- 1Department of Life Sciences and Public Health, Università Cattolica del Sacro Cuore, Rome, Italy
- 2Department of Prevention, AULSS6 Euganea, Padua, Italy
- 3Department of Cardiac, Thoracic, Vascular Sciences, and Public Health, Università degli Studi di Padova, Padua, Italy
- 4Cancer Screening Coordination Unit, Local Health Unit Roma 2, Rome, Italy
- 5Department of Biomedicine and Prevention, University of Rome “Tor Vergata”, Rome, Italy
- 6Agenzia Regionale per la Salute ed il Sociale ARESS, Bari, Italy
- 7Department of Woman and Child Health and Public Health, Fondazione Policlinico Universitario A. Gemelli IRCCS, Rome, Italy
Objectives: Physical activity (PA) is crucial for older adults’ wellbeing. Digital health interventions (DHIs) are important, however a synthesis aimed at healthy community-dwelling OA is lacking. This study aims to synthesize DHIs effect on PA levels among community-dwelling 60-year-old adults or older.
Methods: A systematic review was performed. DHIs using eHealth/mHealth tools, apps and text messaging were included. Primary outcomes were daily steps, moderate-to-vigorous PA and sedentary time. Quality was assessed via Cochrane risk-of-bias tools. Study-reported effect, study quality, sample size, study duration and dropout rate were semi-quantitatively synthesized to determine the overall category effect.
Results: 12 studies were included. 75% were low-quality, sample size was 16–18,080, study duration was 3–18 weeks, average dropout rate was 4.2%–46.7%. The synthesis of “motivational reminders” and “dynamic exercise programs” showed an overall positive effect, of “PA self-monitoring” showed mixed results and “exercise digital coaching” showed a non-positive effect.
Discussion: Motivational reminders and dynamic exercise programs proved more effective in increasing PA in older adults than other interventions and should be more embedded in structured public health programs.
Introduction
Physical activity (PA) is pivotal in maintaining the physical, mental, and social wellbeing of older adults (OA) [1, 2]. Regular PA effectively reduces the risk of chronic conditions [3], enhances cognitive function [4], fosters social interactions [5], and contributes to an improved quality of life (QoL) [6]. Walking, swimming, or yoga delays, for instance, the onset of cognitive impairment [7]. It fosters social connections, combating isolation in community settings [8]. Furthermore, PA is associated with improved psychological wellbeing, essential for QoL in OA [9]. For these reasons, the WHO (World Health Organization) aims to tackle this public health issue [10].
A significant proportion of OA remain inactive. Only a minority meets the WHO recommended levels [11, 12]. The prevalence of adults not meeting the recommended levels is 40%–60% among females aged 45–69 years and 50%–70% among females aged 70 years. In parallel, this prevalence is 30%–50% among males aged 45–69 years old and 40%–60% among 70-year-olds males and older across different WHO regions [12, 13].
In Europe, this prevalence among 55-year-olds and older varies widely, from 4.9% in Sweden to 29.0% in Portugal [14]. On average, OA spend around 9.4 h/day as sedentary [15]. This is often connected to retirement, fewer opportunities for social engagement, and a gradual decline in energy levels [16].
This poses a significant challenge, considering the proportion of people over 60 years old will nearly double by 2050 [17]. The positive effects of increased PA translate into a reduced disease and disability, with lower healthcare costs for chronic conditions management [18]. Moreover, regular PA extends life expectancy and also improves QoL by preserving independence, reducing the risk of disability, and promoting greater social engagement [19]. Digital health interventions (DHIs) emerge as promising opportunities for PA promotion among OA [20]. These interventions leverage information and communication technologies, such as websites, mobile applications, wearable devices, and telehealth platforms, to deliver personalized, scalable, and interactive PA interventions [21]. In 2017, Tedesco et al. reviewed the activity trackers for OA, detailing indicators like energy expenditure, activity assessment and sedentary behavior monitoring, all possible with commercially available devices [22]. The review emphasized the availability of several trackers specifically designed for OA, in addition to those for all ages. More evidence shows a growing interest in different trackers from major brands such as Fitbit and alike, used for interventions targeting the general population [23–25].
eHealth interventions have the potential to address various barriers faced by OA, including limited access to traditional exercise facilities [26]. The ever-increasing number of studies that analyze this public health aspect and the technologies involved corroborate its importance [20, 27–29] for health promotion strategy. However, a synthesis of DHIs aimed at healthy or with generic comorbidities community-dwelling OA is lacking.
The current study, therefore, aims to summarize the effect of DHIs interventions on PA levels of community-dwelling aged 60 years and older by a systematic review of the literature and semiquantitative analysis of the pertinent interventions from a health promotion and public health perspective. The objective is to provide evidence to guide public health policies, ensuring they align with the changing demographic landscape, evolving health needs, and technological advancements.
Methods
The following PICO was adopted to guide studies inclusion:
- Population (P): community-dwelling OA (60 years of age or older);
- Intervention (I): digital communication tools or devices (eHealth/mHealth interventions; Digital interventions; Text-messaging; App-based interventions);
- Comparator (C): control group, self (in case of within-subject design), standard practice;
- Outcome (O): PA level, active lifestyle or sedentary behavior.
This study adopted the FDA (Food and Drug Administration) definition of digital health, which includes “mobile health (mHealth), health information technology (IT), wearable devices, telehealth and telemedicine, and personalized medicine” [25].
Only papers with the following characteristics were included:
- Randomized controlled trials (RCTs) or quasi-experimental studies comparing a Digital Health PA intervention targeting OA aged 60 years and older with a digital or non-digital PA intervention, or no intervention were considered for inclusion.
- English papers with full-text availability were considered for inclusion.
- Studies examining community-dwelling OA, either healthy or with multiple non-severe comorbidities (i.e., diabetes, hypertension etc.) not affecting in any way the ability to perform PA as compared to the general population.
- Studies reporting digital/mHealth/eHealth interventions of any duration to increase regular PA levels or to reduce sedentary behavior time were included, covering interventions using apps, automated text-messaging, digital reminders, and automated digital coaching.
Papers with the following characteristics were excluded:
- Studies with subjects in rehabilitation, hospital inpatients or residents in any healthcare setting. Considering how a consistently shared definition of “older age” was lacking in literature, 60 years of age was adopted as a cut-off point.
- Studies including telephone-based interventions, unless pre-registered and fully automated, or with the tools not accessible via computer or handheld devices (telephones, smartphones, or tablets).
- Studies reporting interventions either specific to a single disease and/or addressing whatever grade of physical inability to exercise (i.e., an exercise program without upper extremity activity to a patient with scapular girdle arthrosis).
- Studies providing participants with self-tracking devices to monitor activities without any health promotion components, such as coaching, support, or motivation.
Only the following were included as comparators:
- A within-subject baseline assessment of the same outcome measures before the intervention;
- An alternative intervention (featuring non-digital tools or other digital tools) or delayed intervention;
- A no-intervention control group.
With regards to primary outcomes, improved levels of regular PA or reductions in sedentary behaviors, such as increasing daily steps, reduction of sedentary time, and increasing the frequency of MVPA sessions were included. In particular, only studies with PA measures either tracked objectively through digital measurement devices or recorded subjectively through other forms of structured data analysis (e.g., via validated scales/tools; studies relying on open-ended questionnaires were excluded), were included. The effect measures were intended as relative and/or absolute differences in PA levels and other outcomes, expressed as numbers or percentages, depending on the study.
With regards to secondary outcomes, the following were included: changes in health parameters such as weight, glycated hemoglobin (HbA1c), cardiovascular health parameters, QoL and adherence rates.
With regards to literature search, the electronic databases PubMed (MEDLINE), Web of Science and Scopus, were queried by AM and MDP. An appropriate search string was created using Boolean operators to combine multiple synonyms related to the following topics: populations aged 60 and older, digital health, mobile apps, communication media, physical activity, and sedentary behavior (see Supplementary Material S1 for the search strings in their exact syntax). Only English papers of interventional studies published after 01-01-2000 were included. The search string was rerun prior to final analysis; however, no new suitable studies were found.
With regards to study selection (see Figure 1), MDP and AM independently performed the process using Rayyan. Disagreements were resolved collectively.
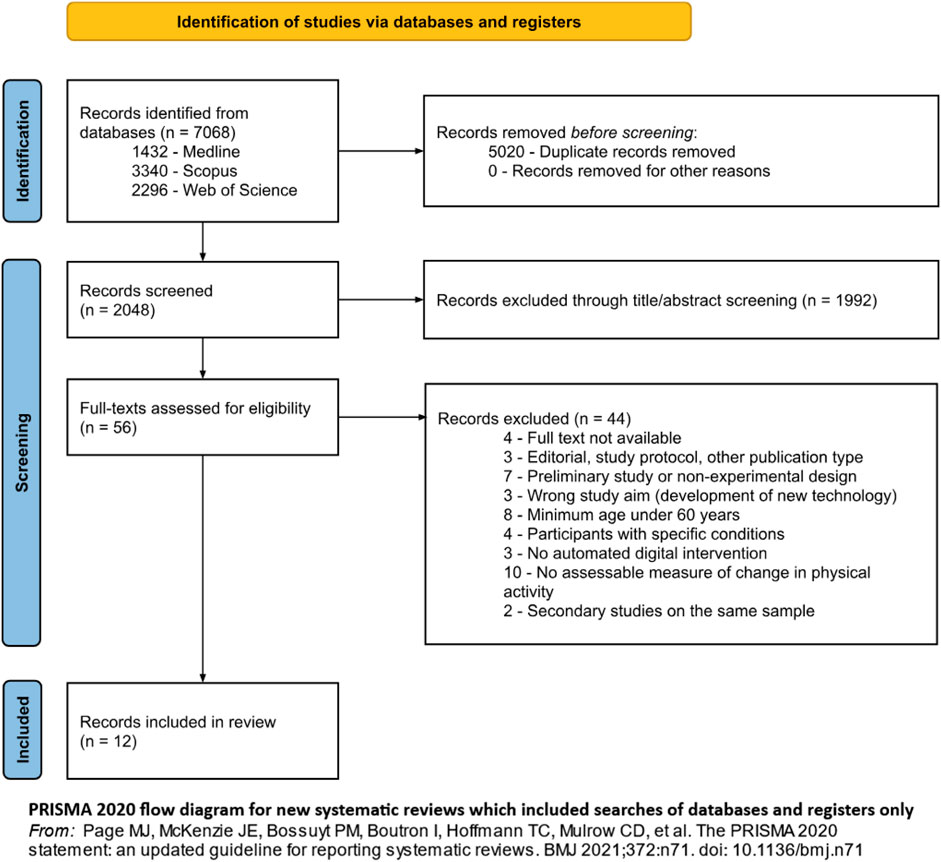
Figure 1. Flow diagram representing study selection and inclusion, from Page MJ et al. The PRISMA 2020 statement: an updated guideline for reporting systematic reviews. BMJ 2021; 372: n71. doi: 10.1136/bmj.n71 (Di Pumpo M. et al. Digital health interventions to promote physical activity in community-dwelling older adults: a systematic review and semiquantitative analysis. Padua, Italy. 2024).
After study selection, data extraction was performed by MDP and AM independently. Summary statistics were reported from original or performed following standard practice. No information relevant for synthesis was missing, no missing data handling techniques were required.
Regarding quality assessment, the included articles underwent a risk-of-bias (ROB) assessment by MDP and AM who independently applied the Cochrane tools. In detail, the Cochrane Rob2 tool was used for RCTs [30] and the Cochrane ROBINS-I tool [31] was used for non-randomized intervention studies. The tools’ final judgments were re-coded as high ROB (with “high risk” or “serious/critical” judgments obtained via the tools), medium ROB (“moderate” or “some concern” judgments obtained via the tools) and low ROB (with “low risk” judgments obtained via the tools) to render Rob2 and ROBINS-I tool overall judgments comparable. Among major items of ROB evaluation are the randomization process, deviation from intended interventions, missing outcome data, measurement of outcome, and selection of reported results. Among major items of ROBINS-I evaluation are confounding, participant selection, intervention classification, outcome measurement, missing data, reporting bias. Studies with a high ROB were classified as low quality, medium ROB as medium quality and high ROB as low quality (see Supplementary Material S2). This assessment subsequently constituted a key dimension of the evidence synthesis process.
Disagreements between MDP and AM were resolved collectively.
Given the heterogeneity of data in terms of intervention types and recorded outcomes, a meta-analysis was deemed not feasible. Instead, a semiquantitative analysis was performed via a harvest plot in order to synthesize the effect on PA levels of the studied interventions, and inform the discussion and recommendations. In particular, the “reported effect” for each study was classified as “positive” the original study statistically significant enhancement in PA levels (step counts, MVPA, sedentary time, see Table 1), or as “non-positive” if otherwise. The intervention characteristics extracted were: reported effect, study quality as assessed via the quality assessment, sample size (classified as under or over 100 participants), study duration (in weeks), the number of participants who completed or abandoned the intervention and the reasons for this. These characteristics were compounded in the semiquantitative synthesis to determine the overall category “effect” and are graphically displayed in Figures 2, 3.
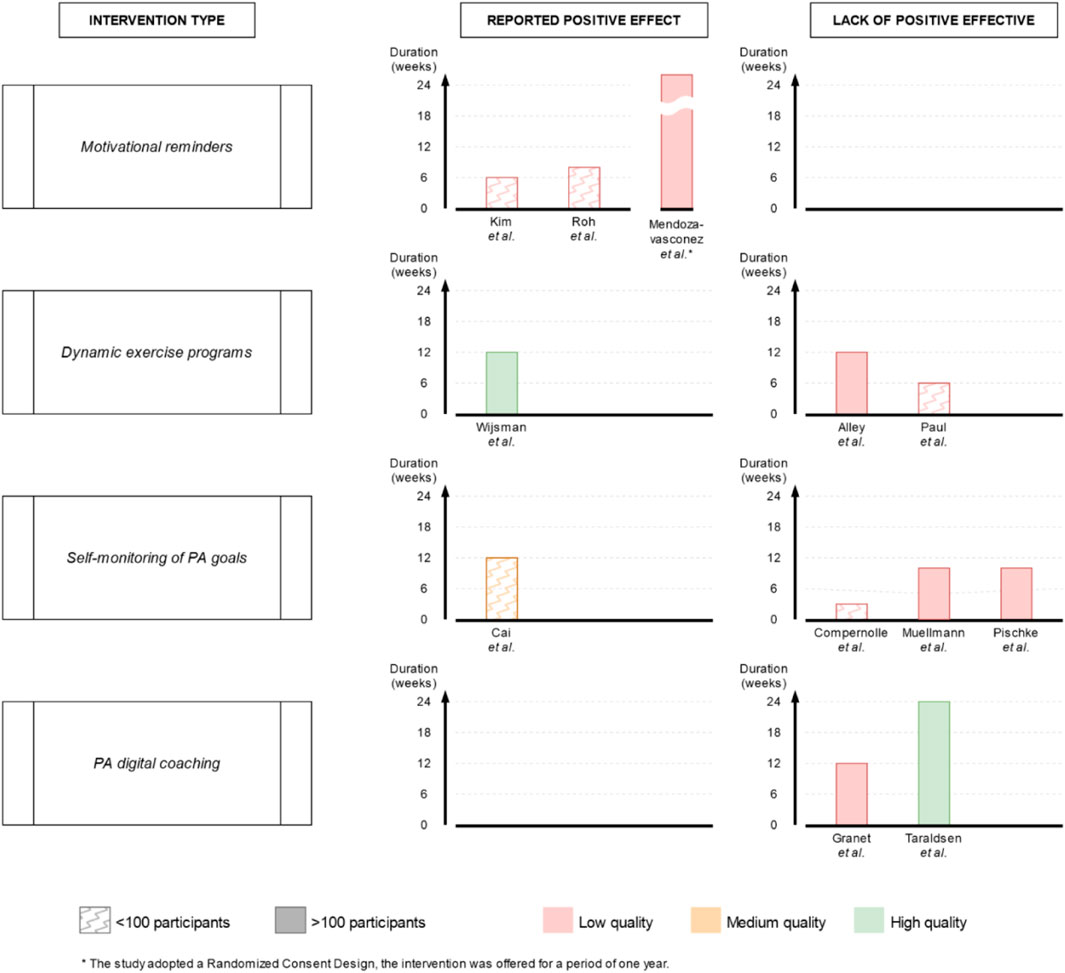
Figure 2. Harvest plot of intervention type by sample size, study quality, study duration and effect in increasing physical activity levels or reducing sedentary time (Di Pumpo M. et al. Digital health interventions to promote physical activity in community-dwelling older adults: a systematic review and semiquantitative analysis. Padua, Italy. 2024).
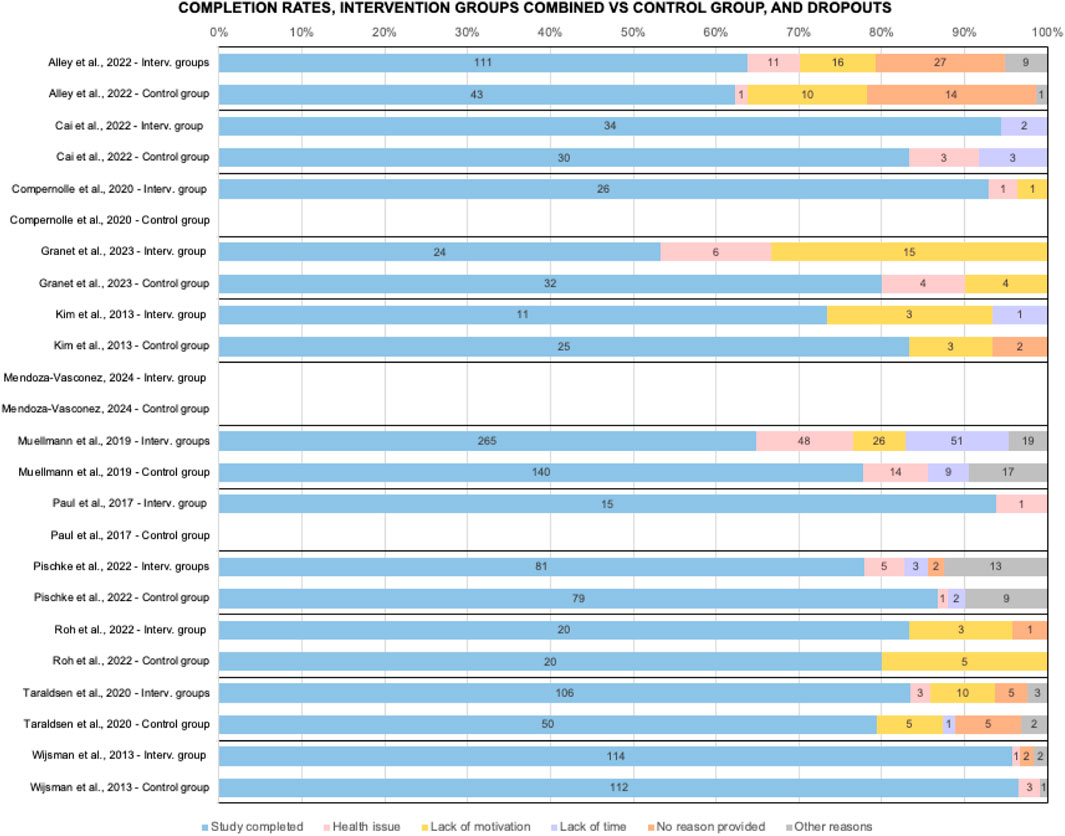
Figure 3. Comparison of studies on completion rates and number of dropouts by cause, interventions groups combined vs control group (when available) (Di Pumpo M. et al. Digital health interventions to promote physical activity in community-dwelling older adults: a systematic review and semiquantitative analysis. Padua, Italy. 2024).
Results
A total of 7,068 records were identified (see Figure 1 for details).
Of the 12 included studies, 9 were RCTs (Alley, 2022 [32], Cai, 2022 [33], Granet, 2023 [34], Kim, 2013 [35], Muellmann, 2019 [36], Pischke, 2022 [37], Roh, 2022 [38], Taraldsen, 2020 [39], Wijsman, 2013 [40]), 2 were mixed-method studies with a pre/post design (Compernolle, 2020 [41], Paul, 2017 [42]), and one was a Randomized Consent Design study (Mendoza-Vasconez, 2024 [43]). A three-arm study design was used in 4 studies (Alley, 2022, Muellmann, 2019, Pischke, 2022, Taraldsen, 2020), comparing a web-based intervention with PA diary functions, an accelerometer combined with a web-based intervention, and a control group; a two-arm design was used in 5 other studies (Cai, 2022, Granet, 2023, Kim, 2013, Roh, 2022, Wijsman, 2013) comparing accelerometer/pedometer-based intervention with no intervention; finally, two studies (Compernolle, 2020, Paul, 2017) featured a pre/post study design and one study adopted a Randomized Consent Design (Mendoza-Vasconez, 2024).
At baseline, the 12 studies included a total sample size of 19,746, ranging from 16 (Paul, 2017) to 18,080 (Mendoza-Vasconez, 2024) participants, with a median sample size of 132 participants. Female participants ranged from 40.9% (Wijsman, 2013 - 96 females in 235 participants) to 100% (Mendoza-Vasconez, 2024). Mean age samples ranged from 65.0 (Compernolle, 2020) to 83.1 years (Mendoza-Vasconez, 2024). Study duration ranged from 3 weeks (Compernolle, 2020) to 6 months (Taraldsen, 2020), with most studies lasting 12 weeks (Alley, 2022, Cai, 2022, Granet, 2023, Wijsman, 2013), and one study being an extension of a community health study started in 1991 (Mendoza-Vasconez, 2024) [44]. 6 out of the 12 studies were conducted in Europe (Compernolle, 2020, Muellmann, 2019, Paul, 2017, Pischke, 2022, Taraldsen, 2020, Wijsman, 2013), 3 in Asia (Alley, 2022, Cai, 2022, Roh, 2022) and 3 in North America (Granet, 2023, Kim, 2013, Mendoza-Vasconez, 2024).
Among the outcomes considered in this systematic review, four studies assessed daily steps (Cai, 2022, Compernolle, 2020, Kim, 2013, Paul, 2017), and four other studies evaluated MVPA (Alley, 2022, Muellmann, 2019, Pischke, 2022, Wijsman, 2013). Additionally, of these eight studies, four also measured total sedentary time (Compernolle, 2020, Alley, 2022, Muellmann, 2019, Pischke, 2022). The remaining four studies included in the review used alternative methods to assess physical activity levels, including the Rapid Assessment of Physical Activity questionnaire (Granet, 2023), time per week spent in all exercise-related activities (Mendoza-Vasconez, 2024), the number of days per week on which the intervention has been completed (Roh, 2022), METs (Mendoza-Vasconez, 2024, Roh, 2022), time per day spent walking and the Exercise Adherence Reporting Scale (Taraldsen, 2020).
In 6 out of 12 studies (Alley, 2022, Cai, 2022, Compernolle, 2020, Muellmann, 2019, Paul, 2017, Pischke, 2022) PA was quantitatively measured using digital devices (i.e., accelerometers, pedometers, smartphone sensors), in 3 studies (Granet, 2023, Roh, 2022, Taraldsen, 2020) PA was estimated through structured qualitative tools (via validated questionnaires and/or as adherence to the intervention protocol), in 3 studies PA was assessed using both quantitative and structured qualitative tools (Kim, 2013, Mendoza-Vasconez, 2024, Wijsman, 2013). Out of the 12 included studies, 2 did not rely on objective PA outcomes assessment (Granet, 2023 and Taraldsen, 2020).
Quality of Life was estimated in 3 out of 12 studies through specific validated questionnaires (Cai, 2022, Granet, 2023, Taraldsen, 2020), however, none of these studies found a significant difference in quality of life between intervention and control groups. Feasibility, acceptability, usability, user engagement and/or satisfaction were examined in 7 out of 12 studies using ad-hoc questionnaires (Alley, 2022, Compernolle, 2020, Granet, 2023, Muellmann, 2019, Paul, 2017, Pischke, 2022, Taraldsen, 2020). However, none of these studies demonstrated significant positive results in increasing physical activity levels or reducing sedentary time.
Supplementary Material S3 shows in detail the main intervention instruments adopted in each study. Four main conceptually distinctive types of intervention were identified (as shown in Figure 2):
• Motivational reminders (n = 3; Kim, 2013, Mendoza-Vasconez, 2024, Roh, 2022): messages and reminders aimed at promoting and sustaining participants' active behaviors, sent exclusively through automated tools, either individually or to the entire intervention group;
• Dynamic exercise programs (n = 3; Alley, 2022, Paul, 2022, Wijsman, 2013): tailored PA schedules made by an automated software using PA data gathered through a digital device, such as pedometers or accelerometers;
• Self-monitoring of PA goals (n = 4; Cai, 2022, Compernolle, 2020, Muellmann, 2019, Pischke, 2022): digital versions of a personal diary to record and monitor over time, with or without automatic data collection via digital device (e.g., pedometer, accelerometer) and peer support, the amount of physical activity performed and/or goals achieved;
• PA digital coaching (n = 2; Granet, 2023, Taraldsen, 2020): automated tools that provide instruction and guidance on performing physical exercises without the need for a human instructor available during training sessions.
A descriptive overview of the interventions for the promotion of physical activity in community-dwelling older adults, drawn from the studies included in the research, can be found as Supplementary Material S4.
Dropout rates ranged from 4.2% (Wijsman, 2013) to 46.7% (Granet, 2023). For larger studies average completion rate 72.6%, 79.3% in smaller studies (among controls was 81.7%). Excluding health problems (26.2% of dropouts from interventions), main causes were lack of motivation (25.5%), lack of time (19.7%) and others (15.9%).
Results related to secondary outcomes are shown in detail as Supplementary Material S5.
Discussion
The semiquantitative synthesis identified different effects on PA levels with mixed characteristics. For the “motivational reminders” category, a positive effect was reported by three low-quality studies. For the “dynamic exercise programs” category results were mixed, with a positive effect reported by one high-quality study with high sample size versus two contrasting low-quality studies. For “digital PA coaching,” no positive effect was reported by one high-quality and one low-quality study. For the “self-monitoring of PA goals” category a no positive effect was reported by three low quality studies versus one contrasting medium quality study.
A first consideration is that the overall quality of the studies was found not sufficient, with 75% of the studies resulting as low-quality. This advocates for the importance of elevated methodological rigor.
In the category “motivational reminders,” all 3 studies (Kim, 2013, Mendoza-Vasconez, 2024, Roh, 2022) showed significant positive effect on physical activity levels of participants. This result is in line with literature [45, 46], which suggests that additional support provided in daily life, alongside exercise prescription, can be effective in promoting PA. No studies implemented goal-oriented functions, such as targets for physical activity or digital rewards. Instead, all three issued periodic reminders via non-personalized messages. Nor automation, facilitation nor coaching were provided. Participants manually filled in a personal diary for track. By contrast, supporting tools as wearable trackers (Kim, 2013, Mendoza-Vasconez, 2024), written brochures (Mendoza-Vasconez, 2024, Roh, 2022) and human assistance upon request were provided (Kim, 2013, Mendoza-Vasconez, 2024). Although promising, there are some concerns about reproducibility of the results regarding “motivational reminders.” The quality assessment revealed serious issues in all three studies, and the duration of the interventions was either short (Kim, 2013 - 6 weeks - and Roh, 2022 - 8 weeks) or inconsistent (Mendoza-Vasconez, 2024 - duration of participation up to each participant due to the randomized consent design of the study). Therefore, the semiquantitative evaluation tends to support a positive effect on physical activity levels of this type of intervention, nonetheless only further high-quality research will be able to draw definitive conclusions.
The results in the category “dynamic exercise programs” were mixed, with only 1 out of three interventions showing efficacy (Wijsman, 2013 on the one hand, Alley, 2022 and Paul, 2017 on the other). The features adopted in two interventions by Alley. (2022) and Wijsman 2013 included a wearable displaying dynamic goals and a digital PA diary synchronized with it. Paul (2017) also included digital rewards and peer messaging. However, the positive result was from a high-quality, two-arm RCT studying 235 participants for a reasonable time (3 months). In comparison, Paul (2017) adopted a pre/post study design with only 16 participants, while Alley (2022) adopted a three-arm study design comparing two different interventions (with or without the use of wearable technology) and a control group, showing the lowest completion rates among all the studies over 100 participants (63.8% intervention, 62.3% controls). This suggests that well-designed interventions leveraging wearable devices for automated goal setting and tracking can significantly enhance PA levels in OA. Fanning [47], who showed how tailored PA interventions can significantly improve health outcomes in OA. Bravata [48] demonstrated that pedometers are associated with significant increases in PA and improvements in health outcomes. App-based technologies, which are dynamic and can be adapted at any time to the user’s needs, are proving increasingly useful for the promotion of PA in elderly and intergenerational contexts [46].
The “self-monitoring of PA goals” category results were also mixed. A medium-quality study (Cai, 2022) showed efficacy in increasing daily steps in the elderly, while three other low-quality studies (Compernolle, 2020, Muellmann, 2019, Pischke, 2022) did not indicate any significant increase in daily moderate-to-vigorous physical activity or reduction in daily sedentary time. Besides the results of the quality assessment, one feature was unique to Cai’s study intervention in this category, namely, that participants were asked to manually fill in the digital diary to keep track of their progress. This approach is similar to what was observed for “motivational reminders.” Other tools provided by the intervention were written brochures to guide PA, peer support through a messaging app and in-person group sessions with trained professionals and peers. Given the study’s limited sample size (72 participants randomized into two groups) and the overall complexity of the intervention, its results cannot provide a reliable indication of effect for interventions that rely on self-monitoring of PA targets. As aforementioned, the self-monitoring aspect reportedly motivates health behaviors [49]. However, without proper engaging features, such as periodic motivational reminders or software designed to require the active participation of the elderly, personal digital exercise diaries may fail to maintain user interest or to significantly impact behaviors, as indicated by Webb [50].
Furthermore, both studies in the “PA digital coaching” category showed no evidence of efficacy (Garnet, 2023, Taraldsen, 2020). Furthermore, one of these studies (Taraldsen, 2020) conducted the longest intervention of all those included in this review (6 months), used an RCT design, included 180 participants and was deemed high quality. Therefore, it appears that digital coaching of exercises, especially when delivered through pre-recorded or on-demand videos, do not significantly improve physical activity levels in the elderly. This finding aligns with the literature suggesting that, while digital coaching can provide valuable information, it lacks the personalized and interactive components that drive behavior change. Morrison [51] highlighted the limited effectiveness of interventions without personalized feedback or interaction. These results show how both tailored exercise plans, dynamically adjusted via wearables, and peer-supported personalized apps result more promising than standalone digital coaching or personal digital diaries. This is in line with the shift towards operational, personalized instructions, especially when embedded in structured institutional programs [52].
Completion rates showed notable variability, with dropout rates largely influenced by low motivation and limited time available. Knowing the positive effects of physical activity appears to be insufficient to make OA prioritize it [53]. This underscores the importance of increasing their self-awareness about physical activity and encouraging them to take an active role for their health. Promoting autonomy and adaptability of interventions supports sustained behavior change and improve both completion rates and engagement [54].
In addition, it is important to underline how many studies (Cai, 2022, Muellmann, 2019, Paul, 2017, Roh, 2022) included a “peer support” component (as shown in Supplementary Material S3), which can be particularly effective when combined with motivational feedback. Indeed, Patel [55] reported increased activity levels in users receiving both peer support and personalized step goals. Digital peer support platforms facilitate information sharing and social support, which are key for major behavioral change theories [47] stating that self-awareness can motivate health behaviors. Maher [56] underscores the potential of social networks in increasing PA by providing social support and normative influence. As aforementioned, the self-monitoring aspect reportedly motivates health behaviors [49]. The difference in personalization dimension could help explain part of the difference in the reported effect between some of the included studies.
Finally, regarding secondary outcomes, engagement with motivational reminders varied significantly depending on factors like initial physical activity levels and functional status. Their general effectiveness on secondary health behaviors, like diet or cognitive activity, was limited. Dynamic exercise programs showed potential in reducing sedentary behavior and improving metabolic and physical health indicators. However, the success of these programs seemed dependent on structured guidance and accountability measures. Also, the preference for specific components suggests that these programs could benefit targeted modifications to fully address OA’s preferences. Self-monitoring tools were generally well-received, particularly for their usability and design. However, self-monitoring alone may not drive substantial behavior change or physical health improvements, as engagement levels varied widely, and usage declined over time. Integrating them with direct guidance and support is key. Digital coaching, in contrast with other synthesis findings, showed good results on motivation and adherence. Interactive and real-time coaching formats generated higher satisfaction and greater improvements in physical outcomes than non-interactive sessions, confirming how personalized interaction and social support plays an important role.
In a time of personalized health promotion policies seem to lag behind [57], and PA change is an excellent field to incorporate individual needs [58] via an increasingly more user-centered design [59]. This field will be greatly benefit from AI-based technologies [60].
These changes cannot be implemented without collaboration between professionals, public health institutional representatives and decision-makers in order to implement effective strategies. Public health initiatives should encourage the adoption of these technologies and also facilitate the partnerships and infrastructure necessary to support their widespread use [10]. It is crucial that all stakeholders are aligned to leverage these promising tools, ensuring they are integrated into comprehensive, equitable, sustainable, and engaging public health interventions.
The success of such interventions in a public health perspective is largely dependent on addressing barriers and facilitators to the use of these tools by OA [61], digital health literacy promotion [62, 63] addressing both individuals and professionals (however young or formally trained) [64], increasing the engagement of both individuals [65] and communities, especially if disadvantaged, by using evidence-based established frameworks [66].
This review needs to be considered in light of its limitations and strengths.
First, the application of the Risk-of-bias tool proved somewhat challenging, considering some criteria were not strictly suitable for the specific nature of the public health intervention studies.
Second, the total number of included studies was not large. However, the adopted stringent cut-off criterion for participant age ensured the inclusion of OA while effectively excluding middle-aged individuals, thereby enhancing the relevance and specificity of our findings to the targeted demographic. Furthermore, the inclusion of only community-dwelling OA, healthy or with generic multiple non-severe comorbidities not limiting their ability to participate in PA, made the results more comparable and scalable to population level.
A careful consideration must be made regarding Mendoza-Vasconez, 2024 due to significant differences both in study design (participants were included using a Randomized Consent Design from a large sample of 18,080 female participants) and intervention protocol (it consists of an analysis of an intervention delivered simultaneously through different means of communication over a long period of time). Its inclusion does not however change the considerations regarding the interventions it involves, considering all studies as shown by the harvest plot.
Another limitation of the study might be represented by the exploratory intervention classification performed. However, even though acknowledging important digital health intervention classifications in scientific literature [67], the study was already much focused in terms of PICO to specific targets, so that a general framework might have lost in applicability. This choice should therefore be regarded as a strength of the study, as it enabled the tailoring of concepts and interventions to the aim of the study and allowed the specific innovative technologies and techniques to emerge.
A major strength of the study is its focus on the most recent evidence-based research on community-dwelling adults aged 60 and over, with an emphasis on scalable, population-level technologies such as app-based tools, wearables and fitness trackers. These tools have the potential to be included in future health promotion intervention impacting many individuals, thanks to their extensive accessibility and widespread distribution. Smartphones and wearables may, in fact, be pivotal in decentralizing healthcare, allowing for health monitoring and telemedicine to become more widely accessible and integrated into daily life [68].
Finally, the current work is partially inspired by Muellman [69], however it presents substantial differences in population inclusion criteria (i.e., age cut-off, community setting, clinical conditions and health status), search strings, and data synthesis. The most interesting feature was the harvest plot. The authors believe it represents a major strength of this study, as it enables simultaneous evaluation of multiple key dimensions of all studies with a single illustration.
In conclusion, the present study synthesizing evidence regarding (DHIs) for community-dwelling OA addresses a significant gap in the scientific literature. The studies included in this review demonstrate considerable heterogeneity in both methodology and digital features. Nonetheless, comparative analysis allowed us to identify promising elements that could enhance physical activity in OA. Notably, dynamic, digitally-tailored exercise plans and periodic motivational reminders were part of the interventions showing positive effects on physical activity levels, likely due to their ability to empower participants and sustain motivation. Conversely, DHIs relying primarily on automation and facilitation were less effective in increasing physical activity and reducing sedentary time. As digital devices become increasingly accessible to OA, policymakers should consider evaluating this diverse array of DHIs to identify and implement the most evidence-based, effective health promotion interventions tailored to this demographic.
Study Registration and Reporting
This systematic review is registered at PROSPERO (registration number: PROSPERO 2023 CRD42023470945. Available from: https://www.crd.york.ac.uk/prospero/display_record.php?ID=CRD42023470945). The research team followed the PRISMA guidelines (Preferred Reporting Items for Systematic Review and Meta-Analyses statement) for data search, extraction, synthesis and reporting.
Author Contributions
Following the CRediT Taxonomy (https://credit.niso.org/), Conceptualization was performed by AB and GD; Data curation was performed by AB, GD, MDP, and AM; Formal Analysis was performed by MDP and AM; Funding acquisition was not required; Investigation was performed by MDP and AM; Methodology was designed by AB, GD, MDP, and AM; Project administration was performed by MDP; Resources were made available by AB, GD, and MR; Software was made available by AM and MDP; Supervision was performed by AB, GD, and VB; Validation was performed by AB and GD; Visualization was performed by AM and MDP; Writing–original draft was performed by MDP and AM; Writing–review and editing was performed by MDP, AM, AB, GD, and MR. All authors contributed to the article and approved the submitted version.
Funding
The author(s) declare that financial support was received for the research, authorship, and/or publication of this article. This research has received funding from and was conducted within the activities of: “EMOTICon-Net: Stakeholders engagement per la creazione di un intervento personalizzato di promozione dell’attività fisica mediato da tecnologia digitale, per un assessment dei bisogni di salute finalizzato alla programmazione sanitaria,” funded by the Italian Ministry of Health, grant number B95E22000790001. This paper was developed within the project funded by Next-Generation EU - “Age-It - Ageing well in an aging society” project (PE0000015), National Recovery and Resilience Plan (NRRP) - PE8 - Mission 4, C2, Intervention 1.3”.
Author Disclaimer
The views and opinions expressed are only those of the authors and do not necessarily reflect those of the European Union or the European Commission. Neither the European Union nor the European Commission can be held responsible for them.
Conflict of Interest
The authors declare that they do not have any conflicts of interest.
Acknowledgments
The authors thank Dr. Luca Gino Sbrogiò, Director of the Department of Prevention of AULSS 6 Euganea, for his help and support. The authors acknowledge the use of a Generative AI tool (ChatGPT-4 by OpenAI) during the preparation of this work, with the sole purpose of improving readability and language use and in full compliance with ethical standards. After using this tool, the authors reviewed and edited the publication content and take full responsibility for it.
Supplementary Material
The Supplementary Material for this article can be found online at: https://www.ssph-journal.org/articles/10.3389/ijph.2024.1607720/full#supplementary-material
References
1. Nelson, ME, Rejeski, WJ, Blair, SN, Duncan, PW, Judge, JO, King, AC, et al. Physical Activity and Public Health in Older Adults: Recommendation From the American College of Sports Medicine and the American Heart Association. Circulation (2007) 116(9):1094–105. doi:10.1161/CIRCULATIONAHA.107.185650
2. Warburton, DER, Nicol, CW, and Bredin, SSD. Health Benefits of Physical Activity: The Evidence. CMAJ (2006) 174(6):801–9. doi:10.1503/cmaj.051351
3. Lee, IM, Shiroma, EJ, Lobelo, F, Puska, P, Blair, SN, Katzmarzyk, PT, et al. Effect of Physical Inactivity on Major Non-Communicable Diseases Worldwide: An Analysis of Burden of Disease and Life Expectancy. Lancet. (2012) 380(9838):219–29. doi:10.1016/S0140-6736(12)61031-9
4. Erickson, KI, Hillman, C, Stillman, CM, Ballard, RM, Bloodgood, B, Conroy, DE, et al. Physical Activity, Cognition, and Brain Outcomes: A Review of the 2018 Physical Activity Guidelines. Med and Sci Sports and Exerc (2019) 51(6):1242–51. doi:10.1249/MSS.0000000000001936
5. Chen, YRR, and Schulz, PJ. The Effect of Information Communication Technology Interventions on Reducing Social Isolation in the Elderly: A Systematic Review. J Med Internet Res (2016) 18(1):e18. doi:10.2196/jmir.4596
6. Garber, CE, Blissmer, B, Deschenes, MR, Franklin, BA, Lamonte, MJ, Lee, IM, et al. American College of Sports Medicine Position Stand. Quantity and Quality of Exercise for Developing and Maintaining Cardiorespiratory, Musculoskeletal, and Neuromotor Fitness in Apparently Healthy Adults: Guidance for Prescribing Exercise. Med and Sci Sports and Exerc (2011) 43(7):1334–59. doi:10.1249/MSS.0b013e318213fefb
7. Greene, C, Lee, H, and Thuret, S. In the Long Run: Physical Activity in Early Life and Cognitive Aging. Front Neurosci (2019) 13:884. doi:10.3389/fnins.2019.00884
8. Zaitsu, M, Kawachi, I, Ashida, T, Kondo, K, and Kondo, N. Participation in Community Group Activities Among Older Adults: Is Diversity of Group Membership Associated With Better Self-Rated Health? J Epidemiol (2018) 28(11):452–7. doi:10.2188/jea.JE20170152
9. Marquez, DX, Aguiñaga, S, Vásquez, PM, Conroy, DE, Erickson, KI, Hillman, C, et al. A Systematic Review of Physical Activity and Quality of Life and Well-Being. Translational Behav Med (2020) 10(5):1098–109. doi:10.1093/tbm/ibz198
10. World Health Organization. Global Action Plan on Physical Activity 2018–2030: More Active People for a Healthier World. Geneva: World Health Organization (2018). Available from: https://iris.who.int/handle/10665/272722 (Accessed January 30, 2024).
11. Sun, F, Norman, IJ, and While, AE. Physical Activity in Older People: A Systematic Review. BMC Public Health (2013) 13(1):449. doi:10.1186/1471-2458-13-449
12. Guthold, R, Stevens, GA, Riley, LM, and Bull, FC. Worldwide Trends in Insufficient Physical Activity From 2001 to 2016: A Pooled Analysis of 358 Population-Based Surveys With 1·9 Million Participants. Lancet Glob Health (2018) 6(10):e1077–86. doi:10.1016/S2214-109X(18)30357-7
13. World Health Organization. Global Status Report on Physical Activity 2022 (2024). Available from: https://www.who.int/publications-detail-redirect/9789240059153 (Accessed February 12, 2024).
14. Gomes, M, Figueiredo, D, Teixeira, L, Poveda, V, Paúl, C, Santos-Silva, A, et al. Physical Inactivity Among Older Adults Across Europe Based on the SHARE Database. Age and Ageing (2017) 46(1):71–7. doi:10.1093/ageing/afw165
15. Harvey, JA, Chastin, SFM, and Skelton, DA. How Sedentary Are Older People? A Systematic Review of the Amount of Sedentary Behavior. J Aging Phys Activity (2015) 23(3):471–87. doi:10.1123/japa.2014-0164
16. Chastin, SFM, Buck, C, Freiberger, E, Murphy, M, Brug, J, Cardon, G, et al. Systematic Literature Review of Determinants of Sedentary Behaviour in Older Adults: A DEDIPAC Study. Int J Behav Nutr Phys Activity (2015) 12(1):127. doi:10.1186/s12966-015-0292-3
17. Department of Economic and Social Affairs, Population Division. World Population Prospects 2019: Highlights. New York: United Nations (2019). Available from: https://population.un.org/wpp2019/ (Accessed December 18, 2023).
18. Duijvestijn, M, De Wit, GA, Van Gils, PF, and Wendel-Vos, GCW. Impact of Physical Activity on Healthcare Costs: A Systematic Review. BMC Health Serv Res (2023) 23(1):572. doi:10.1186/s12913-023-09556-8
19. Matthews, CE, George, SM, Moore, SC, Bowles, HR, Blair, A, Park, Y, et al. Amount of Time Spent in Sedentary Behaviors and Cause-Specific Mortality in US Adults. The Am J Clin Nutr (2012) 95(2):437–45. doi:10.3945/ajcn.111.019620
20. Kwan, RYC, Salihu, D, Lee, PH, Tse, M, Cheung, DSK, Roopsawang, I, et al. The Effect of E-Health Interventions Promoting Physical Activity in Older People: A Systematic Review and Meta-Analysis. Eur Rev Aging Phys Act (2020) 17(1):7. doi:10.1186/s11556-020-00239-5
22. Tedesco, S, Barton, J, and O’Flynn, B. A Review of Activity Trackers for Senior Citizens: Research Perspectives, Commercial Landscape and the Role of the Insurance Industry. Sensors (Basel, Switzerland) (2017) 17(6):1277. doi:10.3390/s17061277
23. Janevic, MR, Shute, V, Murphy, SL, and Piette, JD. Acceptability and Effects of Commercially Available Activity Trackers for Chronic Pain Management Among Older African American Adults. Pain Med (2020) 21(2):e68–78. doi:10.1093/pm/pnz215
24. Li, C, Chen, X, and Bi, X. Wearable Activity Trackers for Promoting Physical Activity: A Systematic Meta-Analytic Review. Int J Med Inform (2021) 152:104487. doi:10.1016/j.ijmedinf.2021.104487
25. Huhn, S, Axt, M, Gunga, HC, Maggioni, MA, Munga, S, Obor, D, et al. The Impact of Wearable Technologies in Health Research: Scoping Review. JMIR mHealth and uHealth (2022) 10(1):e34384. doi:10.2196/34384
26. Gell, NM, Rosenberg, DE, Demiris, G, LaCroix, AZ, and Patel, KV. Patterns of Technology Use Among Older Adults With and without Disabilities. Gerontologist (2015) 55(3):412–21. doi:10.1093/geront/gnt166
27. Daniels, K, Lemmens, R, Knippenberg, E, Marinus, N, Vonck, S, Baerts, J, et al. Promoting Physical Activity and a Healthy Active Lifestyle in Community-Dwelling Older Adults: A Design Thinking Approach for the Development of a Mobile Health Application. Front Public Health (2023) 11:1281923. doi:10.3389/fpubh.2023.1281923
28. Raquel Costa-Brito, A, Bovolini, A, Rúa-Alonso, M, Vaz, C, Francisco Ortega-Morán, J, Blas Pagador, J, et al. Home-Based Exercise Interventions Delivered by Technology in Older Adults: A Scoping Review of Technological Tools Usage. Int J Med Inform (2024) 181:105287. doi:10.1016/j.ijmedinf.2023.105287
29. Velciu, M, Spiru, L, Dan Marzan, M, Reithner, E, Geli, S, Borgogni, B, et al. How Technology-Based Interventions Can Sustain Ageing Well in the New Decade through the User-Driven Approach. Sustainability (2023) 15(13):10330. doi:10.3390/su151310330
30. Sterne, JAC, Savović, J, Page, MJ, Elbers, RG, Blencowe, NS, Boutron, I, et al. RoB 2: A Revised Tool for Assessing Risk of Bias in Randomised Trials. BMJ (2019) 366:l4898. doi:10.1136/bmj.l4898
31. Sterne, JA, Hernán, MA, Reeves, BC, Savović, J, Berkman, ND, Viswanathan, M, et al. ROBINS-I: A Tool for Assessing Risk of Bias in Non-randomised Studies of Interventions. BMJ (2016) 355:i4919. doi:10.1136/bmj.i4919
32. Alley, SJ, Uffelen, Jvan, Schoeppe, S, Parkinson, L, Hunt, S, Power, D, et al. The Effectiveness of a Computer-Tailored Web-Based Physical Activity Intervention Using Fitbit Activity Trackers in Older Adults (Active for Life): Randomized Controlled Trial. J Med Internet Res (2022) 24(5):e31352. doi:10.2196/31352
33. Cai, X, Qiu, S, Luo, D, Li, R, Liu, C, Lu, Y, et al. Effects of Peer Support and Mobile Application-Based Walking Programme on Physical Activity and Physical Function in Rural Older Adults: A Cluster Randomized Controlled Trial. Eur Geriatr Med (2022) 13(5):1187–95. doi:10.1007/s41999-022-00682-w
34. Granet, J, Peyrusqué, E, Ruiz, F, Buckinx, F, Abdelkader, LB, Dang-Vu, TT, et al. Web-Based Physical Activity Interventions Are Feasible and Beneficial Solutions to Prevent Physical and Mental Health Declines in Community-Dwelling Older Adults During Isolation Periods. The Journals Gerontol Ser A (2023) 78(3):535–44. doi:10.1093/gerona/glac127
35. Kim, BH, and Glanz, K. Text Messaging to Motivate Walking in Older African Americans: A Randomized Controlled Trial. Am J Prev Med (2013) 44(1):71–5. doi:10.1016/j.amepre.2012.09.050
36. Muellmann, S, Buck, C, Voelcker-Rehage, C, Bragina, I, Lippke, S, Meyer, J, et al. Effects of Two Web-Based Interventions Promoting Physical Activity Among Older Adults Compared to a Delayed Intervention Control Group in Northwestern Germany: Results of the PROMOTE Community-Based Intervention Trial. Prev Med Rep (2019) 15:100958. doi:10.1016/j.pmedr.2019.100958
37. Pischke, CR, Voelcker-Rehage, C, Ratz, T, Peters, M, Buck, C, Meyer, J, et al. Web-Based Versus Print-Based Physical Activity Intervention for Community-Dwelling Older Adults: Crossover Randomized Trial. JMIR mHealth and uHealth (2022) 10(3):e32212. doi:10.2196/32212
38. Roh, HW, Ryu, H, Jeong, S, Han, J, Park, B, Moon, SY, et al. The Effectiveness of a Motivational Enhancement Smartphone Application Promoting Lifestyle Improvement for Brain Health: A Randomized Controlled Trial. PLoS ONE (2022) 17 (6):e0267806. doi:10.1371/journal.pone.0267806
39. Taraldsen, K, Mikolaizak, AS, Maier, AB, Mellone, S, Boulton, E, Aminian, K, et al. Digital Technology to Deliver a Lifestyle-Integrated Exercise Intervention in Young Seniors—The PreventIT Feasibility Randomized Controlled Trial. Front Digital Health (2020) 2:10. doi:10.3389/fdgth.2020.00010
40. Wijsman, CA, Westendorp, RG, Verhagen, EA, Catt, M, Slagboom, PE, Craen, AJde, et al. Effects of a Web-Based Intervention on Physical Activity and Metabolism in Older Adults: Randomized Controlled Trial. J Med Internet Res (2013) 15(11):e233. doi:10.2196/jmir.2843
41. Compernolle, S, Cardon, G, Ploeg, HPvan der, Nassau, FV, Bourdeaudhuij, ID, Jelsma, JJ, et al. Engagement, Acceptability, Usability, and Preliminary Efficacy of a Self-Monitoring Mobile Health Intervention to Reduce Sedentary Behavior in Belgian Older Adults: Mixed Methods Study. JMIR mHealth and uHealth (2020) 8(10):e18653. doi:10.2196/18653
42. Paul, L, Brewster, S, Wyke, S, McFadyen, AK, Sattar, N, Gill, JM, et al. Increasing Physical Activity in Older Adults Using STARFISH, an Interactive Smartphone Application (App); a Pilot Study. J Rehabil Assistive Tech Eng (2017) 4:2055668317696236. doi:10.1177/2055668317696236
43. Mendoza-Vasconez, AS, King, AC, Chandler, G, Mackey, S, Follis, S, and Stefanick, ML. Engagement with Remote Delivery Channels in a Physical Activity Intervention for Senior Women in the US. Am J Health Promot (2024) 38(5):692–703.
44. Stefanick, ML, Kooperberg, C, and LaCroix, AZ. Women’s Health Initiative Strong and Healthy (WHISH): A Pragmatic Physical Activity Intervention Trial for Cardiovascular Disease Prevention. Contemp Clin Trials (2022) 119:106815. doi:10.1016/j.cct.2022.106815
45. Direito, A, Carraça, E, Rawstorn, J, Whittaker, R, and Maddison, R. mHealth Technologies to Influence Physical Activity and Sedentary Behaviors: Behavior Change Techniques, Systematic Review and Meta-Analysis of Randomized Controlled Trials. Ann Behav Med (2017) 51(2):226–39. doi:10.1007/s12160-016-9846-0
46. King, AC, Hekler, EB, Grieco, LA, Winter, SJ, Sheats, JL, Buman, MP, et al. Harnessing Different Motivational Frames Via Mobile Phones to Promote Daily Physical Activity and Reduce Sedentary Behavior in Aging Adults. PLoS ONE (2013) 8 (4):e62613. doi:10.1371/journal.pone.0062613
47. Fanning, J, Porter, G, Awick, EA, Ehlers, DK, Roberts, SA, Cooke, G, et al. Replacing Sedentary Time With Sleep, Light, or Moderate-To-Vigorous Physical Activity: Effects on Self-Regulation and Executive Functioning. J Behav Med (2017) 40(2):332–42. doi:10.1007/s10865-016-9788-9
48. Bravata, DM, Smith-Spangler, C, Sundaram, V, Gienger, AL, Lin, N, Lewis, R, et al. Using Pedometers to Increase Physical Activity and Improve Health: A Systematic Review. JAMA (2007) 298(19):2296–304. doi:10.1001/jama.298.19.2296
49. Glanz, K, Rimer, BK, and Viswanath, K. Health Behavior and Health Education: Theory, Research, and Practice. John Wiley and Sons (2008). 968.
50. Webb, TL, Joseph, J, Yardley, L, and Michie, S. Using the Internet to Promote Health Behavior Change: A Systematic Review and Meta-Analysis of the Impact of Theoretical Basis, Use of Behavior Change Techniques, and Mode of Delivery on Efficacy. J Med Internet Res (2010) 12(1):e4. doi:10.2196/jmir.1376
51. Morrison, L, Moss-Morris, R, Michie, S, and Yardley, L. Optimizing Engagement with Internet-based Health Behaviour Change Interventions: Comparison of Self-assessment With and without Tailored Feedback Using a Mixed Methods Approach. Br J Health Psychol (2014) 19(4):839–55. doi:10.1111/bjhp.12083
52. Gassner, L, Zechmeister-Koss, I, and Reinsperger, I. National Strategies for Preventing and Managing Non-communicable Diseases in Selected Countries. Front Public Health (2022) 10:838051. doi:10.3389/fpubh.2022.838051
53. Franco, MR, Tong, A, Howard, K, Sherrington, C, Ferreira, PH, Pinto, RZ, et al. Older People’s Perspectives on Participation in Physical Activity: A Systematic Review and Thematic Synthesis of Qualitative Literature. Br J Sports Med (2015) 49(19):1268–76. doi:10.1136/bjsports-2014-094015
54. Collado-Mateo, D, Lavín-Pérez, AM, Peñacoba, C, Del Coso, J, Leyton-Román, M, Luque-Casado, A, et al. Key Factors Associated with Adherence to Physical Exercise in Patients With Chronic Diseases and Older Adults: An Umbrella Review. Int J Environ Res Public Health (2021) 18(4):2023. doi:10.3390/ijerph18042023
55. Patel, MS, Benjamin, EJ, Volpp, KG, Fox, CS, Small, DS, Massaro, JM, et al. Effect of a Game-Based Intervention Designed to Enhance Social Incentives to Increase Physical Activity Among Families: The BE FIT Randomized Clinical Trial. JAMA Intern Med (2017) 177(11):1586–93. doi:10.1001/jamainternmed.2017.3458
56. Maher, C, Ferguson, M, Vandelanotte, C, Plotnikoff, R, De Bourdeaudhuij, I, Thomas, S, et al. A Web-Based, Social Networking Physical Activity Intervention for Insufficiently Active Adults Delivered via Facebook App: Randomized Controlled Trial. J Med Internet Res (2015) 17(7):e174. doi:10.2196/jmir.4086
57. Stark, AL, Geukes, C, and Dockweiler, C. Digital Health Promotion and Prevention in Settings: Scoping Review. J Med Internet Res (2022) 24(1):e21063. doi:10.2196/21063
58. Conroy, DE, Bennett, GG, Lagoa, CM, and Wolin, KY. Steps Towards Digital Tools for Personalised Physical Activity Promotion. Br J Sports Med (2022) 56(8):424–5. doi:10.1136/bjsports-2021-104169
59. Revenäs, Å, Ström, L, Cicchetti, A, and Ehn, M. Toward Digital Inclusion of Older Adults in E-Health: A Case Study on Support for Physical Activity. Univ Access Inf Soc (2023). doi:10.1007/s10209-023-01049-z
60. Wong, DC, O’Connor, S, and Stanmore, E. The Application of Artificial Intelligence in Digital Physical Activity and Falls Prevention Interventions for Older Adults. J Aging Phys Act (2023) 31(5):887–9. doi:10.1123/japa.2022-0376
61. Wilson, J, Heinsch, M, Betts, D, Booth, D, and Kay-Lambkin, F. Barriers and Facilitators to the Use of E-Health by Older Adults: A Scoping Review. BMC Public Health (2021) 21(1):1556. doi:10.1186/s12889-021-11623-w
62. Jung, SO, Son, YH, and Choi, E. E-Health Literacy in Older Adults: An Evolutionary Concept Analysis. BMC Med Inform Decis Mak (2022) 22(1):28. doi:10.1186/s12911-022-01761-5
63. Martins, A, Andrade, I, Pocinho, R, and Belo, P. E-Health Literacy in Ageing. In: Proceedings of the 3rd International Conference on Technological Ecosystems for Enhancing Multiculturality. Porto Portugal: ACM (2015). 53–7. doi:10.1145/2808580.2808589
64. Casà, C, Marotta, C, Di Pumpo, M, Cozzolino, A, D’Aviero, A, Frisicale, EM, et al. COVID-19 and Digital Competencies Among Young Physicians: Are We (Really) Ready for the New Era? A National Survey of the Italian Young Doctors Association. Annali dell’Istituto Superiore di Sanità (2021) 57(1):1–6. doi:10.4415/ANN_21_01_01
65. Krist, AH, Tong, ST, Aycock, RA, and Longo, DR. Engaging Patients in Decision-Making and Behavior Change to Promote Prevention. In Health literacy: new directions in research, theory and practice. (2017). Washington: IOS Press, 284–302. (Studies in Health Technology and Informatics; vol. 240). Available at: https://ebooks.iospress.nl/doi/10.3233/978-1-61499-790-0-284 (cited December 11, 2024).
66. Riccardi, MT, Pettinicchio, V, Di Pumpo, M, Altamura, G, Nurchis, MC, Markovic, R, et al. Community-based Participatory Research to Engage Disadvantaged Communities: Levels of Engagement Reached and How to Increase it. A Systematic Review. Health Policy (2023) 137:104905. doi:10.1016/j.healthpol.2023.104905
67. World Health Organization. Classification of Digital Interventions, Services and Applications in Health: A Shared Language to Describe the Uses of Digital Technology for Health (2023). Available from: https://www.who.int/publications/i/item/9789240081949 (Accessed January 16, 2024).
68. Wall, C, Hetherington, V, and Godfrey, A. Beyond the Clinic: The Rise of Wearables and Smartphones in Decentralising Healthcare. Npj Digit Med (2023) 6(1):219. doi:10.1038/s41746-023-00971-z
Keywords: ageing, exercise, physical fitness, healthy lifestyle, digital health
Citation: Di Pumpo M, Miatton A, Riccardi MT, Graps EA, Baldo V, Buja A and Damiani G (2025) Digital Health Interventions to Promote Physical Activity in Community-Dwelling Older Adults: A Systematic Review and Semiquantitative Analysis. Int J Public Health 69:1607720. doi: 10.3389/ijph.2024.1607720
Received: 01 July 2024; Accepted: 29 November 2024;
Published: 03 January 2025.
Edited by:
Alberto Borraccino, University of Turin, ItalyReviewed by:
H. Ranjani, Madras Diabetes Research Foundation, IndiaOne reviewer who chose to remain anonymous
Copyright © 2025 Di Pumpo, Miatton, Riccardi, Graps, Baldo, Buja and Damiani. This is an open-access article distributed under the terms of the Creative Commons Attribution License (CC BY). The use, distribution or reproduction in other forums is permitted, provided the original author(s) and the copyright owner(s) are credited and that the original publication in this journal is cited, in accordance with accepted academic practice. No use, distribution or reproduction is permitted which does not comply with these terms.
*Correspondence: M. Di Pumpo, bWFyY2VsbG8uZGlwdW1wb0B1bmljYXR0Lml0
†These authors have contributed equally to this work and share first authorship
‡These authors have contributed equally to this work and share last authorship