values in this study.
For SBP and DBP, we separately, calculated the long-term mean and BP variability across visits. Based on previous studies (19–22), visit-to-visit BPV was expressed as average real variability (ARV), standard deviation (SD), and coefficient of variation (CV). The BPV formulas were as follows (23): ARV =
Ascertainment of Incident Diabetes
At each follow-up, the participants were asked to report their previous history of diabetes with a questionnaire-based interview based on the following questions: 1) “has a doctor ever told you that you suffer from diabetes? 2) have you received any of the following glucose-lowering treatments, such as a special diet, weight control, oral medications, insulin injections, traditional Chinese medicine, home remedies, or Qi Gong (spiritual method)?” For each visit, participants were diagnosed as DM if at least one of the two answers was yes. In addition, blood samples were collected from patients after overnight fasting by trained nurses and were analyzed at a national central laboratory in Beijing [International Standards Organization (ISO) medical laboratory accreditation certificate 15189:2007] under strict quality control, and the data were available only in 2009. Fasting plasma glucose (FPG) was measured using the glucose oxidase–phenol and 4-aminophenazone (GOD–PAP) method (Randox Laboratories, Ltd., Crumlin, Co., Antrim, United Kingdom) (24). Hemoglobin A1c (HbA1c) was assessed by high-performance liquid chromatography (HLC-723 G7 analyzer; Tosoh Corporation, Tokyo, Japan) (24). Therefore, an additional third criterion (i.e., FPG ≥7.0 mmol/L [126 mg/dL], and/or HbA1c ≥ 6.5% [48 mmol/mol]) (25) was added for outcome ascertainment in 2009. Thus, diabetes diagnosis was ascertained in 2009 if at least one of the two answers concerning diabetes was yes or the third criterion was met.
Covariates
A standard structured questionnaire was used to collect detailed information on sociodemographic factors, lifestyle factors and medical history. In this study, all baseline covariates with a P value less than 0.1 from the univariate analysis were subsequently included in the stepwise logistic regression analysis to identify the most relevant variables contributing to the risk of DN. The result was presented in Supplementary Table S2. Apart from these covariates, several potential cofounders reported to be associated with DM risk were also considered (26–28). Thus, the following covariates were included in our models: age (continuous), sex (men or women), nationality (Han or Minority), region of residence (urban or suburban/rural), education level, smoking status, alcohol consumption, physical activity, BMI (continuous), antihypertensive drugs (yes or no), history of hypertension (yes or no), mean BP (SBP for SBPV and DBP for DBPV). Education level was grouped into three categories: low (graduated from primary school or not educated); medium (graduated from junior middle school or high middle school); high (graduated from college or higher). The highest education level reported in the survey questionnaire was used. Participants were asked about activity levels, which interviewers categorized into five levels (very light, light, moderate, heavy, and very heavy) based on respondents’ descriptions and time spent sitting, standing, walking and lifting heavy loads (29). In our study, we categorized physical activity level as light (very light or light), moderate, or heavy (heavy or very heavy). Smoking status was categorized as non-/ever-smoking and smoking. Alcohol drinking status was categorised as non-drinking and drinking, according to the question “Have you consumed alcohol (beer, wine or other alcoholic beverage) during the past year (yes, no)”? (30). Body mass index (BMI) was measured as weight in kilograms divided by measured height in meters squared. Hypertension was defined by a self-reported diagnosis of hypertension or SBP ≥140 mmHg or DBP ≥90 mmHg based on the 2018 Chinese guidelines for the management of hypertension (31).
Statistical Methods
Continuous variables are presented as median (25% quartile, 75% quartile), and categorical variables are presented as numbers and percentages. Non-parametric test or Chi-square test were used to assess the difference. The incidence rate of DM was calculated by dividing the number of incident cases by the total follow-up duration (per 1,000 person-years). Cox proportional hazards regression models were used to estimate hazard ratios (HRs) with 95% confidence intervals (CIs) for incident DM. Missing values of all the adjusted covariates accounted for <15.0% and were imputed using the multiple imputation of chained equations (MICEs) method. Characteristics including age, sex, region of residence, and cumulative mean SBP and DBP, were used to impute the missing values. We created 20 imputed data sets and pooled the results using the “mi impute chained” procedure in STATA (version 15; StataCorp, College Station, Texas). The time since the date of the beginning of follow-up was used as the underlying timescale. Participants were followed up from the date they were enrolled in this cohort until the time of incident DM, death, loss to follow-up or were censored at the end of follow-up, whichever occurred first. The proportional hazards assumption was examined using statistical tests and graphical diagnostics based on the scaled Schoenfeld residuals. We divided all measures of BPV into quartiles, using the lowest quartile of BPV as the reference for the regression models. Multivariable adjusted Cox proportional hazards models were applied. Model 1 was adjusted for age, sex, and ethnicity. Model 2 was further adjusted for region of residence, education level, smoking status, alcohol consumption, physical activity level, BMI, and history of hypertension. Model 3 was adjusted for model 2 covariates plus the mean BP (mean SBP for SBPV and mean DBP for DBPV). Tests for linear trend were also performed by entering the median value of each quartile of BPV as a continuous variable in the models. Participants were also divided into 9 groups based on combinations of SBP ARV and DBP ARV tertiles.
We conducted prespecified subgroup analyses within strata of the following factors: age (<50 years or ≥50 years), sex, ethnicity (Han nationality or minority), smoking (yes or no), BMI (<25 kg/m2 or ≥25 kg/m2), hypertension (yes or no), baseline SBP (<140 mmHg or ≥140 mmHg) and baseline DBP (<90 mmHg or ≥90 mmHg). The results of the subgroup analyses are presented as HRs (95% CIs) of the highest quartile (Q4) compared with the lowest quartile (Q1). The statistical significance of the interactions was assessed by adding a multiplicative term to the Cox models. We also performed several sensitivity analyses to test the robustness of the primary results, including 1) the exclusion of those who received antihypertensive drugs at baseline; and 2) use of the DM definition that only includes self-reports of diabetes diagnosis and/or receipt of any of the glucose-lowering treatment.
Statistical analyses were performed using Stata (version 15; StataCorp, College Station, Texas), and a P value < 0.05 was considered to indicate statistical significance.
Results
Characteristics of the Study Population
A total of 15,084 participants with at least three waves of BP measurements and without DM at baseline were included in this study. The baseline characteristics of those in the study population who did or did not develop a DM event are presented in Table 1. Overall, the mean age was 31.8 years (SD: 18.4), and women made up 50.5% of the population. The median baseline SBP and DBP was 113.4 mmHg (IQR: 104.0–120.0) and 73.7 mmHg (IQR: 68.7–80.0), respectively. No missing value for the SD, CV, and ARV of BP were found. A comparison of baseline characteristics between included and not included participants is shown in Supplementary Table S3. Supplementary Tables S4, S5 also show the baseline characteristics of these populations categorized by SBP/DBP quartiles. Supplementary Figure S1 indicates that the mean SBP and DBP rose continuously across visits during the follow-up period in both men and women.
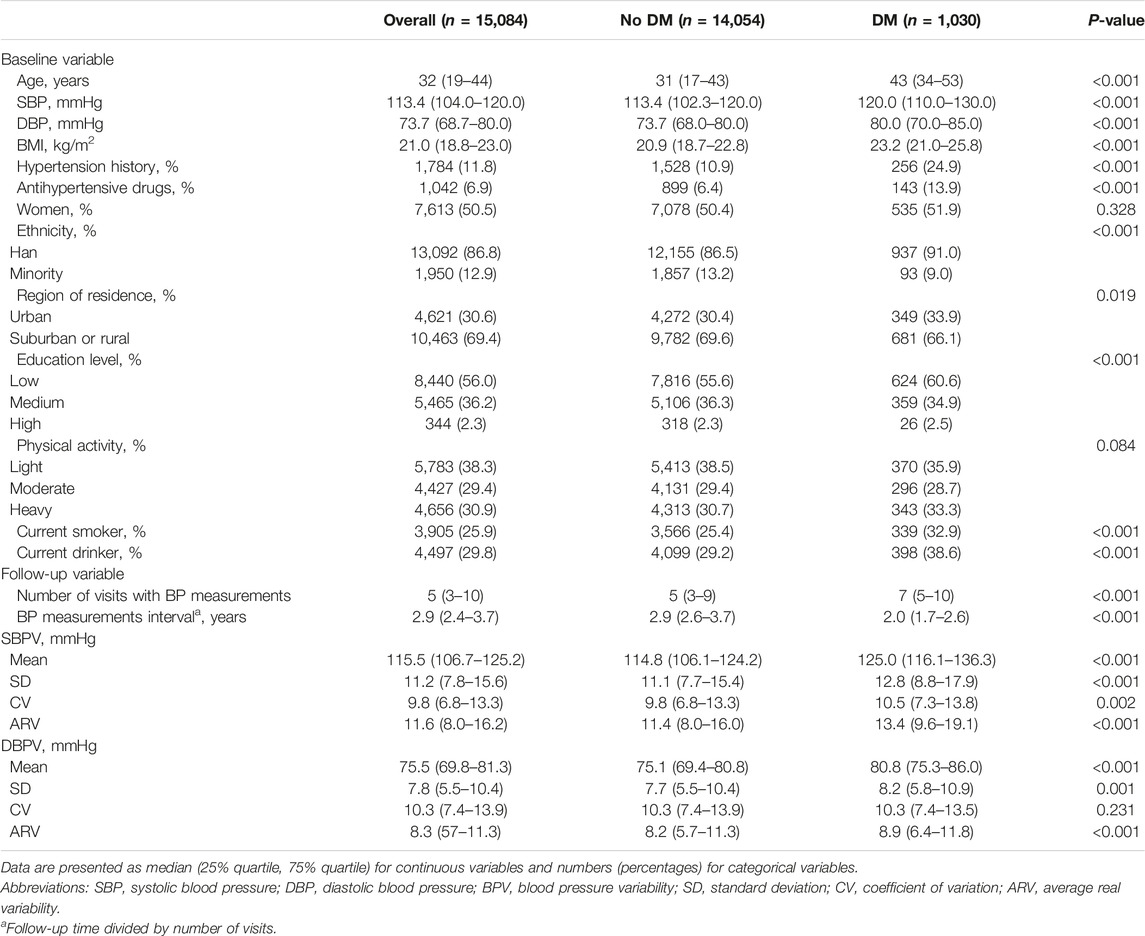
TABLE 1. Characteristics of participants by incident DM status. China Health and Nutrition Survey, China, 1989–2015.
Blood Pressure Variability and Risk of DM
Table 2 shows the number and incidence rate of DM events by quartiles of BPV. During a median follow-up of 16.8 years (range: 3.4–26.2), 1,030 (6.8%) participants developed DM (incidence rate: 4.65 per 1,000 person-years). Table 2 also shows the results of the multivariable Cox proportional hazard regressions. A significantly higher risk of DM was observed with higher ARV quartiles in all three adjusted models, although a plateau appeared at the highest DBP ARV quartile in the fully adjusted model (model 3). Specifically, in model 3, SBP ARV quartiles 2 through 4 (compared with the first quartile) were associated with an increased risk of incident DM, with adjusted HRs of 1.27 (95% CI: 1.02–1.57), 1.41 (95% CI: 1.14–1.74), and 1.60 (95% CI: 1.30–1.97), respectively (P for trend <0.01). Compared with the lowest DBP ARV quartile, the fully adjusted HRs (95% CIs) of quartiles 2 to 4 were 1.28 (95% CI: 1.05–1.55), 1.37 (95% CI: 1.13–1.67) and 1.37 (95% CI: 1.13–1.67), respectively (P for trend = 0.02). When ARV was modeled as a continuous variable, a 1-SD increment in both SBP ARV (HR = 1.11, 95% CI 1.04–1.17) and DBP ARV (HR = 1.09, 95% CI 1.02–1.15) was also significantly associated with an increased risk of DM. Furthermore, a strong, significant trend of increasing DM risk with increasing quartiles of both SBP and DBP variability was observed in Table 2.
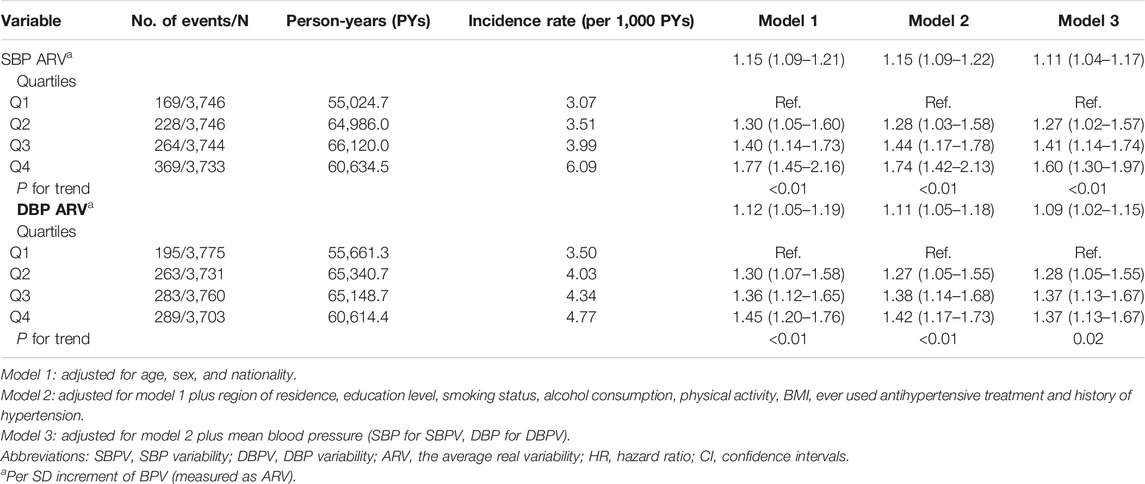
TABLE 2. HRs and 95% CIs for incident diabetes by BPV quartiles. China Health and Nutrition Survey, China, 1989–2015.
We also evaluated the association of different combinations of SBPV/DBPV tertiles with the risk of DM (Figure 2). Compared to participants with both the lowest SBPV and DBPV, those with both the highest SBPV and DBPV tertiles had an elevated risk of DM. The multivariable HRs (95% CIs) of the highest SBPV tertile in each DBP ARV tertile was 1.42 (95% CI: 1.01–2.00), 1.96 (95% CI: 1.49–2.58) and 1.89 (95% CI: 1.47–2.42), respectively, compared with the first SBPV tertile in the first DBP ARV tertile. The highest SBPV tertile in the second DBP ARV tertile showed the highest risk, followed by the third SBPV tertile in the highest DBP ARV tertile.
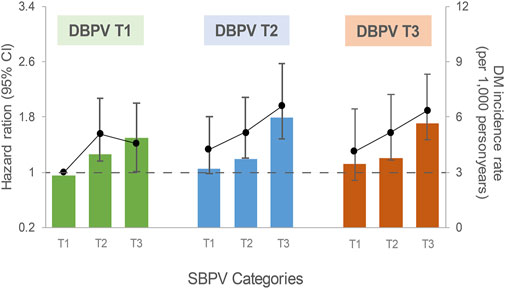
FIGURE 2. Association between SBPV and incident diabetes by DBPV tertiles. China Health and Nutrition Survey, China, 1989–2015. Models were adjusted for age, sex, nationality, region of residence, education level, smoking status, alcohol consumption, physical activity, BMI, ever used antihypertensive treatment, history of hypertension and cumulative mean BP. The solid black lines represent HRs, and colored columns represent the incidence rate of DM (per 1,000 person-years). Abbreviations: SBPV, systolic blood pressure variability; DBPV, diastolic blood pressure variability; CI, confidence intervals.
When alternative variability indexes were used to assess BPV (e.g., SD and CV), the associations were generally diluted and even became null in the fully adjusted models, regardless of the SD or CV (Supplementary Table S6).
Subgroup Analysis
Stratified analyses by age, sex, smoking and drinking status, BMI cutoff, hypertension and baseline SBP/DBP cutoffs were conducted (Figure 3). In general, the highest SBPV and DBPV (quartile 4) was significantly associated with a higher risk of DM across various subgroups. However, a higher adjusted HR of DM was observed among women (P of 0.05 and 0.01 for SBPV and DBPV interaction, respectively).
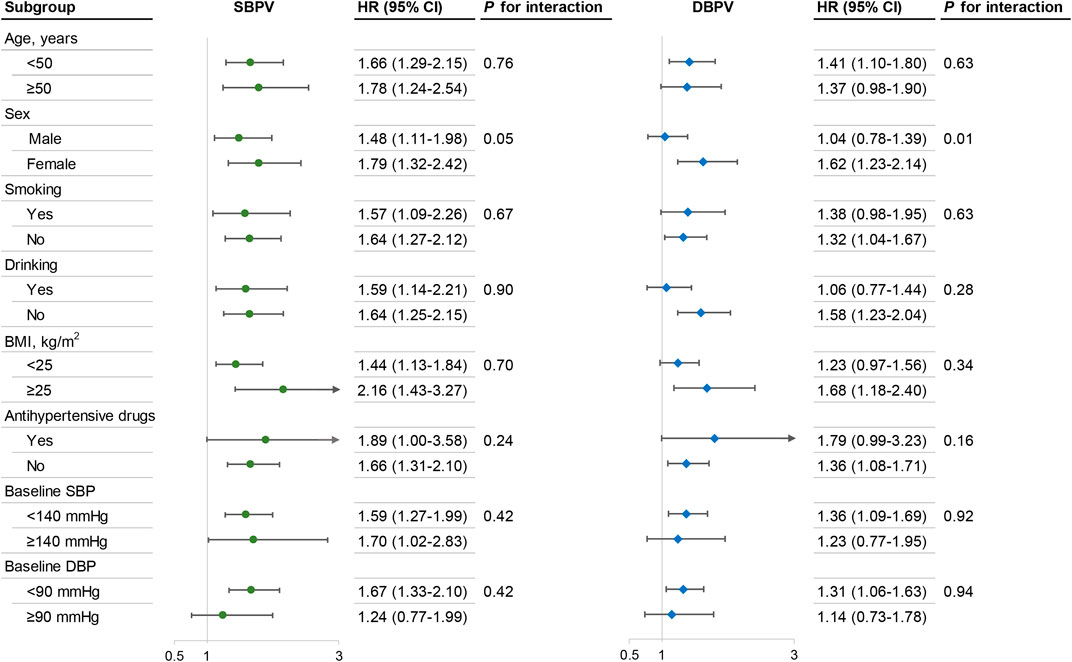
FIGURE 3. Subgroup analysis of the association between BPV and the risk of diabetesa. China Health and Nutrition Survey, China, 1989–2015. Models were adjusted for age, sex, nationality, region of residence, education level, smoking status, alcohol consumption, physical activity, BMI, ever used antihypertensive treatment, history of hypertension, and cumulative mean BP (SBP for SBPV, DBP for DBPV). Abbreviations: SBPV, systolic blood pressure variability; DBPV, diastolic blood pressure variability; BMI, body mass index; HR, hazard ratio; CI, confidence intervals. aThe results are presented in terms of the highest vs. the lowest quartile of BPV.
Since sex tended to be an effect modifier, we performed additional analyses regarding the sex-specific association of BPV with DM risk (Supplementary Table S7). Overall, the association between BPV and incident DM remained significant with the exception of DBPV among men.
Sensitivity Analysis
After excluding participants who had received antihypertensive drugs at baseline, the primary results remained largely unchanged (Supplementary Table S8). Likewise, the main results remained robust, though weakened, when the DM definition only included self-reports of a diabetes diagnosis and/or receipt of any glucose-lowering treatment (Supplementary Table S9).
Discussion
Based on this large-scale, longitudinal study of community-dwelling Chinese men and women, we found that an increased BPV, both SBPV and DBPV, as measured by an increased ARV of intraindividual BP values, was associated with an increased risk of incident DM, independent of baseline characteristics, including hypertension and mean BP. Subgroup analyses revealed that the impact of visit-to-visit BPV on the risk of DM was stronger in women than men.
Our findings strengthen the public health importance of long-term BPV, which might provide additional prognostic information concerning mean BP levels and help to improve risks discrimination for adverse outcomes (7). Until now, few studies have evaluated the association between BPV and incident DM. A previous study based on a large Japanese population demonstrated that a high within-visit BPV was significantly associated with the prevalence of pre-diabetes and DM independent of the mean BP (13). According to other cross-sectional data from the Maastricht study (14), increased 24-h BPV and 7-day home BPV, assessed by the SD of BP, were also linked to an increased risk of type 2 DM. However, those studies merely focused on short-term BPV, and the impact of long-term BPV on DM was less clear. Another prospective study of the Japanese general population reported a positive correlation between long-term SBPV and new-onset DM (16), which is consistent with our findings. Unfortunately, this study did not report the results of DBPV nor the combination of high SBPV and DBPV regarding the risk of DM, and the follow-up period (3 years) was relatively short.
The biological mechanisms underlying the association of long-term BPV with DM remain uncertain. This ambiguity could be partly explained by the contribution of some of the factors that impact long-term BPV to the risk of hyperglycemia, including higher sympathetic nerve activity, lower socioeconomic status, low physical activity, an unhealthy diet, smoking, and sleep apnea (32–36). In addition, a higher long-term BPV may reflect latent metabolic abnormalities (e.g., central obesity and metabolic syndrome [MetS]) and thus relate to DM risk (37, 38). The relationship between long-term BPV and DM can also be explained by BPV as a promoter of endothelial dysfunction and autonomic dysregulation, which may lead to end-organ damage and hyperglycemia (39).
Interestingly, our study also revealed that SBPV had a higher impact than DBPV on DM risk. This result may be partly explained by arterial stiffness, an important factor associated with DM risk, which has been postulated as one of the major causes of SBPV (40). Accumulating evidence in humans and animals has linked arterial stiffness to MetS and its components (e.g., hypertension, hyperglycemia, and obesity), as well as metabolic changes, such as metabolite accumulation, insulin resistance and high free fatty acid concentrations, which may increase the risk of DM (41–44). Furthermore, the highest risk of DM was observed in participants with the highest SBPV tertile and second DBPV tertile, instead of the highest DBPV tertile. The underlying mechanism may be as follows: when DBPV cut-off points exceeded certain limits, components of MetS may not increase consistently but instead tend to reach a plateau (45), leading to a cushion effect of metabolic abnormalities on the risk of DM among those with the highest DBPV (46). Further investigations should highlight the mechanism responsible for the interplay between long-term BPV, MetS, and DM risk.
Another important finding of this study is that the magnitude of the effect of BPV on DM risk was stronger in women than men, regardless of SBPV and DBPV. Similar results were found in a previous observational study (14), which suggested that the associations of 24-h BPV with prediabetes and DM were stronger in women for both day and night. Evidence has shown that BP regulation differs significantly between men and women (47, 48). The autonomic nervous system and its sympathetic arm play important roles in the regulation of BP, and whole-body sympathetic neural activity increases at a greater rate with age in women than in men (49). In addition, the ability to buffer sympathetically mediated vasoconstriction through beta-adrenergic-mediated vasodilation also decreases in women with age (50, 51). Therefore, women have more sympathetic activity than men, resulting in a larger variation in BP. Further research is warranted to examine the sex-specific association between BPV and adverse outcomes.
Compared with the ARV, the SD and CV showed less significance regarding the association of BPV with the risk of DM. Although all three of the above indices are commonly used to assess BPV, only the SD index reflects the dispersion of values around the mean and has been known to be influenced by the average BP level even after adjustment for the average BP level, and neither the SD nor CV account for the order in which BP measurements are obtained (52, 53). Hence, the association between BPV and DM risk might be underestimated when using the SD or CV. In contrast, since the ARV is the average absolute difference between successive BP measurements, which could take into account the sequential order of BP changes while quantifying variability between adjacent readings, it is a more appropriate index of BPV for predicting the risk of DM (54).
Our study adds new evidence that in addition to various adverse outcomes (e.g., cardiovascular events, neurocognitive impairment) (55), BPV measured across visits might be a strong predictor of diabetes, even to a larger extent than average BP values. Given that the use of BPV based on home blood pressure monitoring or ‘in-clinic’ measurements taken over months or years has become increasingly feasible with the proliferation of electronic health records (56), the clinical implication of our study lies in the fact that relatively easily accessible visit-to-visit BPV could improve risk factor stratification for DM, and stabilisation of long-term BP could be considered a potentially important target in reducing risk of DM. BP-lowering therapy, such as calcium-channel blockers or thiazide diuretics (57), may offer benefits in reducing DM risk in high BPV groups.
There were several notable limitations to this study. First, the study was unable to determine causality in the findings because of the observational design. To minimize the possible effects of reverse causality, subjects with preexisting diabetes were excluded, and all subjects with outcomes occurring after the first 3 years of follow-up. Second, the majority of the participants from the original CHNS were not included in this analysis (62%), which might reduce problem of selection bias. Since excluded subjects were more likely to have a history of antihypertensive treatment and a lower educational level (Supplementary Table S3), the association between long-term BPV and risk of DM might have been diluted. Third, although adjustments were made for a wide array of covariates in the Cox regression models, data for several potential confounders (e.g., dietary habits and family history of diabetes) were not available, which might result in some residual confounding. Fourth, BP measurements from each participant across follow-up visits during the entire study duration were not systematically obtained at the same time of day or measured by the same examiner, which could contribute to the random error of BPV and residual confounding, and the associations might be attenuated. Fifth, the diagnosis of DM was mainly based on self-report with only blood samples used in the 2009 survey, leading to relatively higher incident DM cases in the 2009 survey than in other surveys; thus, the Cox model results for time-event estimation may be bias. However, we deemed that this criticism could be assuaged to some extent, given that the primary results remained largely unchanged after performing sensitivity analyses that DM was identified only by self-reports of diabetes diagnosis and/or receiving treatment for diabetes (Supplementary Table S9).
In conclusion, our study adds new evidence that greater long-term variability in both SBP and DBP as assessed by the ARV is independently associated with a significantly increased risk of incident DM after adjusting for baseline characteristics and the cumulative mean BP, and it was augmented when both were present together. Further research is warranted to examine whether interventions for reducing BPV could translate into clinical benefits for the prevention of DM.
Ethics Statement
The studies involving human participants were reviewed and approved by the institutional review committees of the Chinese Center for Disease Control and Prevention. The patients/participants provided their written informed consent to participate in this study.
Author contributions
RZ: substantial contributions to the design, analysis and interpretation of data for the work, and wrote manuscript. F-RL: substantial contributions to the design, acquisition of the data for the work, revised work critically for important intellectual content and discussion. KL, R-DH, H-ML, Z-LY, and J-ZZ: substantial contributions to revise work critically for important intellectual content and discussion. M-CZ: substantial contributions to the design of data for the work, revised work critically for important intellectual content and discussion, and final approval of manuscript version for publication. X-BW: substantial contributions to the acquisition of data for the work, revised work critically for important intellectual content and discussion, and final approval of manuscript version for publication.
Funding
This work was supported by the National Natural Science Foundation of China (82173607), Guangdong Basic and Applied Basic Research Foundation (2021A1515011684), Open Project of the Guangdong Provincial Key Laboratory of Tropical Disease Research (2020B1212060042), and Guangzhou Science and Technology Project (202102080597). The sponsors played no role in the study design or implementation; data collection, management, analysis, and interpretation; manuscript preparation, review, or approval; or the decision to submit the manuscript for publication.
Conflict of Interest
The authors declare that they do not have any conflicts of interest.
Supplementary Material
The Supplementary Material for this article can be found online at: https://www.ssph-journal.org/articles/10.3389/ijph.2023.1605445/full#supplementary-material
References
1. Harding, J, Pavkov, M, Magliano, D, Shaw, J, and Gregg, E. Global Trends in Diabetes Complications: a Review of Current Evidence. Diabetologia (2018) 62:3–16. doi:10.1007/s00125-018-4711-2
2. Zheng, Y, Ley, S, and Hu, F. Global Aetiology and Epidemiology of Type 2 Diabetes Mellitus and its Complications. Nat Rev Endocrinol (2017) 14:88–98. doi:10.1038/nrendo.2017.151
3. Cho, NH, Shaw, J, Karuranga, S, Huang, Y, da Rocha Fernandes, JD, Ohlrogge, A, et al. IDF Diabetes Atlas: Global Estimates of Diabetes Prevalence for 2017 and Projections for 2045. Diabetes Res Clin Pract (2018) 138:271–81. doi:10.1016/j.diabres.2018.02.023
4. Li, Y, Teng, D, Shi, X, Qin, G, Qin, Y, Quan, H, et al. Prevalence of Diabetes Recorded in mainland China Using 2018 Diagnostic Criteria from the American Diabetes Association: National Cross Sectional Study. BMJ (2020) 369:m997. doi:10.1136/bmj.m997
5. Kim, M-J, Lim, N-K, Choi, S-J, and Park, H-Y. Hypertension Is an Independent Risk Factor for Type 2 Diabetes: The Korean Genome and Epidemiology Study. Hypertens Res (2015) 38:783–9. doi:10.1038/hr.2015.72
6. Jung, J, Oh, C-M, Ryoo, J-H, Choi, J-M, Choi, Y-J, Ham, W, et al. The Influence of Prehypertension, Hypertension, and Glycated Hemoglobin on the Development of Type 2 Diabetes Mellitus in Prediabetes: the Korean Genome and Epidemiology Study (KoGES). Endocrine (2018) 59:593–601. doi:10.1007/s12020-018-1530-7
7. Parati, G, Ochoa, J, Salvi, P, Lombardi, C, and Bilo, G. Prognostic Value of Blood Pressure Variability and Average Blood Pressure Levels in Patients with Hypertension and Diabetes. Diabetes Care (2013) 36:S312–24. doi:10.2337/dcS13-2043
8. Muntner, P, Joyce, C, Levitan, E, Holt, E, Shimbo, D, Webber, L, et al. Reproducibility of Visit-To-Visit Variability of Blood Pressure Measured as Part of Routine Clinical Care. J Hypertens (2011) 29:2332–8. doi:10.1097/HJH.0b013e32834cf213
9. Pickering, T, Appel, L, Falkner, B, Jones, D, Kurtz, T, Sheps, S, et al. Recommendations for Blood Pressure Measurement in Humans and Experimental Animals. Hypertension (2005) 45:299–310. doi:10.1161/01.hyp.0000150857.39919.cb
10. Chiriacò, M, Pateras, K, Virdis, A, Charakida, M, Kyriakopoulou, D, Nannipieri, M, et al. Association between Blood Pressure Variability, Cardiovascular Disease and Mortality in Type 2 Diabetes: A Systematic Review and Meta-Analysis. Diabetes Obes Metab (2019) 21(12):2587–98. doi:10.1111/dom.13828
11. Gosmanova, E, Mikkelsen, M, Molnar, M, Lu, JL, Yessayan, L, Kalantar-Zadeh, K, et al. Association of Systolic Blood Pressure Variability with Mortality, Coronary Heart Disease, Stroke, and Renal Disease. J Am Coll Cardiol (2016) 68:1375–86. doi:10.1016/j.jacc.2016.06.054
12. Angelis, K, Senador, D, Mostarda, C, Irigoyen, M, and Morris, M. Sympathetic Overactivity Precedes Metabolic Dysfunction in a Fructose Model of Glucose Intolerance in Mice. Am J Physiol Regul Integr Comp Physiol (2012) 302:R950–7. doi:10.1152/ajpregu.00450.2011
13. Okada, R, Yasuda, Y, Tsushita, K, Wakai, K, Hamajima, N, and Matsuo, S. Within-visit Blood Pressure Variability Is Associated with Prediabetes and Diabetes. Sci Rep (2015) 5:7964. doi:10.1038/srep07964
14. Zhou, T, Kroon, A, Reesink, K, Schram, M, Koster, A, Schaper, N, et al. Blood Pressure Variability in Individuals with and without (Pre)diabetes: The Maastricht Study. J Hypertens (2017) 36:259–67. doi:10.1097/HJH.0000000000001543
15. Marteau, J-B, Zaiou, M, Siest, G, and Visvikis-Siest, S. Genetic Determinants of Blood Pressure Regulation. J Hypertens (2005) 23(12):2127–43. doi:10.1097/01.hjh.0000186024.12364.2e
16. Yano, Y, Fujimoto, S, Kramer, H, Sato, Y, Konta, T, Iseki, K, et al. Long-Term Blood Pressure Variability, New-Onset Diabetes Mellitus, and New-Onset Chronic Kidney Disease in the Japanese General Population. Hypertension (2015) 66:30–6. doi:10.1161/HYPERTENSIONAHA.115.05472
17. Popkin, B, Du, S, Zhai, F, and Zhang, B. Cohort Profile: The China Health and Nutrition Survey – Monitoring and Understanding Socio-Economic and Health Change in China, 1989-2011. Int J Epidemiol (2009) 39:1435–40. doi:10.1093/ije/dyp322
18. Du, S, Neiman, A, Batis, C, Wang, H, Zhang, B, Zhang, J, et al. Understanding the Patterns and Trends of Sodium Intake, Potassium Intake, and Sodium to Potassium Ratio and Their Effect on Hypertension in China. Am J Clin Nutr (2013) 99:334–43. doi:10.3945/ajcn.113.059121
19. Yoo, J, Shin, D, Kyungdo, H, Kim, D, Lee, S-P, Jeong, S-M, et al. Blood Pressure Variability and the Risk of Dementia: A Nationwide Cohort Study. Hypertens (2020) 75:982–90. doi:10.1161/HYPERTENSIONAHA.119.14033
20. Lee, S-R, Choi, Y-J, Choi, E-K, Han, S, Lee, E, Cha, M-J, et al. Blood Pressure Variability and Incidence of New-Onset Atrial Fibrillation: A Nationwide Population-Based Study. Hypertension (2019) 75:309–15. doi:10.1161/HYPERTENSIONAHA.119.13708
21. Levitan, EB, Kaciroti, N, Oparil, S, Julius, S, and Muntner, P. Relationships between Metrics of Visit-To-Visit Variability of Blood Pressure. J Hum Hypertens (2013) 27(10):589–93. doi:10.1038/jhh.2013.19
22. Yano, Y. Visit-to-Visit Blood Pressure Variability—What Is the Current challenge? Am J Hypertens (2016) 30:112–4. doi:10.1093/ajh/hpw124
23. Muntner, P, Whittle, J, Lynch, A, Colantonio, L, Simpson, L, Einhorn, P, et al. Visit-to-Visit Variability of Blood Pressure and Coronary Heart Disease, Stroke, Heart Failure, and Mortality: A Cohort Study. Ann Intern Med (2015) 163:329–38. doi:10.7326/M14-2803
24. Han, T, Zhang, S, Duan, W, Ren, X, Wei, C, Sun, C, et al. Eighteen-year Alcohol Consumption Trajectories and Their Association with Risk of Type 2 Diabetes and its Related Factors: the China Health and Nutrition Survey. Diabetologia (2019) 62:970–80. doi:10.1007/s00125-019-4851-z
25. Gribble, M, Howard, B, Umans, J, Shara, N, Francesconi, K, Goessler, W, et al. Arsenic Exposure, Diabetes Prevalence, and Diabetes Control in the Strong Heart Study. Am J Epidemiol (2012) 176:865–74. doi:10.1093/aje/kws153
26. Kautzky-Willer, A, Harreiter, J, and Pacini, G. Sex and Gender Differences in Risk, Pathophysiology and Complications of Type 2 Diabetes Mellitus. Endocr Rev (2016) 37(3):278–316. doi:10.1210/er.2015-1137
27. Zhang, J, Chen, Z, Pärna, K, van Zon, SKR, Snieder, H, and Thio, CHL. Mediators of the Association between Educational Attainment and Type 2 Diabetes Mellitus: a Two-step Multivariable Mendelian Randomisation Study. Diabetologia (2022) 65(8):1364–74. doi:10.1007/s00125-022-05705-6
28. Zheng, Y, Ley, SH, and Hu, FB. Global Aetiology and Epidemiology of Type 2 Diabetes Mellitus and its Complications. Nat Rev Endocrinol (2018) 14(2):88–98. doi:10.1038/nrendo.2017.151
29. Ng, SW, Howard, A-G, Wang, HJ, So, C, and Zhang, B. The Physical Activity Transition Among Adults in China: 1991–2011. Obes Rev (2014) 15:27–36. doi:10.1111/obr.12127
30. He, J, Fang, A, Yu, S, Shen, X, and Li, K. Dietary Nonheme, Heme, and Total Iron Intake and the Risk of Diabetes in Adults: Results from the China Health and Nutrition Survey. Diabetes Care (2020) 43:776–84. doi:10.2337/dc19-2202
31. Liu, L-S, Wu, Z-S, Wang, J-G, Wang, W, Bao, Y-J, Cai, J, et al. Chinese Guidelines for Prevention and Treatment of Hypertension-A Report of the Revision Committee of Chinese Guidelines for Prevention and Treatment of Hypertension. J Geriatr Cardiol (2019) 16:182–245.
32. Joyner, MJ, Charkoudian, N, and Wallin, BG. Sympathetic Nervous System and Blood Pressure in Humans: Individualized Patterns of Regulation and Their Implications. Hypertens (2010) 56(1):10–6. Available from: https://pubmed.ncbi.nlm.nih.gov/20497993. doi:10.1161/HYPERTENSIONAHA.109.140186
33. Li, Z, Snieder, H, Su, S, Harshfield, G, Treiber, F, and Wang, X. A Longitudinal Study of Blood Pressure Variability in African-American and European American Youth. J Hypertens (2010) 28:715–22. doi:10.1097/HJH.0b013e328336ed5b
34. Tsuchihashi, T, Abe, I, Tsukashima, A, Kobayashi, K, and Fujishima, M. Effects of Meals and Physical Activity on Blood Pressure Variability in Elderly Patients. A Preliminary Study. Am J Hypertens (1991) 3:943–6. doi:10.1093/ajh/3.12.943
35. Ohta, Y, Kawano, Y, Hayashi, S, Iwashima, Y, Yoshihara, F, and Nakamura, S. Effects of Cigarette Smoking on Ambulatory Blood Pressure, Heart Rate, and Heart Rate Variability in Treated Hypertensive Patients. Clin Exp Hypertens (2016) 38:510–3. doi:10.3109/10641963.2016.1148161
36. Kallianos, A, Trakada, G, Papaioannou, T, Nikolopouloss, I, Mitrakou, A, Manios, E, et al. Glucose and Arterial Blood Pressure Variability in Obstructive Sleep Apnea Syndrome. Eur Rev Med Pharmacol Sci (2013) 17:1932–7.
37. Yano, Y, Ning, H, Allen, N, Reis, J, Launer, L, Liu, K, et al. Long-Term Blood Pressure Variability throughout Young Adulthood and Cognitive Function in Midlife the Coronary Artery Risk Development in Young Adults (CARDIA) Study. Hypertension (2014) 64:983–8. doi:10.1161/HYPERTENSIONAHA.114.03978
38. Faramawi, M, Delongchamp, R, Said, Q, Jadhav, S, and Abouelenien, S. Metabolic Syndrome Is Associated with Visit-To-Visit Systolic Blood Pressure Variability in the US Adults. Hypertens Res (2014) 37:875–9. doi:10.1038/hr.2014.89
39. Diaz, K, Veerabhadrappa, P, Kashem, M, Thakkar, S, Feairheller, D, Sturgeon, K, et al. Visit-to-visit and 24-h Blood Pressure Variability: Association with Endothelial and Smooth Muscle Function in African Americans. J Hum Hypertens (2013) 27:671–7. doi:10.1038/jhh.2013.33
40. Michell, A. Prognostic Significance of Blood-Pressure Variability. Lancet (2010) 376:414–5. doi:10.1016/S0140-6736(10)61213-5
41. Koumaras, C, Katsiki, N, Athyros, V, and Karagiannis, A. Metabolic Syndrome and Arterial Stiffness: The Past, the Present and the Future. J Cardiovasc Med (Hagerstown) (2013) 14:687–9. doi:10.2459/JCM.0b013e3283657c96
42. Soler, A, Hunter, I, Joseph, G, Hutcheson, R, Hutcheson, B, Yang, J, et al. Elevated 20-HETE in Metabolic Syndrome Regulates Arterial Stiffness and Systolic Hypertension via MMP12 Activation. J Mol Cel Cardiol (2018) 117:88–99. doi:10.1016/j.yjmcc.2018.02.005
43. Won, K-B, Park, G-M, Lee, S-E, Cho, I-J, Lee, B, Chang, H-J, et al. Relationship of Insulin Resistance Estimated by Triglyceride Glucose index to Arterial Stiffness. Lipids Health Dis (2018) 17:268. doi:10.1186/s12944-018-0914-2
44. Kim, O, Lim, HH, Lee, MJ, Kim, JY, and Lee, J-C. Association of Fatty Acid Composition in Serum Phospholipids with Metabolic Syndrome and Arterial Stiffness. Nutr Metab Cardiovasc Dis (2011) 23:366–74. doi:10.1016/j.numecd.2011.06.006
45. Lin, YK, Liu, PY, Fan, CH, Tsai, KZ, Lin, YP, Lee, JM, et al. Metabolic Biomarkers and Long-Term Blood Pressure Variability in Military Young Male Adults. World J Clin Cases (2020) 8:2246–54. doi:10.12998/wjcc.v8.i11.2246,
46. Lee, HT, Namgung, J, Lim, Y-H, Park, H-C, Park, J, and Shin, J. The Relationship between Visit-To-Visit Variability in Blood Pressure and Incidence of Metabolic Syndrome: a General Population-Based Cohort Study in Korea. Clin Hypertens (2019) 25:11. doi:10.1186/s40885-019-0117-9
47. Reckelhoff, JF. Gender Differences in the Regulation of Blood Pressure. Hypertension (2001) 37:1199–208. doi:10.1161/01.hyp.37.5.1199
48. Baker, S, Limberg, J, Dillon, G, Curry, T, Joyner, M, and Nicholson, W. Aging Alters the Relative Contributions of the Sympathetic and Parasympathetic Nervous System to Blood Pressure Control in Women. Hypertens (2018) 72:1236–42. doi:10.1161/HYPERTENSIONAHA.118.11550
49. Matsukawa, T, Sugiyama, Y, Watanabe, T, Kobayashi, F, and Mano, T. Gender Difference in Age-Related Changes in Muscle Sympathetic Nerve Activity in Healthy Subjects. Am J Physiol (1998) 275:R1600–4. doi:10.1152/ajpregu.1998.275.5.R1600
50. Hart, E, Charkoudian, N, Wallin, G, Curry, T, Eisenach, J, and Joyner, M. Sex and Ageing Differences in Resting Arterial Pressure Regulation: The Role of the β-adrenergic Receptors. J Physiol (2011) 589:5285–97. doi:10.1113/jphysiol.2011.212753
51. Best, S, Okada, Y, Galbreath, M, Jarvis, S, Bivens, T, Adams-Huet, B, et al. Age and Sex Differences in Muscle Sympathetic Nerve Activity in Relation to Haemodynamics, Blood Volume and Left Ventricular Size. Exp Physiol (2014) 99:839–48. doi:10.1113/expphysiol.2013.077248
52. Mena, L, Pintos, S, Queipo, N, Aizpúrua, J, Maestre, G, and Sulbarán, T. A Reliable index for the Prognostic Significance of Blood Pressure Variability. J Hypertens (2005) 23:505–11. doi:10.1097/01.hjh.0000160205.81652.5a
53. Asayama, K, Kikuya, M, Schutte, R, Thijs, L, Hosaka, M, Michihiro, S, et al. Home Blood Pressure Variability as Cardiovascular Risk Factor in the Population of Ohasama. Hypertension (2012) 61:61–9. doi:10.1161/HYPERTENSIONAHA.111.00138
54. Parati, G, Ochoa, J, Lombardi, C, and Bilo, G. Assessment and Management of Blood-Pressure Variability. Nat Rev Cardiol (2013) 10:143–55. doi:10.1038/nrcardio.2013.1
55. Bencivenga, L, De Souto Barreto, P, Rolland, Y, Hanon, O, Vidal, J-S, Cestac, P, et al. Blood Pressure Variability: A Potential Marker of Aging. Ageing Res Rev (2022) 80:101677. doi:10.1016/j.arr.2022.101677
56. Parati, G, Ochoa, JE, Lombardi, C, and Bilo, G. Blood Pressure Variability: Assessment, Predictive Value, and Potential as a Therapeutic Target. Curr Hypertens Rep (2015) 17(4):537. doi:10.1007/s11906-015-0537-1
Keywords: China, diabetes mellitus, blood pressure, variability, cohort
Citation: Zhou R, Li F-R, Liu K, Huang R-D, Liu H-M, Yuan Z-L, Zheng J-Z, Zou M-C and Wu X-B (2023) Long-Term Visit-To-Visit Blood Pressure Variability and Risk of Diabetes Mellitus in Chinese Population: A Retrospective Population-Based Study. Int J Public Health 68:1605445. doi: 10.3389/ijph.2023.1605445
Received: 01 October 2022; Accepted: 25 January 2023;
Published: 06 February 2023.
Edited by:
Licia Iacoviello, Mediterranean Neurological Institute Neuromed (IRCCS), ItalyReviewed by:
Ana Lídia Rouxinol-Dias, University of Porto, PortugalCopyright © 2023 Zhou, Li, Liu, Huang, Liu, Yuan, Zheng, Zou and Wu. This is an open-access article distributed under the terms of the Creative Commons Attribution License (CC BY). The use, distribution or reproduction in other forums is permitted, provided the original author(s) and the copyright owner(s) are credited and that the original publication in this journal is cited, in accordance with accepted academic practice. No use, distribution or reproduction is permitted which does not comply with these terms.
*Correspondence: Meng-Chen Zou, zoumc163@163.com; Xian-Bo Wu, wuxb1010@smu.edu.cn
†These authors have contributed equally to this work and share first authorship