- 1School of Management Engineering and E-Commerce, Zhejiang Gongshang University, Hangzhou, China
- 2School of Business Administration, Zhejiang Gongshang University, Hangzhou, China
Objective: To explore the law of opinion dissemination and individual opinion evolution at the micro level, this paper analyzes the influence of variation and oyster on communication from the perspective of network structure.
Methods: In this paper, we introduce the concepts of “variation” and “oyster”, build a multi-layer coupled network environment combined with the ISOVR model, and conduct simulation experiments of network information dissemination based on the bounded trust model.
Results: The experimental results reveal that the extent and scope of variation’s spread in the network are more dependent on the trust of nodes themselves, and decreasing the trust of nodes significantly reduces the rate and peak value of variation. Changing the silence coefficient of variation does not effectively change the direction of rumor propagation, which indicates that rumor has a strong propagation ability after mutation.
Conclusion: The insights of this paper on the dissemination of public opinions include: 1) pay attention to people with high trust levels, such as opinion leaders; 2) clarify the misinformation in time to prevent further spread of rumors.
Introduction
With the rapid development of mobile networks and various media, the speed and scope of information dissemination have been greatly improved. When some unexpected events occur, all kinds of randomly fabricated and maliciously distorted news are widely spread [1]. New requirements have been put forward on how to effectively guide and control public opinions by governments and other public network opinion organizations. This paper analyses the characteristics of rumor spreading, extending its background to the coupled network, including layers of the online Internet, such as the intermediate transition layer and offline entity layer, and proposes a multi-layer coupled network based on information diffusion and bounded trust. The purpose is to obtain the rules of rumor spreading through different topological structures, to help the government control rumor spreading, and guide public opinions.
In terms of news dissemination, social media has increased the likelihood of one’s exposure to news events [2], also making the News Find Me effect more pronounced. However, the News Find Me effect causes people to receive information in a way that favors passive reception [3] and has no positive effect on the proper understanding of information [4]. Research on rumor spreading based on the infectious disease model began in the 1970s [5–10]. With further research, researchers have verified the fact that a larger trust parameter, forgetting rate, and time delay affect the speed of rumor spreading [11–13]. Du et al. [14] concluded that public education and timely correction of media errors were crucial factors of propagation. From the perspective of the heterogeneity of public risk perception, the lower the heterogeneity of public risk perception, the greater the impact on public opinions [15].
Researchers have used the viewpoint dynamics model to describe rumor propagation and changes in perspectives. Zha et al. [16] constructed a consensus-reaching model with a personalized feedback mechanism to propose acceptable opinions when the bounded confidence level is either known or unknown. Xiao et al. [17] verified that changes in individual opinions were not only influenced by interactions between adjacent nodes, but also by the natural renewal of individuals. Based on previous studies, Vasca et al. [18] constructed a bounded trust HK model with time heterogeneity to describe changes in group perspectives. Zhu et al. [19] divided people into opinion leaders and followers, and found that opinion leaders played a vital role in opinion convergence and initial opinion value. Then they added a trust incentive mechanism for uncorrected information and false information [20] and finally found that it was the copycat phenomenon that contributed to false advertising activities. Ju et al. [21] studied social relationship prediction by integrating personality traits and asymmetric interactions in a social network. The simulation experiment found that the discrete behavior would attract agents who trusted it and made them express extreme views.
More and more researchers have introduced the double-layer or multi-layer coupled network rumor spreading model. Zhang et al. [22] divided nodes into high influence layer and low influence layer, and designed two strategies of information closure and information elimination in rumor spreading. Barnard et al. [23] analyzed the evolution of infectious diseases by combining the association between individuals in the double static-dynamic layer network with time, type, and structural heterogeneity. Wang et al. [24] analyzed the impact of the social reinforcement effect, users’ perceived value, and other factors on rumor spreading from the perspective of user asymmetry in the network environment. Liu et al. [25] analyzed the local stability of the disease-free equilibrium point of the system according to the transformation law between different states in the model. Chen [26] constructed a multi-layer (WeChat layer-Weibo layer-control layer) coupled network model of public opinion communication control. Through simulation experiments, Zhu et al. [27] found that knowledge spreads fastest in the scale-free homogeneous hybrid double-layer coupled network. The communication frequency between online and offline knowledge was relatively close, the spread range was larger and the spread speed was faster.
In recent years, with deepening research on rumor propagation, scholars have analyzed the rules of rumor propagation from different perspectives [28–30], resulting in categories for the types and nature of rumor propagation, dissemination groups, network structure, and the polarization degree of individual opinions, etc. However, few studies have examined the mechanism of rumor spreading online and offline. Thus, this paper constructs a three-layer coupled network. The main contributions of this paper are as follows.
(1) An improved multi-layer coupled network structure is proposed to analyze the interaction of nodes both online and offline.
(2) The phenomena of “variation” and “oyster” are introduced and innovatively described from the perspective of viewpoint interaction.
(3) Experiments on multi-layer coupled network communication incorporating information diffusion and bounded trust are carried out, and the evolution of public opinion dissemination is analyzed from both macro and micro perspectives.
Methods
The main work of this paper is as follows: Firstly, referring to Ju et al. [31], we propose an ISVOR model with concepts of “variation” and “oyster”. According to the model, we divide people into three groups: I (Ignorant), S (Spreader), and V (Variation). Secondly, considering the situation of online and offline interactions in the social network era, a multi-layer coupled network of online Internet layer, intermediate transition layer, and offline entity layer is conducted. Thirdly, based on the model of online rumor spreading with variation and oyster phenomenon, information diffusion and bounded trust are introduced to explore distorted dissemination of information and the short period of stopping dissemination in the life cycle of a rumor. Figure 1 is a three-layer coupled network with random online and offline coupling.
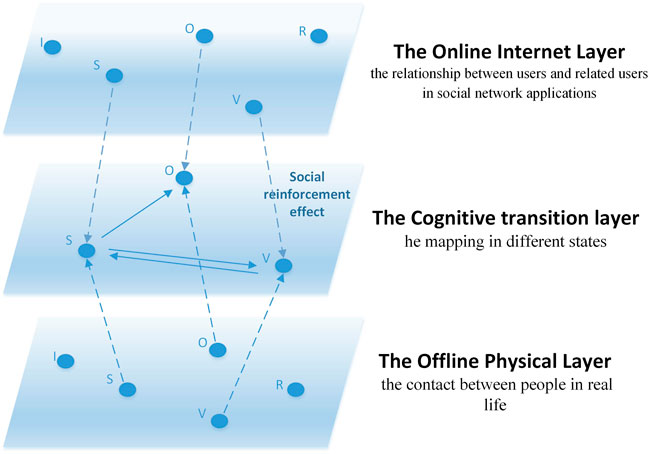
FIGURE 1. Three-layer coupled network with random online and offline coupling (Public opinion research, China, 2021-2022).
For the online Internet layer and offline entity layer, the improved Amend-Linear Threshold (A-LT) model is used to represent the diffusion and elimination of rumors, and the J-A bounded trust model is used to describe the interactions between individuals. The intermediate transition layer of the three-layer coupled network in this paper adopts the random synchronization of the inter-layer state with the social reinforcement effect. The structure of the three-layer coupled network will be described in detail in Three-Layer Coupled Network Structure section.
Characteristics of Individual Influence
The heterogeneity attribute of the node itself can promote or inhibit the spread of rumors [32]. This paper mainly considers the interaction between nodes and adjacent nodes. We use Degree Centrality and Eigen Centrality to describe the influence of structural dimensions of nodes in the propagation. And we set
1) Degree Centrality. The greater the degree of a node is, the greater the influence of this point will be. We use the following equation [33] to describe the degree of centrality of node
2) Eigen Centrality. And the influence of nodes is distributed to all nodes in the network so that nodes with high centrality contribute more [34]. We use
where
where x is the eigenvector corresponding to the eigen values of matrix
3) Assuming that
This paper uses the structural dimension influence and attribute influence to determine the individual influence of node i in Internet Topology, denoted as
In the online Internet layer, the probability of the node
Activation and Extinction of Rumor Diffusion
In the improved linear threshold (A-LT) model, the nodes in the network are divided into three categories: ignorant nodes (I) that are not exposed to rumors or infected by rumors, carriers (C) that are infected with rumors and have the ability to spread rumors, and recoveries (R) that no longer spread rumors. This paper assumes that when the node energy received by the unknown node reaches the wake-up threshold, the node will be activated and become a carrier and spread the rumor to neighboring nodes. At the same time, when the energy of adjacent nodes of the activated carrier is lower than a certain value, the impact of rumors begins to disappear and the node state changes into an immune state. The value here is defined as the Extinguishment threshold.
In the micro process of diffusion, rumor carriers can be subdivided into the spreader (S), variation (V), and the oyster (O), and the three groups of individuals have different influences. After a period of silence, some oysters will become immune to the rumor through rational thinking, and some of them will turn to the state of “spreading rumor”. They ignore the variation of rumors in the propagation and the influence of unstable factors of variation on the transmission chain. The state changes of users in the online Internet layer and offline entity layer are shown in Figure 2.
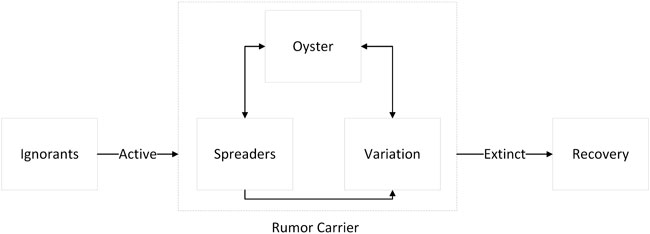
FIGURE 2. Activation and extinction process of rumor diffusion (Public opinion research, China, 2021-2022).
The total number of users in each layer is set as
In this model, the arousal threshold
where
This model uses the blanking threshold
We assume that the extinguishing threshold
We use
Variation and Oyster in Rumor Spreading
There will be the phenomenon of silence and variation in group communication. We call these people oysters and variations. Different from ordinary rumor spreaders, the content spread by Variations has changed significantly with the change in how news and specific information are disseminated, and there is a tendency for constant change. Oysters receive rumors but do not take the initiative to spread rumors. They are either in a thinking state or adopt a wait-and-see attitude.
We assume that the initial value
Referring to the JA model of Jager et al. [35], the rules of opinion update are as follows: when the difference between two opinion values is within the allowed interval
where the
When the difference of opinion values is out of the interval
When the opinion values of other intervals are different, there is no willingness to communicate between nodes, so the opinion values of nodes will not change. Meanwhile, we stipulate that once the opinion value interval of nodes is in
Three-Layer Coupled Network Structure
In the intermediate cognitive transition layer, the social reinforcement effect is redefined as [36]: nodes in the network layer are influenced by multiple neighboring nodes. The probability of random synchronization from the intermediate cognitive transition layer to the offline entity layer in the online Internet layer [37] is:
where
The connection mode of nodes at different layers adopts the combination of the following three modes [38, 39]: 1) assortative links between layers; 2) disassortative links between layers, which is the opposite of assortative links between layers; and 3) random links between layers.
For the topological structure of social networks, the activation and elimination of rumor diffusion, and allowing for the variations and oysters in the process, this paper makes the following provisions: 1) the topology of all networks is static; 2) there is no after-effects in the online internet layer and the offline entity layer; 3) the node relationship between the online internet layer and the offline entity layer is bidirectional; and 4) the nodes between the online Internet layer and the offline entity layer are one-on-one. Figure 3 is the experimental flow chart of this paper.
Result
In this section, we use the Monte Carlo method to simulate on the Python platform. In order to verify the scientificity and reliability of the above model, this paper selected the “Liu Xuezhou Incident”, which was fermented in the campus forum as a case study, verified the science of the three-layer coupled network communication model based on case data, and conducted simulation experiments.
Brief description of the incident: “Liu Xuezhou Incident” was a public opinion event that occurred at the end of 2021. The origin of the incident is as follows: On 6 December 2021, Liu Xuezhou posted a video of a family search on the Internet, which aroused the attention of the general public. On 16 December, the elderly members of the family found Liu Xuezhou’s biological parents through the vaccine book of that year, and the two sides recognized each other through DNA comparison. However, on 17 January 2022, Liu Xuezhou posted an article on a social media platform claiming that they were abandoned by their biological parents. The dispute started because they had hoped that their biological parents could provide a place for them to live. The media also published one-sided and suggestive headline articles to mislead netizens. In the early morning of 24 January, Liu Xuezhou committed suicide after posting a long article. This paper used the content of all users in the #LiuXueZhou incident topic from 6 December 2021, to 31 January 2022, which resulted in 2078 user nodes after filtering out irrelevant data. We defined user interactions in terms of re-Tweets (on the social media platform Twitter) and comments on published content. We finally achieved the following user interaction network diagram based on the interaction between users, shown in Figure 4A. Meanwhile, based on the user data we trawled, the trend graph of the population was obtained through the calculation of the above model, as shown in Figure 4B.
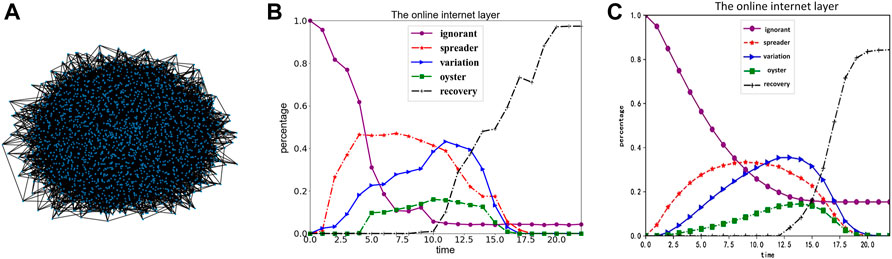
FIGURE 4. Comparison of real data and simulation data (Public opinion research, China, 2021-2022). (A) shows the user interaction network diagram presented according to the actual campus forum user; (B) shows the trend graph of the population change obtained according to the proposed user data and model; (C) shows the population change trend graph obtained according to the parameters mentioned in the text.
According to the Figures 4A,B, this topic can be abstracted as a BA scale-free network, which we simplified to a scale-free network with
The order of the experiment is the change of the proportion of each character, followed by the change of the random social reinforcement effect. We then examined the influence of variation and oyster attributes, the change of trust degree of nodes, and the change and variation of communicator silence coefficient. The rule of rumor spreading is analyzed through the parameter changes of influencing factors. The experimental results are shown in Figures 5, 6:
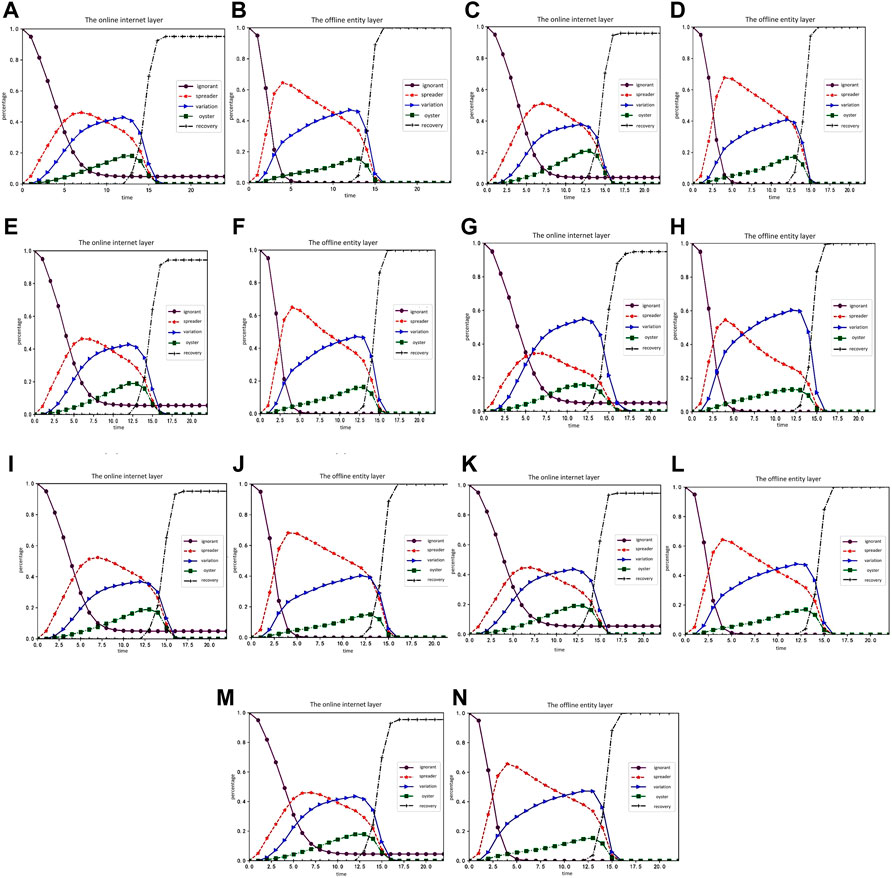
FIGURE 5. Simulation experiment result graph 1 (Public opinion research, China, 2021-2022). (A,B) show the time-varying curves of the proportion of the ignorant, communicator, mutants, oysters, and the immunity in the online internet layer and offline physical layer. (C–H) shows changes in the proportion of each character in the online internet layer and offline entity layer over time when the attribute influences of the mutant ArV are 1.5, 2.0, and 2.5, respectively. (I–N) shows the change of the proportion of each character in the online internet layer and offline entity layer over time when the attribute influences of oysters ArO are 0.25, 0.5, and 0.75, respectively.
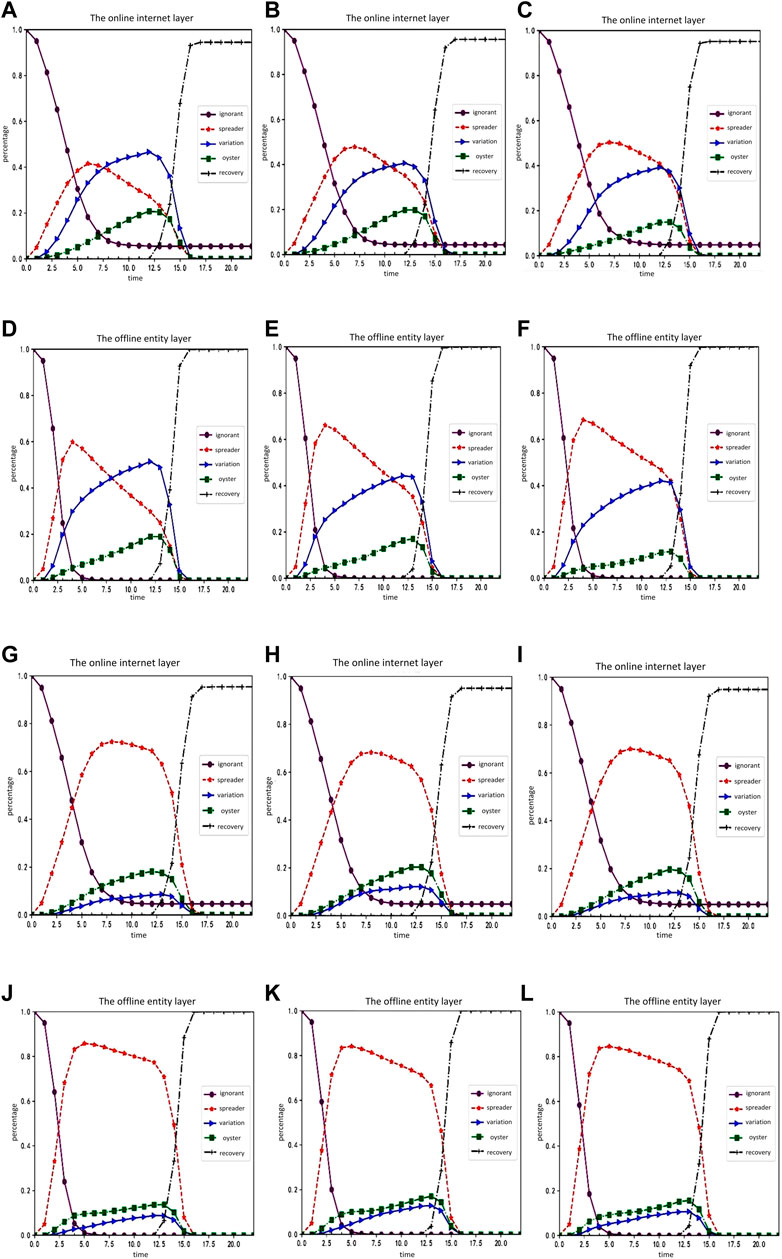
FIGURE 6. Simulation experiment result graph 2 (Public opinion research, China, 2021-2022). (A–F) shows changes in the proportion of each character in the online internet layer and offline entity layer over time when the communicator's silence coefficient ρ1 is −0.45, −0.4 and −0.35, respectively. (G–L) shows the changes of the proportion of each character in the online internet layer and offline entity layer over time when the variation’s silent coefficient ρ3 is 0.15, 0.2 and 0.25, respectively.
Figures 5A,B shows the time-varying curves of the proportion of the ignorant, communicator, mutants, oysters, and the immunity in the online internet layer and offline physical layer. Figures 5C–H shows changes in the proportion of each character in the online internet layer and offline entity layer over time when the attribute influences of the mutant
Figures 6A–F shows changes in the proportion of each character in the online internet layer and offline entity layer over time when the communicator’s silence coefficient
Based on a comprehensive comparative analysis, the following conclusion can be drawn: 1) each character in the offline physical layer changes in the same way as that in the online internet layer; 2) compared with the situation without random social reinforcement, the ignorant people in the offline physical layer are more likely to be infected by rumors and keep up with online events due to the superimposed influence of rumor carriers in the online internet layer; 3) when the attribute influence of mutants increases, the growth rate of silent mutants decreases, and the
Discussion
In this paper, we draw on an ISOVR model of Jiang et al. [31] and construct a multi-coupled network to analyze the online and offline spread of rumors. Meanwhile, we introduce the bounded trust model and social reinforcement effect for further study. The simulation results show that increasing the immunity rate and silence rate of the variation can effectively reduce the rate of rumor diffusion. The high random social reinforcement effect will promote the spread of rumors in the offline entity layer. The offline probability of nodes within a certain range will effectively control the spread of rumors in the online internet layer. In addition, the degree and scope of variation diffusion in the network depend more on the trust degree of the node itself. Increasing the trust degree of nodes can significantly promote the growth rate and peak value of the variation. Therefore, it is necessary to pay attention to the cultivation of influential groups that can play a positive role in the spread of rumors. Changing the silence coefficient of variation cannot control the rumor diffusion, which shows the powerful influence of rumor variation. Therefore, relevant departments should release true information in a timely way to eliminate rumors and prevent further variation and distortion of rumors.
Conclusion
This paper studies the variations and oysters in network communication and puts forward a new transmission mechanism and introduces the phenomenon of stagnation and information mutation in the network. The model has a wide range of application scenarios in the future, including personalized recommendation [40–43], data processing [44–46], sustainable tourism [47, 48], knowledge dissemination [49] and so on. At the same time, there are still some limitations, for example, although the parameters of this paper were obtained after the simulation of actual cases, it is not considered in the later analysis that the parameters will change dynamically according to the development of actual events [50]. As mentioned in this study, it is necessary to strengthen the cultivation of groups that have a positive impact on the spread of rumors. However, this paper does not cover how to identify and cultivate such groups, nor how to guide the dissemination and control of public opinion based on the feedback between groups [51]. In follow-up research, we will further analyze the influence of increasing intervention measures and inter-group feedback on the dissemination of false mutation information in the process of public opinion dissemination. We might also consider combining the optimal control model, taking the control cost of public opinion dissemination as the objective function to dynamically adjust the impact of the intervention strategy on information dissemination.
Author Contributions
All authors listed have made a substantial, direct, and intellectual contribution to the work and approved it for publication.
Funding
This research was funded by Zhejiang Provincial Key Project of Philosophy and Social Sciences (Grant No. 20NDJC10Z), Natural Science Foundation of Zhejiang Province (Nos LQ20G010002 and LY20G010001), Soft Science Research Program of Zhejiang Province (no. 2021C25010), the project of China (Hangzhou) Cross-border E-commerce College (No. 2021KXYJ06), the Philosophy and Social Science Foundation of Zhejiang Province (No. 21NDJC083YB), and Contemporary Business and Trade Research Center of Zhejiang Gongshang University (Nos XT202103 and XT202105).
Conflict of Interest
The authors declare that the research was conducted in the absence of any commercial or financial relationships that could be construed as a potential conflict of interest.
References
1. Liu, X. Brief Analyses on Allport's the Psychology of Rumor. Sci Soc Psychol (2014) 2014(Z1):44–8.
2. Park, CS, and Kaye, BK. What's This? Incidental Exposure to News on Social Media, News-Finds-Me Perception, News Efficacy, and News Consumption. Mass Commun Soc (2020) 23(2):157–80. doi:10.1080/15205436.2019.1702216
3. Gil de Zúñiga, H, Weeks, B, and Ardèvol-Abreu, A. Effects of the News-Finds-Me Perception in Communication: Social media Use Implications for News Seeking and Learning about Politics. J Comput-mediat Comm (2017) 22(3):105–23. doi:10.1111/jcc4.12185
4. Segado-Boj, F, Díaz-Campo, J, Navarro-Asencio, E, and Remacha-González, L. Influence of News-Finds-Me Perception on Accuracy, Factuality and Relevance Assessment. Case Study of News Item on Climate Change. Revista mediterránea de comunicación (2020) 11(2):85–103. doi:10.14198/medcom2020.11.2.12
5. Allport, GW, and Postman, L. An Analysis of Rumor. Public Opin Q (1946) 10(4):501–17. doi:10.1086/265813
6. Daley, DJ, and Kendall, DG. Stochastic Rumours. IMA J Appl Math (1965) 1(1):42–55. doi:10.1093/imamat/1.1.42
7. Sudbury, A. The Proportion of the Population Never Hearing a Rumour. J Appl Probab (1985) 22(2):443–6. doi:10.2307/3213787
8. Zanette, DH. Dynamics of Rumor Propagation on Small-World Networks. Phys Rev E Stat Nonlin Soft Matter Phys (2002) 65(4):041908. doi:10.1103/PhysRevE.65.041908
9. Moreno, Y, Nekovee, M, and Pacheco, AF. Dynamics of Rumor Spreading in Complex Networks. Phys Rev E Stat Nonlin Soft Matter Phys (2004) 69(6):066130. doi:10.1103/PhysRevE.69.066130
10. Gu, Y, and Xia, L. The Propagation and Inhibition of Rumor in Online Social Network. Chin J Phys (2012) 61(23):544–50. doi:10.7498/aps.61.238701
11. Wang, Y-Q, Yang, X-Y, Han, Y-L, and Wang, X-A. Rumor Spreading Model with Trust Mechanism in Complex Social Networks. Commun Theor Phys (2013) 59(4):510–6. doi:10.1088/0253-6102/59/4/21
12. Nekovee, M, Moreno, Y, Bianconi, G, and Marsili, M. Theory of Rumour Spreading in Complex Social Networks. Physica A: Stat Mech its Appl (2007) 374(1):457–70. doi:10.1016/j.physa.2006.07.017
13. Zhang, Y, and Chen, Z. SETQR Propagation Model for Social Networks. IEEE Access (2019) 7:127533–43. doi:10.1109/access.2019.2939150
14. Du, E, Chen, E, Liu, J, and Zheng, C. How Do Social media and Individual Behaviors Affect Epidemic Transmission and Control? Sci Total Environ (2021) 761:144114. doi:10.1016/j.scitotenv.2020.144114
15. Su, YY, Zhang, YM, He, X, and Du, CC. Research on Network Public Opinion Propagation Model of Emergency under Cross-Evolution of Risk Perception. Inf Sci (2020) 40(12):100–13.
16. Zha, Q, Dong, Y, Zhang, H, Chiclana, F, and Herrera-Viedma, E. A Personalized Feedback Mechanism Based on Bounded Confidence Learning to Support Consensus Reaching in Group Decision Making. IEEE Trans Syst Man, Cybernetics: Syst (2019) 51(6):3900–10. doi:10.1109/TSMC.2019.2945922
17. Xiao, R, Yu, T, and Hou, J. Modeling and Simulation of Opinion Natural Reversal Dynamics with Opinion Leader Based on HK Bounded Confidence Model. Complexity (2020) 2020:7360302. doi:10.1155/2020/7360302
18. Vasca, F, Bernardo, C, and Iervolino, R. Practical Consensus in Bounded Confidence Opinion Dynamics. Automatica (2021) 129:109683. doi:10.1016/j.automatica.2021.109683
19. Zhu, X, and Meng, J. The Evolution of Leaders and Followers’ Opinion in Multi-Layer Networks Based on Bounded Confidence Model. Inf Sci (2019) 37(06):30–6. doi:10.13833/j.issn.1007-7634.2019.06.005
20. Douven, I, and Hegselmann, R. Mis- and Disinformation in a Bounded Confidence Model. Artif Intelligence (2021) 291:103415. doi:10.1016/j.artint.2020.103415
21. Ju, C, Li, G, Bao, F, Gao, T, Zhu, Y, and Zhu, Y. Social Relationship Prediction Integrating Personality Traits and Asymmetric Interactions. Front Psychol (2022) 13:778722. doi:10.3389/fpsyg.2022.778722
22. Zhang, Y, and Pan, D. Layered SIRS Model of Information Spread in Complex Networks. Appl Mathematics Comput (2021) 411:126524. doi:10.1016/j.amc.2021.126524
23. Barnard, RC, Kiss, IZ, Berthouze, L, and Miller, JC. Edge-Based Compartmental Modelling of an SIR Epidemic on a Dual-Layer Static-Dynamic Multiplex Network with Tunable Clustering. Bull Math Biol (2018) 80(10):2698–733. doi:10.1007/s11538-018-0484-5
24. Wang, JK, Yu, H, Wang, XH, and Bai, L. Dissemination and Control Model of Public Opinion in Online Social Networks Based on Users’ Relative Weight. Syst Engineering-Theory Pract (2019) 39(6):1565–79.
25. Liu, N, Fang, J, Deng, W, and Liu, P. Stability Analysis for a Fractional-Order SIR Model Based on Two-Layer Coupled Complex Networks. Mathematics Pract Theor (2020) 50(20):117–23.
26. Shuai, C. Research on Dissemination and Control of Public Opinion Based on Multilayer Coupled Network. J Syst Simulation (2020) 32(12):2353.
27. Hongmiao, Z, Zhen, J, and Jiajin, Q. Research on Knowledge Spreading Dynamics on Online and Offline Double-Layer Coupled Network. Syst Engineering-Theory Pract (2020) 40(02):403–14.
28. Zhao, Y, Kou, G, Peng, Y, and Chen, Y. Understanding Influence Power of Opinion Leaders in E-Commerce Networks: An Opinion Dynamics Theory Perspective. Inf Sci (2018) 426:131–47. doi:10.1016/j.ins.2017.10.031
29. Li, R-D, Guo, Q, Ma, H-T, and Liu, J-G. Network Reconstruction of Social Networks Based on the Public Information. Chaos: Interdiscip J Nonlinear Sci (2021) 31(3):033123. doi:10.1063/5.0038816
30. Nguyen, VX, Xiao, G, Zhou, J, Li, G, and Li, B. Bias in Social Interactions and Emergence of Extremism in Complex Social Networks. Chaos: Interdiscip J Nonlinear Sci (2020) 30(10):103110. doi:10.1063/5.0009943
31. Ju, C, Jiang, Y, Bao, F, Zou, B, and Xu, C. Online Rumor Diffusion Model Based on Variation and Silence Phenomenon in the Context of COVID-19. Front Public Health (2021) 9:788475. doi:10.3389/fpubh.2021.788475
32. Sun, J, and Tang, J. A Survey of Models and Algorithms for Social Influence Analysis. In: Social Network Data Analytics. Boston, MA: Springer (2011). p. 177–214. doi:10.1007/978-1-4419-8462-3_7
33. Wasserman, S, and Faust, K. Social Network Analysis: Methods and Applications. Cambridge, UK: Cambridge University Press (1994).
34. Zhao, R, and Wang, J. Research of International Social Network Analysis in Frontier Domains in Visualized Information. Document, Inf Knowledge (2011) 32(1):88–94. (in Chinese).
35. Jager, W, and Amblard, Fd. r. Uniformity, Bipolarization and Pluriformity Captured as Generic Stylized Behavior with an Agent-Based Simulation Model of Attitude Change. Comput Math Organiz Theor (2005) 10(4):295–303. doi:10.1007/s10588-005-6282-2
36. Luo, S, Xia, H, and Yin, B. Continuous Opinion Dynamics on an Adaptive Coupled Random Network. Adv Complex Syst (2014) 17:03n041450012. doi:10.1142/s021952591450012x
37. Zhang, YM, Tang, CS, and Li, WG. Research on Interest Attenuation and Social Reinforcement Mechanism for Rumor Spreading in Online Social Networks. J China Soc Scientific Tech Inf (2015) 34:833–44.
38. Wang, J, Jiang, C, and Qian, J. Robustness of Interdependent Networks with Different Link Patterns against Cascading Failures. Physica A: Stat Mech its Appl (2014) 393:535–41. doi:10.1016/j.physa.2013.08.031
39. Wang, J, and Wang, X. Research on the Enterprise Public Opinion Spreading Model and Control Strategy in Two-Layer Social Networks. J Management Sci (2019) 32(1):28–41.
40. Xu, C. A Novel Recommendation Method Based on Social Network Using Matrix Factorization Technique. Inf Process Manag (2018) 54(3):463–74. doi:10.1016/j.ipm.2018.02.005
41. Xu, C, Ding, AS, and Liao, SS. A Privacy-Preserving Recommendation Method Based on Multi-Objective Optimisation for mobile Users. Int J Bio-Inspired Comput (2020) 16(1):23–32. doi:10.1504/ijbic.2020.108995
42. Xu, C, Ding, AS, and Zhao, K. A Novel POI Recommendation Method Based on Trust Relationship and Spatial-Temporal Factors. Electron Commerce Res Appl (2021) 48:101060. doi:10.1016/j.elerap.2021.101060
43. Xu, C, Liu, D, and Mei, X. Exploring an Efficient POI Recommendation Model Based on User Characteristics and Spatial-Temporal Factors. Mathematics (2021) 9(21):2673. doi:10.3390/math9212673
44. Han, X, Ju, C, Bao, F, Xu, C, Zhu, Y, and Chen, Y. Sharing Reward Program Based on Face Consciousness in Social Media. Psychol Res Behav Manag (2022) 15:1147–1166. doi:10.2147/PRBM.S362920
45. Bao, F, Mao, L, Zhu, Y, Xiao, C, and Xu, C. An Improved Evaluation Methodology for Mining Association Rules. Axioms (2021) 11(1):17. doi:10.3390/axioms11010017
46. Bao, F, Xu, W, Feng, Y, and Xu, C. A Topic-Rank Recommendation Model Based on Microblog Topic Relevance & User Preference Analysis. Hum-Cent Comput Inf Sci (2022) 12:10. doi:10.22967/HCIS.2022.12.010
47. Xiang, K, Xu, C, and Wang, J. Understanding the Relationship between Tourists' Consumption Behavior and Their Consumption Substitution Willingness under Unusual Environment. Psychol Res Behav Management (2021) 14:483–500. doi:10.2147/PRBM.S303239
48. Wang, J, Xu, C, and Liu, W. Understanding the Adoption of Mobile Social Payment? From the Cognitive Behavioral Perspective. Int J Mobile Commun (2022) 20(4):483–506. doi:10.1504/IJMC.2022.10036150
49. Lin, Y, Wang, X, Ma, H, Wang, L, Hao, F, and Cai, Z. An Efficient Approach to Sharing Edge Knowledge in 5G-Enabled Industrial Internet of Things. IEEE Trans Ind Inf (2022) 2022:1. doi:10.1109/TII.2022.3170470
50. Wang, X, Wang, X, Min, G, Hao, F, and Chen, C. An Efficient Feedback Control Mechanism for Positive/negative Information Spread in Online Social Networks. IEEE Trans Cybernetics (2020) 52(99):87–100. doi:10.1109/TCYB.2020.2977322
Keywords: rumor spreading, variation, coupled network, oyster, opinion interaction
Citation: Ju C, Wang C, Jiang Y, Bao F, Zhou H and Xu C (2022) Exploring a Multi-Layer Coupled Network Propagation Model Based on Information Diffusion and Bounded Trust. Int J Public Health 67:1604887. doi: 10.3389/ijph.2022.1604887
Received: 03 March 2022; Accepted: 03 June 2022;
Published: 18 July 2022.
Edited by:
Nino Kuenzli, Swiss Tropical and Public Health Institute (Swiss TPH), SwitzerlandCopyright © 2022 Ju, Wang, Jiang, Bao, Zhou and Xu. This is an open-access article distributed under the terms of the Creative Commons Attribution License (CC BY). The use, distribution or reproduction in other forums is permitted, provided the original author(s) and the copyright owner(s) are credited and that the original publication in this journal is cited, in accordance with accepted academic practice. No use, distribution or reproduction is permitted which does not comply with these terms.
*Correspondence: Fuguang Bao, YmFvZnVndWFuZ0AxMjYuY29t