- 1School of Media and Communication, Shanghai Jiao Tong University, Shanghai, China
- 2China Institute for Urban Governance, Shanghai Jiao Tong University, Shanghai, China
- 3School of International and Public Affairs, Shanghai Jiao Tong University, Shanghai, China
- 4School of Journalism, Fudan University, Shanghai, China
Objectives: People’s mental health and digital usage have attracted widespread attention during the COVID-19 pandemic. This study aimed to investigate how social media overload influenced depressive symptoms under the COVID-19 infodemic and the role of risk perception and social media fatigue.
Methods: A questionnaire survey was conducted on 644 college students during the COVID-19 lockdown in Shanghai, and data analysis was conducted using the PROCESS4.0 tool.
Results: The findings showed that in the COVID-19 information epidemic: 1) both information overload and communication overload were significantly and positively associated with depressive symptoms; 2) risk perception of COVID-19, and social media fatigue mediated this association separately; 3) and there was a chain mediating relationship between communication overload and depressive symptoms.
Conclusion: Social media overload was positively associated with depressive symptoms among college students under the COVID-19 infodemic by increasing risk perception and social media fatigue. The findings sparked further thinking on how the public should correctly use social media for risk communication during public health emergencies.
Introduction
COVID-19 has been regarded as the first pandemic in the digital age [1]. According to the World Health Organization (WHO), healthcare institutions are battling not only epidemics but also the infodemic. In the early stages of the Omicron wave, Shanghai experienced a citywide lockdown, during which rumors and misinformation quickly spread through social media. Compared to traditional media, social media are frequently seen as being more interactive, actual, credible, and dialogic [2]. Through social media, epidemic information spreads faster and more widely. Since the outbreak of the COVID-19 pandemic, people’s use of social media has increased significantly [3]. People rely heavily on social media to get information and connect with others. Social media reflects people’s attention to public health emergencies [4]. The role of the media expected by society is to provide accurate and timely information about epidemics so that citizens can respond promptly [5]. However, social media is not only a platform for information transmission and risk communication but also an amplification station for overloaded information and communication. The concept of social overload was first proposed to describe the negative effects of population congestion [6]. According to the bounded rationality theory, Social media overload refers to an individual’s social media processing capacity falling short of the massive information and social input [7].
A systematic review pointed out that four aspects of social media including time spent on, activity, investment, and addiction are associated with negative mental health outcomes such as depression, anxiety, and stress [8]. Research showed that the popularity of COVID-19 has affected people’s mental health, the prevalence of depressive symptoms during the COVID-19 pandemic was more than three times higher than before the pandemic [9]. On the Chinese search engine Baidu, there is an upsurge in inquiries about depression [10]. Besides, rates of depression among college students are significantly greater than those in the general population [11]. A systematic review found that the prevalence of depressive symptoms was 34% among higher education students, which was higher compared to pre-pandemic prevalence in similar populations [12]. It is necessary to explore the predictors of depressive symptoms and their influence mechanism among college students during the pandemic lockdown.
This study explores the potential mechanism of the impact of social media overload on depression symptoms among college students in the context of the infodemic. The present study has three purposes: 1) to provide a new perspective on the influencing factors of college students’ mental health. 2) Examine the underlying mechanism of how social media overload affects depressive symptoms during the lockdown. 3) Explore further strategies for the proper use of social media during a pandemic to avoid negative effects on mental health.
Social Media Overload and Depressive Symptoms
Social media which is built on the philosophical and technical principles of Web 2.0 are defined as internet applications that enable the production and distribution of user-generated content [13]. There are two primary domains of social media overload: information overload (IO) and communication overload (CO). IO refers to an individual’s information processing ability that cannot meet massive information inputs at a high rate [14, 15]. The information redundancy and low quality of information brought by social media cause people to spend a lot of time processing information, which brings negative emotions [16, 17]. Previous studies pointed out that COVID-19 information overload positively affects depressive symptoms [18, 19]. CO is defined as a situation when communication demands from diverse sources exceed an individual’s communication capacity [20, 21]. Social media is used as a means of rapid communication in a crisis [16]. One study proved that interpersonal communication about COVID-19 had no significant effect on depression [17]. However, the means of social media communication include not only person-to-person communication but also group communication and mass communication [22]. Overloaded communication about the pandemic may magnify the severity of the pandemic, and increase worry and stress, which may result in depressive symptoms. Reinecke et al. pointed out that communication overload had a significant indirect effect on depression through perceived stress [23]. However, according to a survey of Romanian social media users, excessive COVID-19-related information had no significant effect on depression during the lockdown [1]. Therefore, the association between social media overload and depressive symptoms is still to be confirmed, we hypothesized the following:
H1a. Information overload will be positively associated with depressive symptoms
H1b. Communication overload will be positively associated with depressive symptoms
The Mediating Role of Risk Perception of COVID-19
Risk perception refers to people’s subjective judgments and evaluations of hazards that they are or may experience [24, 25]. Social media plays an irreplaceable role in shaping people’s risk perceptions [26]. It has huge potential in forming an individual’s perception of risk, conceptions, fears, and opinions collide in social media interactions [27]. Kasperson et al put forward the “social amplification of risk” framework, considering social media as an amplification for perception of risk [25]. High social media exposure was substantially linked to greater levels of COVID-19 risk perception, anxiety, and depression [28]. It has been proved that information overload was positively related to perceived risk of the vaccine [27], and colon cancer risk perceptions [29]. Students with high levels of information-seeking reported a greater risk perception of COVID-19 [30]. Dryhurst suggested that social amplification through friends and families were important determinants of risk perception [31]. During the COVID-19 lockdown, online interaction with family and friends through social media has been the main channel of communication. Hence, information overload and communication overload may have a positive effect on the risk perception of COVID-19. Besides, risk perception has been widely examined as a predictor of depression [32, 33]. One study showed that affective risk perception had a positive effect on depression, while cognitive risk perception has a negative effect on depression [34]. Hence, we propose the following hypotheses:
H2a. Risk perception of COVID-19 mediates the relationship between information overload and depressive symptoms
H2b. Risk perception of COVID-19 mediates the relationship between communication overload and depressive symptoms
The Mediating Role of Social Media Fatigue
Social media fatigue (SMF) is defined as negative emotions such as exhaustion and tiredness caused by social media use, and the tendency to withdraw from social media [21, 35]. Individuals may feel overwhelmed, fatigued, and anxious when faced with massive information and communication [7, 36]. The stressor-strain-outcome (SSO) model is the main theoretical framework to explain social media fatigue. This model reveals how social media overload affects strain variables. Based on the SSO model, studies found that information overload and communication overload were significant stressors of social media fatigue [37, 38]. A study in the context of the pandemic also suggested that information overload significantly affects emotional exhaustion [39]. However, one study holds a different opinion that communication overload had no significant effect on fatigue [40]. In addition, social media fatigue is an important predictor of negative psychological health outcomes, such as anxiety, stress, and depression [37, 41]. COVID-19-related fatigue was found to predict depression in a significant and beneficial way [42]. It has also been found that social media fatigue mediated the association between information overload and social anxiety [43]. Therefore, we proposed the following assumptions:
H3a. Social media fatigue (SMF) mediates the relationship between information overload and depressive symptoms
H3b. Social media fatigue (SMF) mediates the relationship between communication overload and depressive symptoms
The Chain Mediating Model
Perceived risk of COVID-19 was negatively related to mental health, and emotions mediated this relationship [44]. Risk perception of SNS may increase social network fatigue [45]. In the context of the COVID-19 pandemic, some studies explored the relationship between risk perception and fatigue. One study showed that the effect of fatigue on protective behaviors reduced as the perceived risk of COVID-19 increased [46]. Another study pointed out that pandemic risk perception can be also a predictor of pandemic fatigue through the mediation of perceived stress and preventive coping [47]. Different kinds of fatigue have been proven to be associated with risk perception. Employee fatigue in healthcare settings has been the subject of numerous types of research. Job-related risks increase employees’ susceptibility to exhaustion [48]. Social media amplifies the level of risk of COVID-19, and when people perceive more risk, they will become tired and reluctant to social media. Therefore, we proposed the following assumptions:
H4a. Information overload will be positively associated with depressive symptoms through the chain mediating role of risk perception of COVID-19 and social media fatigue.
H4b. Communication overload will be positively associated with depressive symptoms through the chain mediating role of risk perception of COVID-19 and social media fatigue.
Figure 1 shows the theoretical model of the relationship between social media overload and depressive symptoms.
Methods
Sample and procedure
Between the 25th of March and the 13th of April 2022, we surveyed a group of university students enrolled in Shanghai using Wen Juan Xing (www.wjx.cn), the largest online survey platform in China, which is the equivalent of Qualtrics and SurveyMonkey, providing online data for business and academic fields [49–53]. Its sample database contains over 2.6 million respondents who confirmed their personal information [52], and more than 10 million people on average fill out questionnaires on the platform every day, reaching nearly 300 million users every month, and accurate questionnaires can be placed according to user interest labels and specific groups now. Our survey was conducted during the COVID-19 pandemic and the Omicron Wave Lockdown in Shanghai. The specific steps were that the Wen Juan Xing survey platform first randomly selected respondents who met the requirements for interview and data collection in its huge sample pool and then invited this group of respondents to find new potential respondents to answer the questionnaire through social media and other means, and in this way circulated the respondents who forwarded the diffusion questionnaire until the number of questionnaires recovered reached 800. The average response time per student was 810.25 s, and after excluding invalid samples based on response time and basic information, and further controlling the sample with quotas based on age, gender, and education information, 644 valid samples were finally retained.
Respondents’ Information
The total sample included 383 female and 261 male college students, with a larger proportion of female (59.5%) respondents than male (40.5%). The proportion of respondents under the age of 20 was 18.3%, and the largest proportion were in the 20–21 (30.4%) and 22–23 (30.1%) age groups, but the smallest proportion was in the 30 and above (0.3%) age group. In terms of the academic stage, the largest number of respondents were undergraduate students (80.4%), followed by master’s students (14.9%) and doctoral students (4.6%). 63.2% of respondents had a campus outbreak at their school, 64.3% lived in the school dormitory, but only 1.1% of respondents had COVID-19 negative. In addition, during the Shanghai Omicron Wave Lockdown period, most respondents spent 1–3 h (45.3%) and 4–5 h (28.4%) per day on social media, but 12.7% of respondents spent more than 5 h per day on social media and 13.5% spent less than 1 h per day or did not use it.
Measures
Information Overload (IO)
Information overload was assessed by adapting scales from Zhang et al.’s study [54]. The scale contains four items and was measured using a five-degree Likert scale (1 = strongly disagree, 5 = strongly agree). The results of respondents’ responses were aggregated and the mean was calculated to measure the extent of information overload. Its Cronbach’s α is 0.832, and five items had factor loadings values of 0.535, 0.725, 0.765, 0.779, and 0.709 for the confirmatory factor analysis (CFA).
Communication Overload (CO)
It was measured concerning Cho et al.’s study [55, 56]. The scale contains four items and was measured using a five-degree Likert scale (1 = strongly disagree, 5 = strongly agree). Four five-point Likert scale items (1 = strongly disagree, 5 = strongly agree) were used to measure respondents’ level of agreement. The results of respondents’ replies were aggregated and the mean was calculated to measure the degree of communication overload. Its Cronbach’s α is 0.668, and the factor loadings values of CFA for the four items were 0.628, 0.636, 0.569, and 0.568.
Risk Perception of COVID-19 (RPC)
Risk Perception of COVID-19 was measured based on the scale developed by Zhuang et al. [57] Four five-point Likert scale items (1 = strongly disagree, 5 = strongly agree) were used to measure risk perception of COVID-19. Respondents’ responses were summarized and the mean was calculated to measure the extent of risk perception of COVID-19. Its Cronbach’s α is 0.795, and four items had CFA factor loadings values of 0.582, 0.531, 0.840, and 0.840.
Social Media Fatigue (SMF)
Social Media Fatigue was measured from a study by Maier et al. [58]. Three five-point Likert scale items (1 = strongly disagree, 5 = strongly agree) were used to measure social media fatigue. Respondents’ responses were totaled and the mean was calculated to measure the extent of social media fatigue. Its Cronbach’s α is 0.872, and three items had CFA factor loading values that were 0.815, 0.882, and 0.806, respectively.
Depressive Symptoms (DS)
Depressive symptoms were measured on a scale from the study by Salokangas et al. [59]. Five 5-point Likert scale items (1 = strongly disagree, 5 = strongly agree) were used. This measurement has been used in prior research [60, 61]. Respondents’ answers were pooled and the mean was calculated to construct the depressive symptoms measure score. Its Cronbach’s α is 0.749, and the CFA factor loadings values for the five items were 0.587, 0.510, 0.704, 0.578, and 0.741.
All the measurements are listed in Appendix A. In addition, the variable measures section above reports their respective structural validity and factor loading values for each question item, indicating that the requirements for further analysis were met. The adjusted CFA model has good fit indicators, where χ2/df is 2.172 (it is generally accepted that less than 5 is acceptable and less than 3 is good); IFI, TLI, and CFI are all greater than 0.9; PGFI, PNFI, and PCFI are greater than 0.7, 0.7, and 0.8, 0.9, respectively; and RMSEA is less than 0.05.
Control Variable
Three kinds of control variables were selected including socio-demographic characteristics, school characteristics, and life status. Specifically, the sociodemographic characteristics variables included gender (Female, Male), age, and university stage (Bachelor’s degree in progress, Master’s degree in progress, Doctorate in progress). School characteristics included school outbreaks (Yes, No), and university type (General University; Key University; Top University). Life state characteristic variables included on-campus accommodation (Yes, No). COVID-19 negative (Yes; No), residence status (Living alone; Others), and social media usage (Never use; <1 h; 1∼3 h; 4∼5 h; 5 h <).
Statistical Analysis
With the help of SPSS26, we did the statistical analysis and common method bias for all variables and Pearson correlation analysis for the main variables. Mediated effects analysis can help the study identify the mechanism of action by which social media overload exerts its influence on depression, as well as the calculation and comparison of total, direct, and indirect effects. In testing the multiple mediating effects, we were conducted using model 6 of the macro PROCESS4.0 tool [62], a tool that helps us to estimate the multiple mediating effects model, as well as the total, direct, and indirect effects of the model [63]. We used 5,000 bootstrap samples randomly selected from the sample to calculate indirect effects and confidence intervals. In particular, it should be noted that in bootstrap analysis, the impact effect of this path is considered significant when the confidence interval does not include 0.
Common Method Bias
Common method bias, which is artificial covariance among measurement variables due to the same data source or respondents, the same measurement environment, the item discourse, and the characteristics of the items themselves, can lead to biased research findings. Harman’s single-factor test is a diagnostic technique for evaluating the severity of common method bias [64]. We conducted Harman’s single-factor test using SPSS26 for the main variables measured. The results showed that the variance of the first single factor was 29.523%, which is below the limit of 40%. This indicates that the quality of the data is adequate for further analysis.
Results
Correlation Analysis Between Main Variables
Bivariate correlations were conducted for the main variables using Pearson correlation, as well as their means and variances were calculated (see Table 1). Positive correlations were found between depressive symptoms, information overload, communication overload, risk perception of COVID-19, and social media fatigue, all of which were statistically significant at the 1% level of significance. Specifically, information overload (r = 0.326, p < 0.01) and communication overload (r = 0.219, p < 0.001) were both positively correlated with depressive symptoms, indicating that college students with higher levels of information overload and communication overload also had higher rates of depressive symptoms during the omicron lockdown. information overload (r = 0.503, p < 0.01) and communication overload (r = 0.206, p < 0.01) were both positively correlated with risk perception of COVID-19, and both information overload (r = 0.366, p < 0.01) and communication overload (r = 0.301, p < 0.01) were also positively correlated with social media fatigue, which indicates that college students with higher levels of social media overload also had higher perceived risk of COVID-19 and social media burnout. In addition, risk perception of COVID-19 and social media fatigue were positively correlated (r = 0.191, p < 0.01), and both risk perception of COVID-19 (r = 0.318, p < 0.01) and social media fatigue (r = 0.357, p < 0.01) were also significantly positively correlated with depressive symptoms, suggesting that college students with higher perceived risk during omicron lockdown also had higher levels of social media fatigue, as well as a higher prevalence of depressive symptoms. The plausibility of the proposed research hypothesis was initially confirmed.
The Chain Mediating Effect of Information Overload and Depressive Symptoms
After controlling for the three types of control variables, we tested the hypotheses using model 6 of SPSS PROCESS 4.0 macro, and the results are shown in Figure 2 and Table 2. Specifically, the “Total effect” in Table 2 indicates that information overload has a positive relationship with depressive symptoms (b = 0.323, CI = 0.259–0.388), which supports hypothesis H1a: Information overload will be positively associated with depressive symptoms.
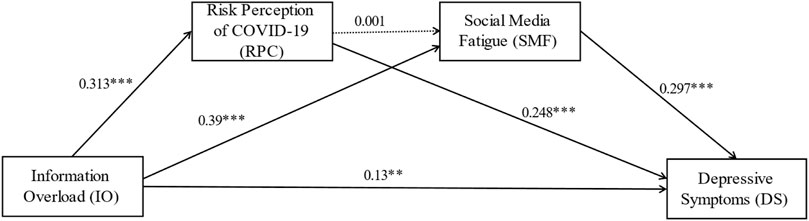
FIGURE 2. The chain mediating effect of information overload and depressive symptoms (Shanghai, China. 2022). Note: Unstandardized path coefficients. The dotted line indicates that the path coefficients are not statistically significant. **p < 0.01; ***p < 0.001.

TABLE 2. Total effect, direct effect, and indirect effect of the multiple mediating effects (Shanghai, China. 2022).
Figure 2 reports the unstandardized path coefficients of the relationship between information overload, risk perception of COVID-19, and social media fatigue and depressive symptoms. Information overload positively influenced risk perception of COVID-19 (b = 0.313, p < 0.001), and also Risk Perception of COVID-19 positively influenced Depressive symptoms (b = 0.248, p < 0.001), which indicated that risk perception of COVID-19 mediated the relationship between information overload and depressive symptoms, and supported hypothesis H2a: Risk Perception of COVID-19 mediates the relationship between information overload and depressive symptoms.
Information Overload positively influenced social media fatigue (b = 0.39, p < 0.001), and at the same time, social media fatigue positively influenced depressive symptoms (b = 0.297, p < 0.001), indicating that social media fatigue mediated the relationship between information overload and depressive symptoms, and supported hypothesis H3a: Social media fatigue (SMF) mediates the relationship between information overload and depressive symptoms. In addition, there is no significant correlation between risk perception of COVID-19 and social media fatigue, suggesting the absence of a chain mediating mechanism. Therefore, hypothesis H4a “Information overload will be positively associated with depressive symptoms through the chain mediating role of risk perception of COVID-19 and social media fatigue” was not supported. In terms of control variables, only gender and social media usage had a significant positive effect on depressive symptoms, while the other control variables were not statistically significant.
After verifying the multiple mediating mechanisms of risk perception of COVID-19 and social media fatigue, we proceeded to calculate the total effect, direct effect, and indirect effect of the two mediating paths (Table 3). Specifically, in the process of information overload positively affecting depressive symptoms, the total effect was 0.323, the direct effect was 0.13, and the indirect effect (0.194) accounted for 60.06% and 149.23% of the total and direct effects, respectively. In other words, the proportion of the indirect effect exceeded the direct effect, and this indirect effect played its effect from two mediated paths (a) CO -> RPC -> DS and (b) CO -> SMF -> DS, which accounted for 24.15% and 35.91% of the total effect, respectively. This indicates that the mediating effect of social media fatigue is stronger than the risk perception of COVID-19. In addition, the above tests for total effect, direct effect, and mediating effects (a) and (b), do not include 0 in the 95% confidence interval and are statistically significant.
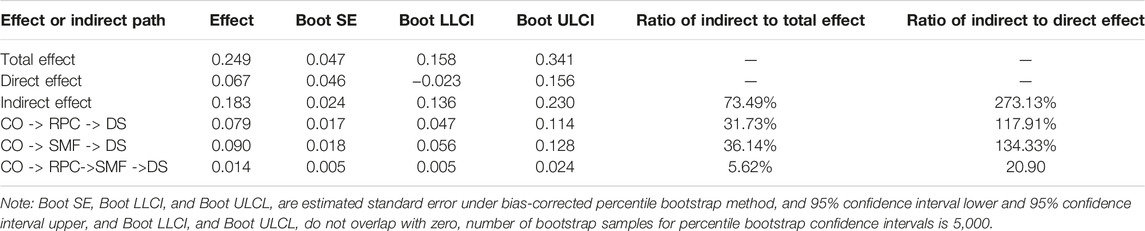
TABLE 3. Total effect, direct effect, and indirect effect of the chain mediating effects (Shanghai, China. 2022).
The Chain Mediating Effect of Communication Overload and Depressive Symptoms
After adding the control variables, we proceeded to test the hypotheses using Model 6 of SPSS PROCESS 4.0 macro, and the results are shown in Figure 3 and Table 3. Table 3 in the “Total effect” section shows that communication overload has a positive relationship with depressive symptoms (b = 0.249, CI = 0.158–0.341), which supports hypothesis H1b: Communication overload will be positively associated with depressive symptoms.
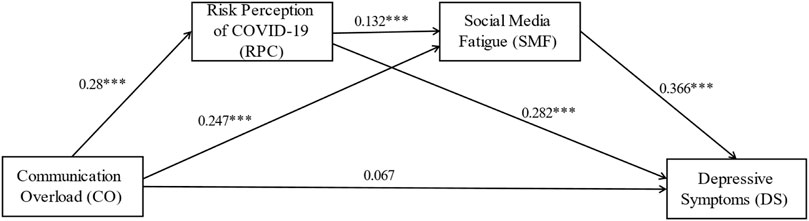
FIGURE 3. The chain mediating effect of communication overload and depressive symptoms (Shanghai, China. 2022). Note: Unstandardized path coefficients. ***p < 0.001.
We reported the unstandardized path coefficients of the relationship between communication overload, risk perception of COVID-19, social media fatigue, and depressive symptoms in Figure 3. Communication overload positively influenced risk perception of COVID-19 (b = 0.28, p < 0.001), and at the same time, risk perception of COVID-19 positively influenced depressive symptoms (b = 0.282, p < 0.001), which indicated that risk perception of COVID-19 mediated the relationship between communication overload and depressive symptoms, and supported hypothesis H2b: Risk perception of COVID-19 mediates the relationship between communication overload and depressive symptoms.
Communication overload positively influenced social media fatigue (b = 0.247, p < 0.001), and meanwhile, social media fatigue positively influenced depressive symptoms (b = 0.366, p < 0.001), suggesting that social media fatigue mediated the relationship between information overload and depressive symptoms, supporting hypothesis H3b: Social media fatigue (SMF) mediates the relationship between communication overload and depressive symptoms. In addition, risk perception of COVID-19 positively influenced social media fatigue (b = 0.132, p < 0.001), and then, risk perception of COVID-19 and social media fatigue mediated the relationship between communication overload and depressive symptoms, respectively. Thus, the existence of the chain mediating mechanism was demonstrated, which subsequently supported hypothesis H4b: Communication overload will be positively associated with depressive symptoms through the chain mediating role of risk perception of COVID-19 and social media fatigue. In addition, only gender and social media usage had a significant positive effect on depressive symptoms; the other control variables were not statistically significant.
The same analysis process as in the above section. Following the verification of the chain mediation mechanism of risk perception of COVID-19 and social media fatigue, we proceeded to calculate the total effect, direct effect, and indirect effect for the three mediated paths (Table 3). Specifically, during the process of communication overload positively affecting depressive symptoms, the total effect was 0.249, the direct effect was 0.067, and the indirect effect (0.183) accounted for 73.49% and 273.13% of the total and direct effects, respectively. It indicates that the proportion of indirect effect surpasses the direct effect, and the indirect effect works from three mediated paths of (a) CO -> RPC -> DS, (b) CO -> SMF -> DS and (c) CO -> RPC -> SMF -> DS, which account for 31.73%, 36.14% and 5.62% of the total effect, correspondingly. It shows that the mediating effect of social media fatigue is stronger than the risk perception of COVID-19 and chain mediating paths, and the chain mediating path has the weakest mediating effect. Furthermore, all of the above tests for total effect, direct effect, and mediated effect (a), (b), and (c), did not include 0 in the 95% confidence interval and were statistically significant.
Discussion
This study examined the association between social media overload and depressive symptoms among university students during the COVID-19 infodemic. The results indicated that information overload and communication overload were positively associated with depressive symptoms, supporting H1a and H1b. The more information and communication people perceive on social media, the higher level of depression they will feel [35]. University students who relied on social media for updates reported being exposed to more contradicting information [65]. An alarming amount of fake information, conspiracy theories, miraculous treatments, and hateful news are circulated during the pandemic [66]. It is difficult to distinguish between true and false, people have to spend time and energy dealing with the overloaded COVID-19-related information and communication, which may lead to depressive symptoms. This finding shows that avoiding social media overload is an effective way to resist infodemic and negative psychological problems.
Risk perception of COVID-19 mediated the relationship between social media overload and depressive symptoms, lending support to hypotheses H2a and H2b. The perception of public risks and mental health can be significantly impacted by social media [28]. The result is consistent with a previous study that perceived vulnerability to COVID-19 mediated the relationship between media exposure and depressive symptoms [67]. Exceeding information and communication about COVID-19 on social media reinforces feelings of risk and higher levels of risk perception increase university students’ depressive symptoms. Imai et al. examined the relationship between risk perception and depression among older adults and found that there was no significant correlation [68]. However, the current study confirmed the association among university students. A possible explanation may be that the affordability of health-related risks increases with age. This finding combines the information mechanism and response mechanism of risk, expanding the social amplification framework of risk. As an amplification station, social media, affects individuals’ risk perception through the processing and dissemination of information, thus forming a response mechanism to psychological health.
Besides, social media fatigue also mediated the relationship between social media overload and depressive symptoms, supporting hypotheses H3a and H3b. It has been confirmed that information overload and communication overload were positively associated with social media fatigue. And there is a positive relationship between social media fatigue and internalizing symptoms such as depression and anxiety [69]. Fatigue was highly associated with psychological factors such as depression and anxiety [70]. In addition, it has also been found that individuals with depressive symptoms are more likely to experience social media fatigue. Being bombed by various kinds of information and communications from social media may lead to fatigue and disrupted task processing, impairing self-regulation and emotional control [71]. This finding provides empirical evidence that social media fatigue may be the antecedent of depressive symptoms. Based on the stressor-strain-outcome (SSO) framework, the present study explored a conceptual research model to show how two different stressors, namely, information overload and communication overload, affect people’s psychological states under the COVID-19 infodemic.
The study yielded interesting findings that risk perception of COVID-19 and social media fatigue played a chain mediating role in the relationship between communication overload and depressive symptoms, lending support to hypothesis H4b. However, this chain-mediated relationship did not make sense between information overload and depressive symptoms, and hypothesis H4a has not been supported. Information overload focuses more on the processing of a huge amount of information that people seek. Pariser proposed the concept of “bubble filters.” The search algorithm pushes personalized information that conforms to the original attitude to individuals through user preferences, which leads to increasingly narrow views on available information, this narrowing creates an invisible filter bubble [72]. The information based on personal preferences (such as the pandemic risk and negative information) affects the level of risk perception. People may turn to social media for further information seeking the effect of the high level of risk perception [73].
Communication overload is concerned with interruptions and frequent unplanned communication that is initiated by a third party [74]. Communication overload is related to both passive and active usage including racking, receiving, and sending [75]. Due to the uncontrollability of communication overload, the risk perception affected by communication overload may lead to social media fatigue. Therefore, risk perception of COVID-19 and social media fatigue played a chain-mediating role in the association between communication overload and depressive symptoms.
Chinese government adopted a zero-COVID strategy and took strict lockdown measures during the COVID-19 [76]. Multiple studies have shown that strict lockdown policies increase people’s psychological burden [76, 77]. The present study provides experimental evidence for the psychological health status of college students in this context. Therefore, in the face of future public health emergencies, protecting people’s mental health issues should become an important consideration for policymakers.
There are some limitations of this study. First, this study is a cross-sectional survey, so that the causative interpretations cannot be determined. Besides, we were unable to establish the necessary temporality to assess the incidence of depressive symptoms and rule out reverse causation. Second, this study relied on self-report scales for the measurement of key variables, which may have resulted in response bias due to errors in respondents’ understanding of scale items, false responses, cognitive level, emotional state, etc. Third, there is still a lack of in-depth exploration on the impact of the type of social media (instant communication tools or short videos), the source of information (We media information or official information), and with whom to communicate (families or peers) on depressive symptoms. Fourth, the current study examines university students during the COVID-19 school lockdown, further exploration is needed to determine whether these findings are applicable to other populations.
Conclusion
This study provides a new perspective for us to understand the impact of social media overload on mental health during public health emergencies. The findings showed that both information overload and communication overload were predictors of depressive symptoms, besides, risk perception of COVID-19 and social media fatigue mediated this relationship. The higher the degree of information overload and communication overload, the stronger people’s risk perception and social media fatigue, thereby increasing the degree of depressive symptoms. We also conducted a chain mediating relationship between communication overload and depressive symptoms. These findings indicate that strict lockdown policies in epidemics can have an impact on the mental health of college students, and the use of social media plays an important predictive role. The present manuscript sparked further thinking on how people should correctly use social media for risk communication under the infodemic. Individuals should reduce the discussion and dissemination of uncertain information during public health emergencies, avoiding social media overload and mental health problems. Besides, the findings provide strong evidence for policy-making in public health emergencies. Future public health policies need to be optimized in the following areas: firstly, for government departments, it’s important to make information available, dispel rumors, and create efficient channels for public feedback. Secondly, social media platforms should establish information filtering mechanisms to provide reliable information during public health emergencies and avoid infodemic. Thirdly, public health policymakers should take more into account individual mental health, especially for vulnerable groups like college students. Measures such as providing psychological counseling services should be taken to alleviate the psychological problems of vulnerable groups in public health emergencies.
Ethics Statement
The studies involving human participants were reviewed and approved by the Ethics Review Committee for Scientific and Technological Research Involving Human Beings of Shanghai Jiao Tong University (H2022200I). The patients/participants provided their written informed consent to participate in this study.
Author Contributions
TX: Framework, Model analyses, Data curation, Writing–original draft. YW: Writing–review and editing, Data curation. YC: Framework, Writing, Data curation. All authors contributed to the article and approved the submitted version.
Conflict of Interest
The authors declare that they do not have any conflicts of interest.
Acknowledgments
The authors are grateful to the participants who participated in this study.
References
1. Cordoș, A-A, and Bolboacă, SD. Lockdown, Social Media Exposure Regarding COVID-19 and the Relation With Self-Assessment Depression and Anxiety. Is the Medical Staff Different? Int J Clin Pract (2021) 75(4):e13933. doi:10.1111/ijcp.13933
2. Schultz, F, Utz, S, and Göritz, A. Is the Medium the Message? Perceptions of and Reactions to Crisis Communication Via Twitter, Blogs and Traditional Media. Public Relations Rev (2011) 37(1):20–7. doi:10.1016/j.pubrev.2010.12.001
3. Nabity-Grover, T, Cheung, CMK, and Thatcher, JB. Inside Out and outside in: How the COVID-19 Pandemic Affects Self-Disclosure on Social Media. Int J Inf Manage (2020) 55:102188. doi:10.1016/j.ijinfomgt.2020.102188
4. Zhao, Y, Cheng, S, Yu, X, and Xu, H. Chinese Public’s Attention to the COVID-19 Epidemic on Social Media: Observational Descriptive Study. J Med Internet Res (2020) 22(5):e18825. doi:10.2196/18825
5. Lee, J, Kim, K, Park, G, and Cha, N. The Role of Online News and Social Media in Preventive Action in Times of Infodemic From a Social Capital Perspective: The Case of the COVID-19 Pandemic in South Korea. Telematics Inform (2021) 64:101691. doi:10.1016/j.tele.2021.101691
6. McCarthy, D, and Saegert, S. Residential Density, Social Overload, and Social Withdrawal. Hum Ecol (1978) 6(3):253–72. doi:10.1007/BF00889026
7. Whelan, E, Najmul Islam, AKM, and Brooks, S. Is Boredom Proneness Related to Social Media Overload and Fatigue? A Stress–Strain–Outcome Approach. Internet Res (2020) 30(3):869–87. doi:10.1108/INTR-03-2019-0112
8. Keles, B, McCrae, N, and Grealish, A. A Systematic Review: The Influence of Social Media on Depression, Anxiety and Psychological Distress in Adolescents. Int J Adolescence Youth (2020) 25(1):79–93. doi:10.1080/02673843.2019.1590851
9. Ettman, CK, Abdalla, SM, Cohen, GH, Sampson, L, Vivier, PM, and Galea, S. Prevalence of Depression Symptoms in US Adults Before and During the COVID-19 Pandemic. Jama Netw Open (2020) 3(9):e2019686. doi:10.1001/jamanetworkopen.2020.19686
10. Zhou, W, Zhang, X, Zheng, Y, Gao, T, Liu, X, and Liang, H. Psychological Impact of COVID-19 Lockdown and Its Evolution: A Case Study Based on Internet Searching Data During the Lockdown of Wuhan 2020 and Shanghai 2022. Healthcare (2023) 11(3):289. Art. 3. doi:10.3390/healthcare11030289
11. Ibrahim, AK, Kelly, SJ, Adams, CE, and Glazebrook, C. A Systematic Review of Studies of Depression Prevalence in University Students. J Psychiatr Res (2013) 47(3):391–400. doi:10.1016/j.jpsychires.2012.11.015
12. Deng, J, Zhou, F, Hou, W, Silver, Z, Wong, CY, Chang, O, et al. The Prevalence of Depressive Symptoms, Anxiety Symptoms and Sleep Disturbance in Higher Education Students During the COVID-19 Pandemic: A Systematic Review and Meta-Analysis. Psychiatry Res (2021) 301:113863. doi:10.1016/j.psychres.2021.113863
13. Kaplan, AM, and Haenlein, M. Users of the World, Unite! the Challenges and Opportunities of Social Media. Business Horizons (2010) 53(1):59–68. doi:10.1016/j.bushor.2009.09.003
14. Lipowski, ZJ. Sensory and Information Inputs Overload: Behavioral Effects. Compr Psychiatry (1975) 16(3):199–221. doi:10.1016/0010-440X(75)90047-4
15. Misra, S, and Stokols, D. Psychological and Health Outcomes of Perceived Information Overload. Environ Behav (2012) 44(6):737–59. doi:10.1177/0013916511404408
16. Sweetser, KD, and Metzgar, E. Communicating During Crisis: Use of Blogs as a Relationship Management Tool. Public Relations Rev (2007) 33(3):340–2. doi:10.1016/j.pubrev.2007.05.016
17. First, JM, Shin, H, Ranjit, YS, and Houston, JB. COVID-19 Stress and Depression: Examining Social Media, Traditional Media, and Interpersonal Communication. J Loss Trauma (2021) 26(2):101–15. doi:10.1080/15325024.2020.1835386
18. Matthes, J, Karsay, K, Schmuck, D, and Stevic, A. “Too Much to Handle”: Impact of Mobile Social Networking Sites on Information Overload, Depressive Symptoms, and Well-Being. Comput Hum Behav (2020) 105:106217. doi:10.1016/j.chb.2019.106217
19. Wang, Q, Luo, X, Tu, R, Xiao, T, and Hu, W. COVID-19 Information Overload and Cyber Aggression During the Pandemic Lockdown: The Mediating Role of Depression/Anxiety and the Moderating Role of Confucian Responsibility Thinking. Int J Environ Res Public Health (2022) 19(3):1540. doi:10.3390/ijerph19031540
20. Chen, W, and Lee, K-H. Sharing, Liking, Commenting, and Distressed? The Pathway Between Facebook Interaction and Psychological Distress. Cyberpsychology, Behav Soc Networking (2013) 16(10):728–34. doi:10.1089/cyber.2012.0272
21. Lee, AR, Son, S-M, and Kim, KK. Information and Communication Technology Overload and Social Networking Service Fatigue: A Stress Perspective. Comput Hum Behav (2016) 55:51–61. doi:10.1016/j.chb.2015.08.011
22. Cheng, X, Fu, S, de Vreede, G-J, Mysore, KS, Udvardi, M, and Wen, J. Understanding Trust Influencing Factors in Social Media Communication: A Qualitative Study. Int J Inf Manage (2017) 37(2):25–37. doi:10.1007/978-1-4939-7003-2_3
23. Reinecke, L, Aufenanger, S, Beutel, ME, Dreier, M, Quiring, O, Stark, B, et al. Digital Stress Over the Life Span: The Effects of Communication Load and Internet Multitasking on Perceived Stress and Psychological Health Impairments in a German Probability Sample. Media Psychol (2017) 20(1):90–115. doi:10.1080/15213269.2015.1121832
25. Kasperson, RE, Renn, O, Slovic, P, Brown, HS, Emel, J, Goble, R, et al. The Social Amplification of Risk: A Conceptual Framework. Risk Anal (1988) 8(2):177–87. doi:10.1111/j.1539-6924.1988.tb01168.x
26. Tsoy, D, Tirasawasdichai, T, and Ivanovich Kurpayanidi, K. Role of Social Media in Shaping Public Risk Perception During COVID-19 Pandemic: A Theoretical Review. THE INTERNATIONAL JOURNAL MANAGEMENT SCIENCE BUSINESS ADMINISTRATION (2021) 7(2):35–41. doi:10.18775/ijmsba.1849-5664-5419.2014.72.1005
27. Honora, A, Wang, K-Y, and Chih, W-H. How Does Information Overload About COVID-19 Vaccines Influence Individuals’ Vaccination Intentions? The Roles of Cyberchondria, Perceived Risk, and Vaccine Skepticism. Comput Hum Behav (2022) 130:107176. doi:10.1016/j.chb.2021.107176
28. Alrasheed, M, Alrasheed, S, and Alqahtani, AS. Impact of Social Media Exposure on Risk Perceptions, Mental Health Outcomes, and Preventive Behaviors During the COVID-19 Pandemic in Saudi Arabia. Saudi J Health Syst Res (2022) 2(3):107–13. doi:10.1159/000525209
29. Hay, J, Coups, E, and Ford, J. Predictors of Perceived Risk for Colon Cancer in a National Probability Sample in the United States. J Health Commun (2006) 11(001):71–92. doi:10.1080/10810730600637376
30. Capone, V, Caso, D, Donizzetti, AR, and Procentese, F. University Student Mental Well-Being During COVID-19 Outbreak: What Are the Relationships Between Information Seeking, Perceived Risk and Personal Resources Related to the Academic Context? Sustainability (2020) 12(17):7039. Art. 17. doi:10.3390/su12177039
31. Dryhurst, S, Schneider, CR, Kerr, J, Freeman, ALJ, Recchia, G, van der Bles, AM, et al. Risk Perceptions of COVID-19 Around the World. J Risk Res (2020) 23(7–8):994–1006. doi:10.1080/13669877.2020.1758193
32. Yan, J, Kim, S, Zhang, SX, Foo, M-D, Alvarez-Risco, A, Del-Aguila-Arcentales, S, et al. Hospitality Workers’ COVID-19 Risk Perception and Depression: A Contingent Model Based on Transactional Theory of Stress Model. Int J Hospitality Manage (2021) 95:102935. doi:10.1016/j.ijhm.2021.102935
33. Zhang, N, Hong, D, Yang, H, Mengxi, G, Huang, X, Wang, A, et al. Risk Perception, Anxiety, and Depression Among Hospital Pharmacists During the COVID-19 Pandemic: The Mediating Effect of Positive and Negative Affect. J Pac Rim Psychol (2022) 16:183449092211016. doi:10.1177/18344909221101670
34. Ding, Y, Xu, J, Huang, S, Li, P, Lu, C, and Xie, S. Risk Perception and Depression in Public Health Crises: Evidence From the COVID-19 Crisis in China. Int J Environ Res Public Health (2020) 17(16):5728. doi:10.3390/ijerph17165728
35. Bright, LF, Kleiser, SB, and Grau, SL. Too Much Facebook? An Exploratory Examination of Social Media Fatigue. Comput Hum Behav (2015) 44:148–55. doi:10.1016/j.chb.2014.11.048
37. Fu, S, Li, H, Liu, Y, Pirkkalainen, H, and Salo, M. Social Media Overload, Exhaustion, and Use Discontinuance: Examining the Effects of Information Overload, System Feature Overload, and Social Overload. Inf Process Manage (2020) 57(6):102307. doi:10.1016/j.ipm.2020.102307
38. Sun, J, and Lee, SK. Flooded With Too Many Messages? Predictors and Consequences of Instant Messaging Fatigue. Inf Tech People (2021) 35:2026–42. ahead-of-print(ahead-of-print). doi:10.1108/ITP-03-2021-0239
39. Sheng, N, Yang, C, Han, L, and Jou, M. Too Much Overload and Concerns: Antecedents of Social Media Fatigue and the Mediating Role of Emotional Exhaustion. Comput Hum Behav (2023) 139:107500. doi:10.1016/j.chb.2022.107500
40. Elci̇yar, K. Overloading in Lockdown: Effects of Social, Information and Communication Overloads in Covid-19 Days. İNİF E - Dergi (2021) 6(1):329–42. doi:10.47107/inifedergi.872896
41. Elmer, T, Mepham, K, and Stadtfeld, C. Students Under Lockdown: Comparisons of Students’ Social Networks and Mental Health Before and During the COVID-19 Crisis in Switzerland. PLOS ONE (2020) 15(7):e0236337. doi:10.1371/journal.pone.0236337
42. Karakose, T, Yirci, R, and Papadakis, S. Examining the Associations Between COVID-19-Related Psychological Distress, Social Media Addiction, COVID-19-Related Burnout, and Depression Among School Principals and Teachers Through Structural Equation Modeling. Int J Environ Res Public Health (2022) 19(4):1951. doi:10.3390/ijerph19041951
43. Pang, H. How Compulsive WeChat Use and Information Overload Affect Social Media Fatigue and Well-Being During the COVID-19 Pandemic? A Stressor-Strain-Outcome Perspective. Telematics Inform (2021) 64:101690. doi:10.1016/j.tele.2021.101690
44. Han, Q, Zheng, B, Agostini, M, Bélanger, JJ, Gützkow, B, Kreienkamp, J, et al. Associations of Risk Perception of COVID-19 With Emotion and Mental Health During the Pandemic. J Affective Disord (2021) 284:247–55. doi:10.1016/j.jad.2021.01.049
45. Zhang, X, Ding, X, Wang, G, and Ma, L. Investigating the Influences of Social Overload and Task Complexity on User Engagement Decrease. Total Qual Manage Business Excell (2020) 31(15–16):1774–87. doi:10.1080/14783363.2018.1509698
46. Scandurra, C, Bochicchio, V, Dolce, P, Valerio, P, Muzii, B, and Maldonato, NM. Why People Were Less Compliant With Public Health Regulations During the Second Wave of the Covid-19 Outbreak: The Role of Trust in Governmental Organizations, Future Anxiety, Fatigue, and Covid-19 Risk Perception. Curr Psychol (2021) 42:7403–13. doi:10.1007/s12144-021-02059-x
47. Wang, B, Zhong, X, Fu, H, Zhang, H, Hu, R, and Chen, C. Risk Perception and Public Pandemic Fatigue: The Role of Perceived Stress and Preventive Coping [Preprint] (2023). In Review. 10.21203/rs.3.rs-2714571/v1.
48. Leiter, MP. Perception of Risk: An Organizational Model of Occupational Risk, Burnout, and Physical Symptoms. Anxiety, Stress, & Coping (2005) 18(2):131–44. doi:10.1080/10615800500082473
49. Wang, Z, Xiao, J, Jiang, F, Li, J, Yi, Y, Min, W, et al. The Willingness of Chinese Adults to Receive the COVID-19 Vaccine and Its Associated Factors at the Early Stage of the Vaccination Programme: A Network Analysis. J Affective Disord (2022) 297:301–8. doi:10.1016/j.jad.2021.10.088
50. Du, M, Tao, L, and Liu, J. Association Between Risk Perception and Influenza Vaccine Hesitancy for Children Among Reproductive Women in China During the COVID-19 Pandemic: A National Online Survey. BMC Public Health (2022) 22(1):385. doi:10.1186/s12889-022-12782-0
51. Wang, Z, Chen, L, Xiao, J, Jiang, F, Min, W, Liu, S, et al. Subjective Health Status: An Easily Available, Independent, Robust and Significant Predictive Factor at the Prometaphase of Vaccination Programs for the Vaccination Behavior of Chinese Adults. BMC Psychiatry (2022) 22(1):180. doi:10.1186/s12888-022-03830-5
52. Wang, J, Lyu, Y, Zhang, H, Jing, R, Lai, X, Feng, H, et al. Willingness to Pay and Financing Preferences for COVID-19 Vaccination in China. Vaccine (2021) 39(14):1968–76. doi:10.1016/j.vaccine.2021.02.060
53. Wang, J, Jing, R, Lai, X, Zhang, H, Lyu, Y, Knoll, MD, et al. Acceptance of COVID-19 Vaccination During the COVID-19 Pandemic in China. Vaccines (2020) 8(3):482. Art. doi:10.3390/vaccines8030482
54. Zhang, S, Zhao, L, Lu, Y, and Yang, J. Do You Get Tired of Socializing? An Empirical Explanation of Discontinuous Usage Behaviour in Social Network Services. Inf Manage (2016) 53(7):904–14. doi:10.1016/j.im.2016.03.006
55. Chung, CJ, and Goldhaber, G. Measuring Communication Load: A Three-Dimensional Instrument. Chicago: meeting of the International Communication Association (1991).
56. Cho, J, Ramgolam, DI, Schaefer, KM, and Sandlin, AN. The Rate and Delay in Overload: An Investigation of Communication Overload and Channel Synchronicity on Identification and Job Satisfaction. J Appl Commun Res (2011) 39(1):38–54. doi:10.1080/00909882.2010.536847
57. Zhuang, Y, Zhao, T, and Shao, X. Mechanism of WeChat’s Impact on Public Risk Perception During COVID-19. Risk Manage Healthc Pol (2021) 14:4223–33. doi:10.2147/RMHP.S328175
58. Maier, C, Laumer, S, Eckhardt, A, and Weitzel, T. Giving Too Much Social Support: Social Overload on Social Networking Sites. Eur J Inf Syst (2015) 24(5):447–64. doi:10.1057/ejis.2014.3
59. Salokangas, RK, Poutanen, O, and Stengård, E. Screening for Depression in Primary Care Development and Validation of the Depression Scale, a Screening Instrument for Depression. Acta Psychiatrica Scand (1995) 92(1):10–6. doi:10.1111/j.1600-0447.1995.tb09536.x
60. Dhir, A, Yossatorn, Y, Kaur, P, and Chen, S. Online Social Media Fatigue and Psychological Wellbeing—A Study of Compulsive Use, Fear of Missing Out, Fatigue, Anxiety and Depression. Int J Inf Manage (2018) 40:141–52. doi:10.1016/j.ijinfomgt.2018.01.012
61. Pradhan, S. Social Network Fatigue: Revisiting the Antecedents and Consequences. Online Inf Rev (2022) 46(6):1115–31. doi:10.1108/OIR-10-2020-0474
62. Hayes, AF. An Index and Test of Linear Moderated Mediation. Multivariate Behav Res (2015) 50(1):1–22. doi:10.1080/00273171.2014.962683
63. Choi, D-H, and Noh, G-Y. Associations Between Social Media Use and Suicidal Ideation in South Korea: Mediating Roles of Social Capital and Self-Esteem. Health Commun (2020) 35(14):1754–61. doi:10.1080/10410236.2019.1663466
64. Podsakoff, PM, MacKenzie, SB, Lee, J-Y, and Podsakoff, NP. Common Method Biases in Behavioral Research: A Critical Review of the Literature and Recommended Remedies. J Appl Psychol (2003) 88(5):879–903. doi:10.1037/0021-9010.88.5.879
65. Jones, NM, Thompson, RR, Dunkel Schetter, C, and Silver, RC. Distress and Rumor Exposure on Social Media During a Campus Lockdown. Proc Natl Acad Sci (2017) 114(44):11663–8. doi:10.1073/pnas.1708518114
66. Rathore, F, and Farooq, F. Information Overload and Infodemic in the COVID-19 Pandemic. J Pakistan Med Assoc (2020) 70:S162-S165–S165. doi:10.5455/JPMA.38
67. Olagoke, AA, Olagoke, OO, and Hughes, AM. Exposure to Coronavirus News on Mainstream Media: The Role of Risk Perceptions and Depression. Br J Health Psychol (2020) 25(4):865–74. doi:10.1111/bjhp.12427
68. Imai, H, Okumiya, K, Fukutomi, E, Wada, T, Ishimoto, Y, Kimura, Y, et al. Association Between Risk Perception, Subjective Knowledge, and Depression in Community-Dwelling Elderly People in Japan. Psychiatry Res (2015) 227(1):27–31. doi:10.1016/j.psychres.2015.03.002
69. Zheng, H, and Ling, R. Drivers of Social Media Fatigue: A Systematic Review. Telematics Inform (2021) 64:101696. doi:10.1016/j.tele.2021.101696
70. Chen, MK. The Epidemiology of Self-Perceived Fatigue Among Adults. Prev Med (1986) 15(1):74–81. doi:10.1016/0091-7435(86)90037-X
71. Vannucci, A, Ohannessian, CM, and Gagnon, S. Use of Multiple Social Media Platforms in Relation to Psychological Functioning in Emerging Adults. Emerging Adulthood (2019) 7(6):501–6. doi:10.1177/2167696818782309
72. Pariser, E. The Filter Bubble: What the Internet Is Hiding From You. Penguin UK: The Penguin Press (2011).
73. Huurne, ET, and Gutteling, J. Information Needs and Risk Perception as Predictors of Risk Information Seeking. J Risk Res (2008) 11(7):847–62. doi:10.1080/13669870701875750
74. Yu, L, Cao, X, Liu, Z, and Wang, J. Excessive Social Media Use at Work: Exploring the Effects of Social Media Overload on Job Performance. Inf Tech People (2018) 31(6):1091–112. doi:10.1108/ITP-10-2016-0237
75. Eliyana, A, Ajija, SR, Sridadi, AR, Setyawati, A, and Emur, AP. Information Overload and Communication Overload on Social Media Exhaustion and Job Performance. Syst Rev Pharm (2020) 11(8):8.
76. Wang, Y, Xu, J, and Xie, T. Social Media Overload and Anxiety Among University Students During the COVID-19 Omicron Wave Lockdown: A Cross-Sectional Study in Shanghai, China, 2022. Int J Public Health (2023) 67:1605363. doi:10.3389/ijph.2022.1605363
77. Hall, BJ, Li, G, Chen, W, Shelley, D, and Tang, W. Prevalence of Depression, Anxiety, and Suicidal Ideation During the Shanghai 2022 Lockdown: A Cross-Sectional Study. J Affective Disord (2023) 330:283–90. doi:10.1016/j.jad.2023.02.121
Appendix A
Keywords: social media overload, depressive symptoms, public health, risk perception, social media fatigue
Citation: Xie T, Wang Y and Cheng Y (2023) Social Media Overload as a Predictor of Depressive Symptoms Under the COVID-19 Infodemic: A Cross-Sectional Survey From Chinese University Students. Int J Public Health 68:1606404. doi: 10.3389/ijph.2023.1606404
Received: 17 July 2023; Accepted: 11 October 2023;
Published: 20 October 2023.
Edited by:
Sonja Merten, Swiss Tropical and Public Health Institute, SwitzerlandReviewed by:
Carla Pires, CBIOS, Universidade Lusófona Research Center for Biosciences and Health Technologies, PortugalPier Luigi Sacco, University of Studies G. d’Annunzio Chieti and Pescara, Italy
Copyright © 2023 Xie, Wang and Cheng. This is an open-access article distributed under the terms of the Creative Commons Attribution License (CC BY). The use, distribution or reproduction in other forums is permitted, provided the original author(s) and the copyright owner(s) are credited and that the original publication in this journal is cited, in accordance with accepted academic practice. No use, distribution or reproduction is permitted which does not comply with these terms.
*Correspondence: Yali Cheng, Y2NjaGVuZ3lhbGlAc2p0dS5lZHUuY24=
This Original Article is Part of the IJPH Special Issue “Digital Democracy and Emergency Preparedness: Engaging the Public in Public Health”