- 1Leibniz Institute for Prevention Research and Epidemiology (BIPS), Bremen, Germany
- 2Institute of Statistics, Faculty of Mathematics and Computer Science, University of Bremen, Bremen, Germany
- 3Department of Public Health and Primary Care, Ghent University, Ghent, Belgium
- 4School of Public Health and Community Medicine, Institute of Medicine, Sahlgrenska Academy, University of Gothenburg, Gothenburg, Sweden
- 5Department of Pediatrics, Medical School, University of Pécs, Pécs, Hungary
- 6GENUD (Growth, Exercise, Nutrition and Development) Research Group, Faculty of Health Sciences, Instituto Agroalimentario de Aragón (IA2), Instituto de Investigación Sanitaria Aragón (IIS Aragón), University of Zaragoza, Zaragoza, Spain
- 7Centro de Investigación Biomédica en Red de Fisiopatología de la Obesidad y Nutrición (CIBERObn), Madrid, Spain
- 8Institute of Food Sciences, National Research Council, Avellino, Italy
- 9Amsterdam University Medical Center, Amsterdam, Netherlands
- 10Department of Social Medicine Public and Occupational Health, Amsterdam Public Health Research Institute, Amsterdam UMC, University of Amsterdam, Amsterdam, Netherlands
- 11Research and Educational Institute of Child Health, Strovolos, Cyprus
- 12Sciensano, Directorate Epidemiology and Public Health, Brussels, Belgium
- 13Estonian Centre of Behavioral and Health Sciences, National Institute for Health Development, Tallinn, Estonia
Objectives: To explore the age-dependent associations between 26 risk factors and BMI in early life, and differences by parental educational level.
Methods: Data of 10,310 children (24,155 measurements) aged 2–16 years participating in a multi-centre European cohort from 2007 to 2014 were utilized. Trajectories of overweight/obesity risk factors and their age-specific associations with BMI were estimated using polynomial mixed-effects models.
Results: Exposure to most unfavourable factors was higher in the low/medium compared to the high education group, e.g., for PC/TV time (12.6 vs. 10.6 h/week). Trajectories of various risk factors markedly changed at an age of 9–11 years. Having a family history of obesity, maternal BMI, pregnancy weight gain and birth weight were positively associated with BMI trajectories throughout childhood/adolescence in both education groups; associations of behavioural factors with BMI were small. Parental unemployment and migrant background were positively associated with BMI in the low/medium education group.
Conclusion: Associations of risk factors with BMI trajectories did not essentially differ by parental education except for social vulnerabilities. The age period of 9–11 years may be a sensitive period for adopting unfavourable behaviours.
Introduction
Overweight/obesity (OW/OB) has reached alarming proportions among children and adolescents, both in high and in low/middle income countries [1]. Childhood OW/OB is linked to adulthood OW/OB, and in turn to a higher risk of chronic diseases including certain types of cancer [2], cardio-metabolic diseases [3] as well as increased mortality and premature death [4]. Several factors have been associated with childhood OW/OB. These can be divided into non-modifiable factors (from a child’s perspective) such as socio-economic status [5], genetic predisposition [6], family structure [7] and pre-, post- and perinatal factors [8, 9] versus modifiable behavioural factors such as sleep [10], diet, sedentary time and physical activity [11]. The persistently high prevalence of OW/OB in children and adolescents in Western societies [1] underlines the pivotal role of risk factors to which the child is not irrevocably exposed to, i.e., modifiable factors.
Recent studies reaffirmed that particularly socio-economically disadvantaged groups such as children of low-educated or unemployed parents or from low-income families are at increased risk of obesity and subsequent metabolic disturbances in Western countries [12–14]. Moreover, unfavourable health-related behaviours were shown to be more prevalent in children of lower educated parents [15]. To date, health interventions have attained only small effects on reducing the risk of OW/OB [16, 17], and particularly vulnerable groups have only been reached to a limited degree [18, 19].
Research into the time windows when OW/OB-related behaviours are shaped, e.g., critical developmental periods such as school entry or start of puberty, is still lacking. Furthermore, periods when non-modifiable factors exert the strongest effects on weight status are hardly known. In this context, impediments to a favourable behaviour change and the role of social vulnerabilities seem to be of pivotal importance. To close this knowledge gap, this study explores 1) trajectories of a large range of OW/OB-related risk factors as well as 2) associations between OW/OB risk factors and body mass index (BMI) development from childhood to adolescence in children of parents with low/medium vs. high educational level based on data of the pan-European IDEFICS (Identification and Prevention of Dietary- and Lifestyle-Induced Health Effects in Children and Infants)/I.Family cohort [20]. Throughout the analyses, we differentiate between factors being modifiable (e.g., diet and physical activity) vs. non-modifiable factors from a child’s perspective (e.g., early life factors like breast feeding duration or vulnerabilities like migrant background).
The results will help to identify the most important risk factors out of a large number of risk factors acting throughout childhood and adolescence and broaden our understanding on potential time windows during which modifiable and non-modifiable OW/OB risk factors exert their most detrimental effects on OW/OB. To the best of our knowledge, this is the first study exploring a large number of risk factor trajectories and their associations with OW/OB based on a European children cohort considering social vulnerabilities.
Methods
Study Population and Data
All analyses are based on the data of the IDEFICS/I. Family cohort, a multi-centre population-based study aiming to investigate the causes of diet- and lifestyle-related diseases in children, adolescents and their families [20, 21]. The baseline survey wave (W0) was conducted in 2007/2008 in eight European countries (Belgium, Cyprus, Estonia, Germany, Hungary, Italy, Spain and Sweden) and included children aged 2.0–9.9 years. In total, 16,229 children fulfilling the inclusion criteria participated. The survey included interviews with parents concerning demographics, family life, health-related behaviours and dietary intakes as well as physical examinations of the children. All measurements were taken using standardized procedures in all eight countries. Additional details on the IDEFICS/I. Family study can be obtained from [20, 21]. A follow-up examination (W1) was conducted in 2009/2010 and the same standardized assessments were applied in 11,043 children who had participated at W0 and in 2,544 newly recruited children. A second follow-up examination (W2) took place in 2013/2014, when 7,118 of the children participating already in W0 or W1 were included. In W2, children aged 12 years or older self-reported their health-related behaviours, wellbeing and family life.
Before children entered the study, parents provided written informed consent. Additionally, all children ≥12 years gave written consent, while younger children gave oral assent in addition to parental consent for the examinations and sample collection. Ethical approval was obtained from the institutional review boards of all eight study centres. The IDEFICS/I. Family cohort is registered under ISRCTN62310987.
Outcome: BMI
Height (cm) was measured to the nearest 0.1 cm with a calibrated stadiometer (Seca 225 stadiometer, Birmingham, United Kingdom), body weight (kg) was measured in fasting state in light underwear on a calibrated scale accurate to 0.1 kg (adapted Tanita BC 420 MA for children ≤6 years, BC 418 MA for children >6 years, Tanita Europe GmbH, Sindelfingen, Germany). BMI was calculated as weight (kg) divided by height (m) squared. BMI measurements were converted to age- and sex-specific z-scores using the extended criteria of the International Obesity Task Force [22].
Educational Level of Parents
Highest educational level of parents was categorized according to the International Standard Classification of Education (ISCED) [23]. The maximum ISCED level of both parents was dichotomized (low/medium = ISCED levels 0, 1, 2, 3, 4, 5 vs. high = ISCED levels 6, 7, 8) and used for stratification in the analyses. In case of single parents, the highest educational level of that parent was used.
Exposures
For our analyses, we selected 13 OW/OB risk factors that are non-modifiable focussing on early life factors and familial/social vulnerabilities: family history of obesity (yes vs. no), maternal BMI (kg/m2), smoking during pregnancy (never/rarely vs. several occasions a week/daily), weight gain during pregnancy (kg), pre-term delivery (yes vs. no), birth weight (1 unit∼100 g), total breast feeding (in months), age at introduction of solid foods (month), migrant status (yes vs. no), number of children in household, being an only child (yes vs. no), one-parent family (yes vs. no), parental unemployment (yes vs. no). We further selected 13 modifiable risk factors focussing on health-related behaviours and wellbeing: major frustrations, e.g., at school (yes vs. no/missing), wellbeing score (ranging from 0 to 48; assessed with the KINDL-R [24, 25]), nocturnal sleep duration (h/night), number of media in bedroom, average PC/TV time (h/week), membership in sports club (yes vs. no), active transport (yes vs. no), as well as dietary intakes of water (times/day), fruits (times/day), vegetables (times/day), sweetened drinks (times/day), savoury fast or snack food (times/day) and simple sugar foods (times/day).
Variables were selected based on previous literature as well as availability of data in the IDEFICS/I. Family cohort and comparability across waves. All exposures as well as covariates used for adjustment (e.g., pubertal status and variables reflecting family life and consumer attitudes) are described in detail in Supplementary Material S1. Supplementary Material S2 depicts a directed acyclic graph (DAG) with the assumed associations among risk factors. The DAG was used to identify confounders and corresponding minimal adjustment sets for our exposures.
Analysis Dataset
In the present analysis, we considered all children that participated in at least two assessment waves of IDEFICS/I.Family. For each child, waves with more than five missing values (out of the considered variables) were excluded from the analysis. The remaining missing values were imputed as detailed in Supplementary Material S3.
The analysis group consisted of 10,310 children in total, of which 6,775 had participated in two and 3,535 in three examination waves (9,532 at W0, 9,487 at W1 and 5,136 at W3). A flow chart depicting the selection process leading to this analysis group is shown in Figure 1.
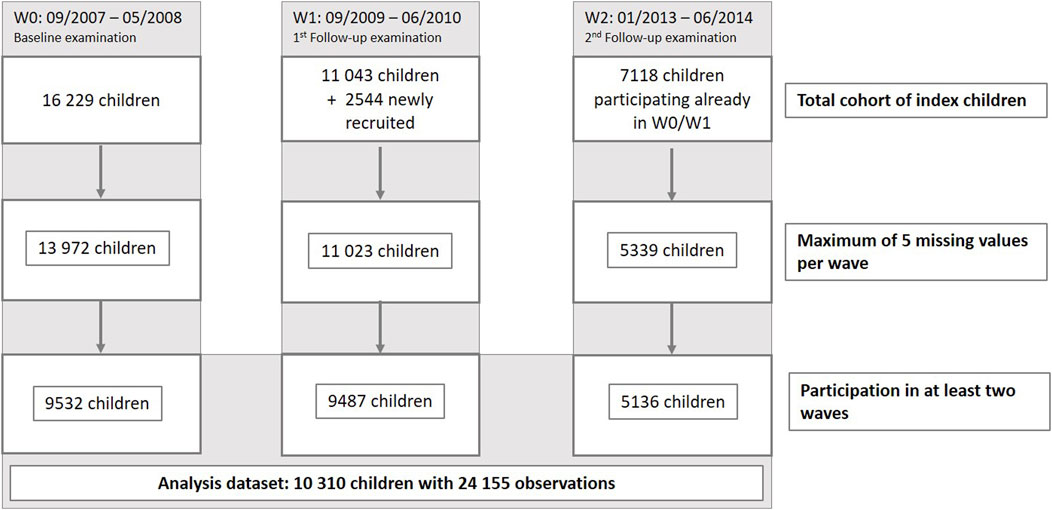
FIGURE 1. Flow chart depicting the selection process leading to the final study sample (IDEFICS/I.Family study, eight European countries, 2007–2014).
Estimation of Age-Dependent Changes (Trajectories) of OW/OB Risk Factors
Polynomial linear mixed-effects models [26] with a random intercept and random slope and three powers of age were used to assess the development of continuous risk factors from infancy to adolescence; analogously logistic mixed models including a random intercept and three powers of age were used to estimate the evolution of binary risk factors. All risk factor models were adjusted for sex and region of residence (North/Central Europe, Southern Europe, Eastern Europe) and estimated stratified by low/medium vs. high educational level of the parents (classified according to the International Standard Classification of Education; ISCED, 2011 [23]). Least square means (also called marginal means) were calculated as a linear combination (sum) of the estimated effects from our polynomial mixed-effects models (using the LSMEANS statement in SAS Proc MIXED). Least square means were estimated at selected ages to plot the risk factor trajectories and compare the risk factor changes in boys and girls from 3 to 15 years of age. A common limitation of polynomial models is that the effect estimates at the lower and upper end of the covered age range are less stable. For this reason, we only report estimates from 3 to 15 years of age (while our models were estimated based on data covering the age span from 2 to 16 years) and estimates for the ages 3 and 15 should be interpreted with caution.
Estimation of Age-Dependent Associations Between Risk Factors and BMI
Polynomial linear mixed models with two levels (measurement occasion and individual) and three powers of age were used to assess the age-dependent associations between the modifiable and non-modifiable risk factors and BMI [27]. An unstructured covariance matrix was chosen for modelling the covariance of the random effects. Continuous co-variables were centred before model fitting to obtain meaningful model estimates.
For each exposure of interest, a separate model was fitted including interaction terms of that exposure with age, as well as confounders. All models included sex and geographic region and corresponding interactions with age. Further confounders were selected for each exposure based on the DAG depicted in Supplementary Material S2. Supplementary Material S4 provides a table listing all exposures and corresponding adjustment sets.
Effect estimates from polynomial mixed-effects models are in general difficult to interpret. In order to directly obtain estimates for the associations of the risk factors with BMI at selected ages (3, 6, 9, 12, and 15 years), all models were reparametrized by centring the age variables at ages 3, 6, 9, 12, and 15 years, respectively.
Least square means were calculated to plot and compare BMI trajectories of children with different exposure levels (e.g., of children that sleep 8 vs. 10 h/night) while standardizing over all included confounders.
All analyses were performed stratified by highest educational level of the parents (low/medium vs. high). The group of children with low parental ISCED level was too small to be regarded separately.
Analyses were performed using SAS® statistical software version 9.4 (SAS Institute, Inc., Cary, NC, United States). Proc MI was used for the multiple imputation and Proc MIXED for the linear mixed-effects models. Effect estimates for the multiple imputed datasets were combined using Proc MIANALYZE and 99% confidence intervals (99% CI) were estimated to account at least partially for multiple testing. Effects were considered as statistically significant if the confidence interval does not include the null value.
Results
Baseline characteristics are displayed in Table 1 stratified by low/medium vs. high educational level of parents. The mean age at baseline was 6.0 years (SD: 1.8 years) and the sexes were almost balanced (50.7% males, 49.3% females). The percentage of children with a migration background (14.6% vs. 11.4%), living with a single parent (17.7% vs. 9.1%) as well as the percentage being an only child (22.6% vs. 16.8%) were higher in the low/medium compared to the high education group. While the mean total breastfeeding duration (6.1 months vs. 7.7 months) and percentage of children being member in a sports club (41.2% vs. 52.1%) was lower in the low/medium education group, the mean number of media in the bedroom (1.1 vs. 0.5 media), average PC/TV time (12.6 vs. 10.6 h/week) and consumption frequency of sweetened drinks (2.1 vs. 1.6 times/day) were higher in the low/medium vs. high education group.
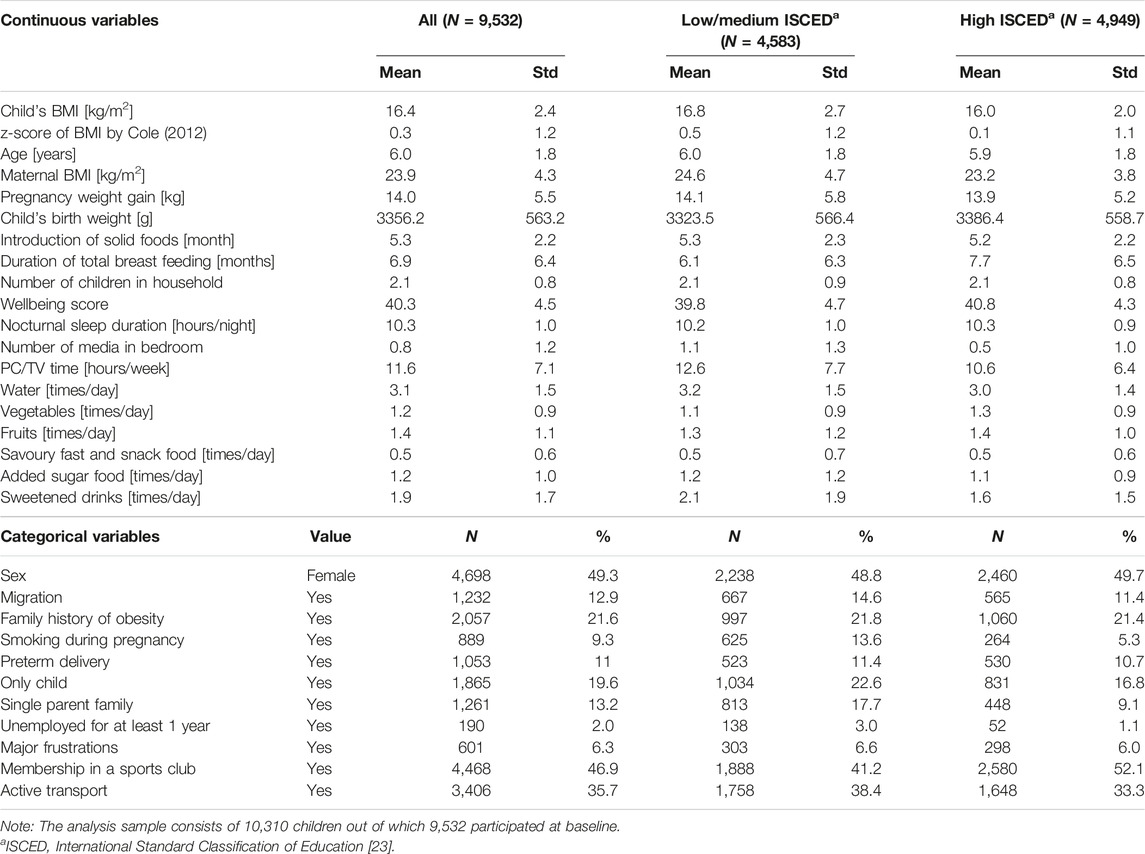
TABLE 1. Baseline (W0) characteristics: means and standard deviation of continuous variables and numbers and percentages of categorical variables for the total baseline sample as well as stratified by educational level of parents. (IDEFICS/IFamily study, eight European countries, 2007–2014).
Trajectories of Risk Factors
Figure 2 displays the trajectories of selected modifiable risk factors from 3 to 15 years of age stratified by sex and educational level; corresponding numbers and confidence intervals as well as the graphical displays of the trajectories of all remaining time-varying risk factors are shown in Supplementary Material S5. Risk factor trajectories differed by parental educational attainment and sex (see Figure 2 and Supplementary Material S5). From the age of 4 years, sports club membership was higher in boys and among children with high parental educational level. The average PC/TV time continuously increased until adolescence (e.g., from 8.8 to 29.3 h/week in boys in the low/medium education group) and was generally higher among boys and in the low/medium education group. While the consumption of savoury snacks and fast food increased in boys and girls from the low/medium education group after the age of 9, it slightly declined among girls in the high education group. The consumption of sweetened drinks was higher in the low/medium education group and among boys across all ages. However, it increased in all groups after the age of 9 years. Conversely, water consumption decreased in boys with low/medium parental education after the age of 9 years.
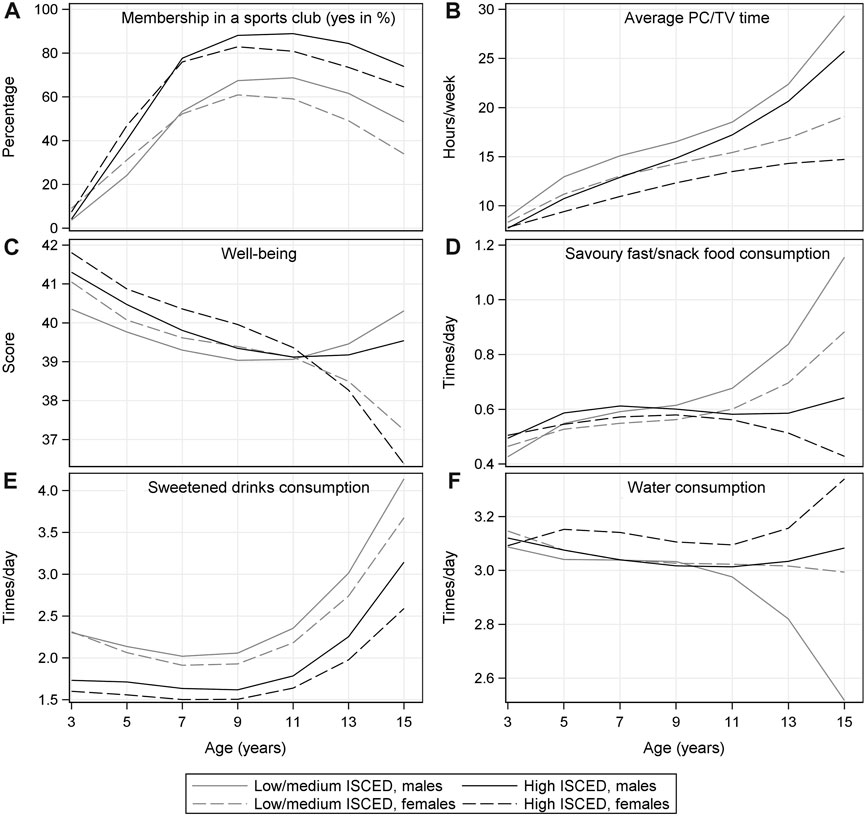
FIGURE 2. Trajectories of selected risk factors from 3 to 15 years of age by sex and educational level of parents (low/medium vs. high ISCED level); marginal means estimated based on the fractional polynomial mixed effects models (IDEFICS/I.Family study, eight European countries, 2007–2014).
The slope of the trajectories changed for many risk factors at the ages of 9–11 years, e.g., the consumption frequency of savoury snacks/fast food and sweetened drinks increased; the mean wellbeing score increased in boys while it decreased in girls. The proportion of children with a sports club membership peaked at 89% at the age of 10–11 years and declined thereafter.
Age-Specific Associations of Risk Factors With BMI
Non-Modifiable Risk Factors
Table 2 depicts the effect estimates and 99% CIs for the associations between the 13 non-modifiable risk factors and BMI at ages 3, 6, 9, 12 and 15 years stratified by parental educational level. With respect to the early life factors, associations with BMI were mainly similar comparing children with low/medium vs. high parental education. For instance, maternal weight gain during pregnancy and birth weight were positively associated with BMI in both education groups from 3 years of age onwards. Also, the family history of obesity and maternal BMI were found to be positively associated with the BMI of the child from age 6 onwards in both education groups with the strengths of the associations increasing with age. For the family history of obesity, effect estimates were larger in the low/medium (increasing from β = 0.71 at age 6 to β = 1.50 at age 15) compared to the high education group (increasing from β = 0.32 at age 6 to β = 0.83 at age 15). Smoking during pregnancy revealed a positive association with BMI particularly at age 15 in the low/medium education group (β = 1.40 [0.43; 2.37]; 99% CI in brackets).
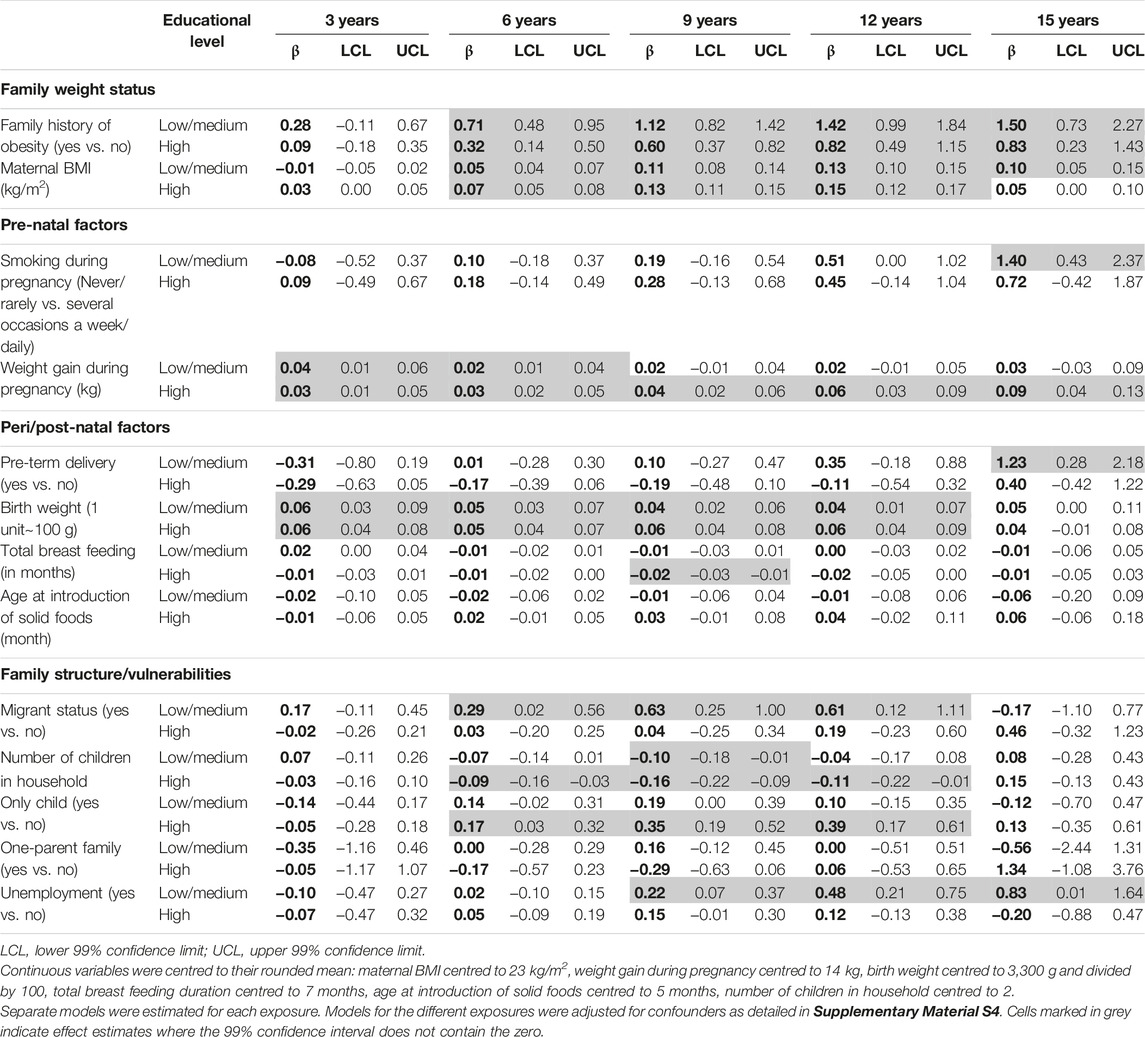
TABLE 2. Effect estimates (β coefficients; printed in bold) and 99% confidence intervals obtained from polynomial linear mixed models for the associations between non-modifiable risk factors (from a child’s perspective) and BMI at the age of 3, 6, 9, 12 and 15 (IDEFICS/I.Family study, eight European countries, 2007–2014).
Differences between the low/medium vs. high parental education groups were further observed with regard to family structure and vulnerabilities: migrant status (up to age 12) and unemployment (ages 9–15) showed positive associations with BMI in the low/medium education group only. Being an only child was associated with a higher BMI in the high education group, but effect estimates pointed to the same direction in the low/medium education group. A larger number of children in the household was associated with a lower BMI in both education groups.
Modifiable Risk Factors
Associations of wellbeing and the behavioural risk factors with BMI were in general small and mainly appeared beyond the age of 9 years (Table 3). Again, associations with BMI were similar comparing the two education groups, except for some of the dietary variables. In both education groups, longer sleep duration, membership in a sports club and active transport were associated with a lower BMI at age 9 and/or 12 years. The number of media in the bedroom was positively associated with BMI at ages 6 and/or 9 and PC/TV time from ages 9 to 15. Regarding the dietary variables, some effect estimates changed direction between age 9 and 15. For example, water consumption was positively associated with BMI at age 9 and negatively associated at age 15 while added sugar food consumption and savoury snack/fast food (low/medium education group only) showed a negative association at age 9 and a positive association at age 15. For vegetable consumption we observed a negative association with BMI in the high education group from ages 9 to 12.
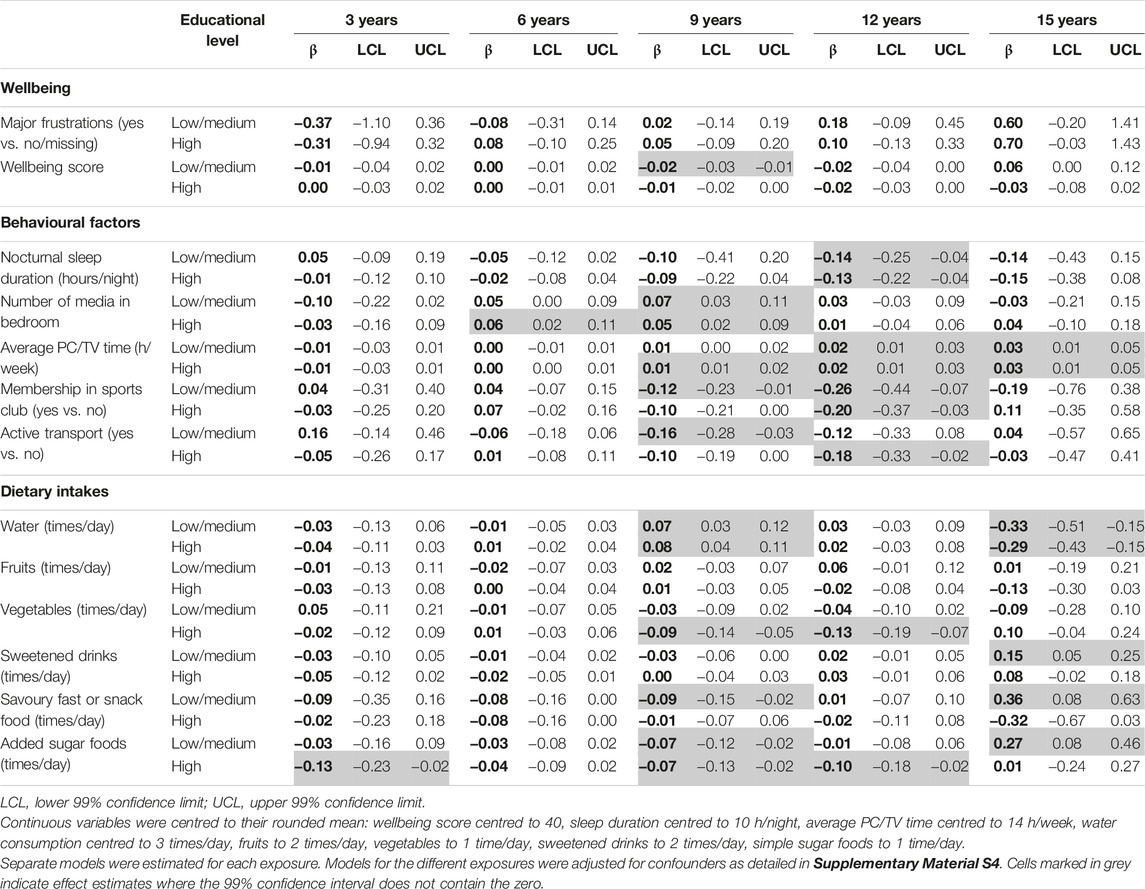
TABLE 3. Effect estimates (β coefficients; printed in bold) and 99% confidence intervals obtained from polynomial linear mixed models for the associations between modifiable risk factors and body mass index (BMI) at the age of 3, 6, 9, 12 and 15 years (IDEFICS/I.Family study, eight European countries, 2007–2014).
BMI Trajectories at Selected Exposure Levels
Figure 3 and Supplementary Material S6 compare BMI trajectories of children at certain fixed exposure levels (e.g., 8 h vs. 10 h nocturnal sleep duration) while standardizing over all covariates. In general, the BMI trajectories were higher in the low/medium vs. high education group. Large differences appeared when comparing children with vs. without family history of obesity (see Figures 3A, B) whereas for most behavioural factors the observed differences were rather small within the same education group (see, e.g., Figures 3C, D for sleep duration, and Supplementary Material S6 for all other exposures).
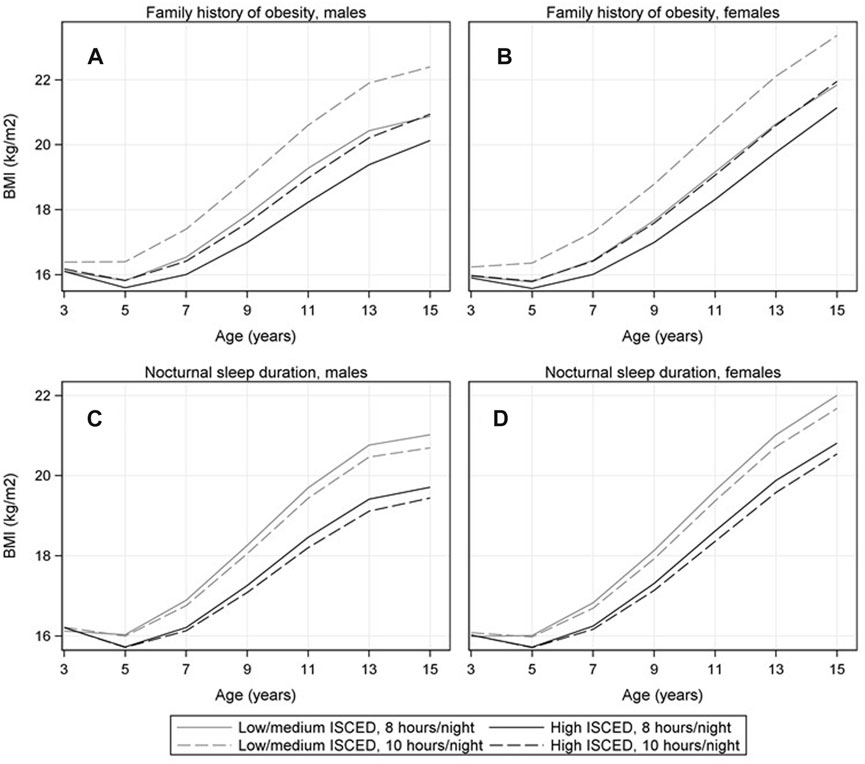
FIGURE 3. Mean BMI trajectories comparing children with vs. without family history of obesity [panel (A) in males, panel (B) in females] and BMI trajectories comparing children that sleep 8 vs. 10 h/night [panel (C) in males; panel (D) in females] stratified by educational level of parents (low/medium vs. high ISCED level). The numbers represent the marginal means estimated based on the confounder-adjusted fractional polynomial mixed effects models, i.e., the mean values at fixed exposure levels were standardized over covariates (IDEFICS/I.Family study, eight European countries, 2007–2014).
Discussion
We studied a wide range of risk factors for OW/OB during the early life course as well as differences by educational level of parents. Our results confirm that large differences exist concerning the age-specific prevalence of risk factors during childhood/adolescence comparing children of parents with a low/medium vs. high educational level. Further differences exist between boys and girls. The higher prevalence of OW/OB risk factors in children with low/medium parental education is well documented in previous literature [28] and is likely to explain the higher OW/OB prevalence in that group. Nevertheless, the associations of most risk factors with BMI are similar among children of both education groups. This suggests that a risk factor, if present, will have its detrimental effect on BMI independently of the parental educational level.
Non-Modifiable Risk Factors
Our data confirm the effects of well-established risk factors acting early in life, e.g., for high pregnancy weight gain [29], high birth weight, and high maternal pre-pregnancy BMI [30, 31], and further demonstrate the persistence of these effects into adolescence. For example, our data reveal maternal weight gain during pregnancy and maternal BMI to be associated with an increased BMI even 15 years after birth.
Modifiable Risk Factors
In line with previous studies, membership in a sports club and active transport [32] as proxies for physical activity were negatively associated with BMI while higher screen time [33] as proxy for sedentary behaviour was positively associated with BMI. These associations were observed from the age of 9–12 years onwards and independent of the parental educational level. Moreover, sleep duration turned out to be a protective factor for BMI, supporting previous studies [34]. A recent study that did not observe effects of behavioural factors on adiposity in children up to the age of 5 years suspected that young children were less likely to, e.g., consume fast food or engage in PA [35]. Consequently, the lower variability in such health behaviours may make it more difficult to detect effects on adiposity in young children. This may explain why associations of behavioural factors with BMI were mainly observed in later childhood/adolescence in our study.
Some of the effect estimates of the dietary variables changed their direction at approximately 12 years of age which corresponds to the age when we switched from parental reports to self-reports. For fruit and vegetable consumption, parent and child reports were previously shown to differ at the individual, but not at the group level [36]. Consequently, the authors of that study concluded that parent and child reports can be used in determinant studies. Nevertheless, we cannot preclude that our findings may be partially explained by differences in social desirability bias and differential reporting behaviour in parents and teens [37, 38]. In addition, dietary data are particularly prone to misreporting [37] which may be another reason for the partly unexpected findings for water, added sugar foods and savoury fast/snack foods.
Beyond that, our estimated risk factor trajectories indeed point to the age period of 9–11 years being a potential turning point for many health behaviours. Certain risk factors such as average PC/TV time, consumption frequency of sweetened drinks and savoury fast and snack foods increased after the age of 10. This may be explained by increased peer influence and stronger autonomy of children getting older. A recent paper by [39] revealed that the peer resemblance of health behaviours surpasses the sibling resemblance by the age of 9–10 years. Moreover, use of digital media increases with age. This may lead to a higher exposure to advertisements for unhealthy foods, which in turn is associated with less healthy food and beverage intake [40, 41].
Sex-Specific Differences in Risk Factor Trajectories
A sports club membership was more common in boys than in girls in our study, which has been previously observed across European countries [42]. One reason may be an insufficient variety of activities that suits girl’s preferences [42]. Boys also spent more time with PC/TV than girls which was also reported for primary school children across Europe. Different cultural values regarding sedentary behaviours of boys and girls may be responsible for this difference which in turn influence parental support [42]. Again in agreement with other studies, the consumption of sweetened drinks was higher among boys than girls [43–45] which may partly be explained by the higher screen time of boys [40, 45]. In our study, at the ages of 9–11 years, the mean wellbeing score increased in boys while it decreased in girls. A study in 11 to 15 years-old German adolescents also reported lower life satisfaction and self-related health in girls than in boys. Furthermore, girls reported psychosomatic health complaints more often than boys with the frequency increasing with age [46]. Potential reasons for this gap may be sex-specific differences in pubertal development, e.g., physical changes and the development of self-identity as well as different coping strategies for increased school stress of boys and girls [46].
Social Vulnerability and Family Structure
Previous studies showed social vulnerabilities such as unemployment [47] and having a migration background [48] to be risk factors for childhood OW/OB. We found unemployment and migration background to be associated with a higher BMI particularly in children with low/medium parental education. It has been suggested that the socio-economic position of parents may exacerbate or buffer the effect social vulnerabilities may have on different health-related behaviours and stress [28, 48]. Unemployment affects not only the economic resources but reduces social integration and life satisfaction. A migration background can result in language barriers and a lack of acceptance by people of the new country which are stressors for migrant children [48]. Furthermore, risk factors for OW/OB like unfavourable dietary patterns, shorter sleep duration [49] and sedentary behaviours have been reported to be more prevalent among children with a migration background [50]. Nevertheless, the OW/OB risk can vary depending on the reasons of migration, the similarity with the host country and the language spoken at home [48] which may be causes that could explain why the associations are stronger in the low/medium ISCED group. Further social vulnerabilities which have previously been shown to increase the risk of childhood OW/OB include living in a single parent family [51] or being an only child [52]. In our study only the latter was found to be associated with the children’s BMI and this association was stronger in the high compared to the low/medium education group. In line with the literature [53] we found a higher number of children in the household to decrease the risk for childhood OW/OB. This association was not observed at the age of 3 years when siblings may not have been born yet for many children and not at the age of 15 years when siblings may have already moved out or become less important because of a higher degree of independence.
In general, associations between the single behavioural factors and BMI were rather small. Nevertheless, multi-factorial interventions addressing several modifiable factors may still have a large impact on BMI development as confirmed by previous meta-analyses [16, 54].
Limitations and Strengths
We acknowledge several limitations: Although information on the type of occupation and family income would have been available, we focussed on differences by parental educational level in our study. Employment status was previously shown to be less related to childhood OW/OB [5] and reported income is known to be more prone to misreporting and typically contains many missing values (as also in our data). In addition, educational level may not only reflect the socio-economic status but is further a marker for health literacy [55] which is likely to be linked to many modifiable OW/OB risk factors [56]. Highly educated parents and their children were more likely to participate in our cohort, i.e., selection towards a healthier, well-educated population is likely [57].
All exposure variables except for maternal BMI at W2 were based on self- or proxy-reports and may hence be affected by recall and social-desirability bias where the latter may even differ between self-reports vs. parental reports. We further used rather simple indicators to reflect dietary intakes of foods considered healthy/unhealthy to ease interpretation, but these cannot capture the complexity of the diet as a whole. The use of polynomial models with three powers of age is still a compromise between model complexity, adequate model fit and interpretability. As we modelled mean BMI trajectories starting after an age of 2 years and hence with only one local minimum (adiposity rebound) we considered models with three powers of age to provide sufficient flexibility [26].
Strengths of our study include the highly standardized and quality-controlled assessment procedures, the large multi-centre study with a prospective design, the use of a DAG for confounder selection and the sophisticated statistical methods used to estimate the exposure trajectories and their associations with BMI covering the early life course from infancy to adolescence. Our study is unique in that it considered a large number of known and suspected OW/OB risk factors simultaneously while adjusting for a comprehensive set of confounders. The overall credibility of our results is supported by the fact that associations with most well-established risk factors for OW/OB are replicated by our analysis.
Conclusion
The age-specific prevalence of many OW/OB risk factors largely differs by educational level of parents, especially with view to dietary variables. At approximately 9–11 years of age certain risk factors like the consumption of sweetened drinks and savoury fast and snack foods markedly increase. This may be explained by increased peer influence and stronger autonomy as well as by higher exposure to advertisements for unhealthy foods as children grow older. Sex-specific differences were found for PC/TV use that increases much stronger in boys than in girls and for wellbeing that decreases in girls while it increases in boys beyond the age of 11 years. Associations of risk factors with BMI trajectories did not essentially differ by parental educational level except for social vulnerabilities like migration status and unemployment which seem to exert more detrimental effects in children of parents with low/medium education. BMI trajectories also differed between children with vs. without a family history of obesity, while modifiable behavioural factors showed mainly small associations with BMI. Although the single effects may appear small, multi-factorial interventions addressing several modifiable factors may still have a large impact on BMI development.
Ethics Statement
The studies involving humans were approved by the appropriate institutional review boards of all eight study centres (1. Belgium: Ethics Committee of the Gent University Hospital, 15 October 2007, ref: No. EC UZG 2007/243 and 19 February 2013, No. B670201316342; 2. Cyprus: Cyprus National Bioethics Committee, 12 July 2007, ref: No. EEBK/EM/2007/16 and 21 February 2013, No. EEBK/ETI/2012/33; 3. Estonia: Tallinn Medical Research Ethics Committee (TMREC), 14 June 2007, ref: No. 1093 and 17 January 2013, No. 128; 4. Germany: Ethic Commission of the University of Bremen, 16 January 2007 and 11 December 2012; 5. Hungary: Medical Research Council, 21 June 2007, ref: 22-156/2007-1018EKU and 18 December 2012, 4536/2013/EKU; 6. Italy: Ethics Committee of the Local Health Authority (ASL) in Avellino, 19 June 2007, ref: No. 2/CE and 18 September 2012, No. 12/12; 7. Spain: Ethics Committee for Clinical Research of Aragon (CEICA), 20 June 2007, ref: No. PI07/13 and 13 February 2013, No. PI13/0012; 8. Sweden: Regional Ethics Research Board in Gothenburg, 30 July 2007, ref: No. 264-07 and 10 January 2013, No. 927-12). The studies were conducted in accordance with the local legislation and institutional requirements. Written informed consent for participation in this study was provided by the participants’ legal guardians/next of kin.
Author Contributions
CB, MW, and WA conceived and designed the study. CB performed the analysis. CB, MW, and ES interpreted the data and drafted the manuscript. CB, MW, ES, WA, AS, KW, SV, MH, and TVr made substantial contributions to the analysis and the interpretation of the data. WA, SD, MH, DM, LM, PR, MT, and TVe contributed data. All authors contributed to the article and approved the submitted version.
Funding
The GrowH! project is funded by the Joint Programming Initiative “A Healthy Diet for a Healthy Life” (JPI HDHL), a research and innovation initiative of EU member states and associated countries. The funding agencies supporting this work are (in alphabetical order of participating countries): Belgium: Research Foundation—Flanders (FWO); Germany: Federal Ministry of Education and Research (BMBF; grant no. 01EA2102A); Spain: Spanish State Research Agency (AEI); Netherlands: Netherlands Organisation for Health Research and Development (ZonMw). This project has received funding from the European Union’s Horizon 2020 research and innovation programme under the ERA-NET Cofund action N° 727565. The data have been made available for this publication by the IDEFICS (http://www.idefics.eu) and I.Family studies (http://www.ifamilystudy.eu/). The IDEFICS Study was funded by the European Community within the Sixth RTD Framework Programme Contract No. 016181 (FOOD). The I.Family Study was funded by the European Community within the Seventh RTD Framework Programme Contract No. 266044.
Conflict of Interest
The authors declare that they do not have any conflicts of interest.
Acknowledgments
This work was done based on the data of the IDEFICS study and the I.Family Study (http://www.ifamilystudy.eu/). We are grateful for the support of school boards, head teachers and communities. The authors wish to thank the IDEFICS children and their parents for participating in this extensive examination.
Supplementary Material
The Supplementary Material for this article can be found online at: https://www.ssph-journal.org/articles/10.3389/ijph.2023.1605798/full#supplementary-material
References
1. Abarca-Gómez, L, Abdeen, ZA, and Hamid, ZA. Worldwide Trends in Body-Mass Index, Underweight, Overweight, and Obesity From 1975 to 2016: A Pooled Analysis of 2416 Population-Based Measurement Studies in 128.9 Million Children, Adolescents, and Adults. The Lancet (2017) 390:2627–42. doi:10.1016/S0140-6736(17)32129-3
2. Basen-Engquist, K, and Chang, M. Obesity and Cancer Risk: Recent Review and Evidence. Curr Oncol Rep (2011) 13:71–6. doi:10.1007/s11912-010-0139-7
3. Baker, JL, Olsen, LW, and Sørensen, TIA. Childhood Body-Mass Index and the Risk of Coronary Heart Disease in Adulthood. New Engl J Med (2007) 357:2329–37. doi:10.1056/NEJMoa072515
4. Franks, PW, Hanson, RL, Knowler, WC, Sievers, ML, Bennett, PH, and Looker, HC. Childhood Obesity, Other Cardiovascular Risk Factors, and Premature Death. New Engl J Med (2010) 362:485–93. doi:10.1056/NEJMoa0904130
5. Buoncristiano, M, Williams, J, Simmonds, P, Nurk, E, Ahrens, W, Nardone, P, et al. Socioeconomic Inequalities in Overweight and Obesity Among 6- to 9-Year-Old Children in 24 Countries From the World Health Organization European Region. Obes Rev (2021) 22(6):e13213. doi:10.1111/obr.13213
6. Loos, RJF, and Bouchard, C. FTO: The First Gene Contributing to Common Forms of Human Obesity. Obes Rev (2008) 9:246–50. doi:10.1111/j.1467-789X.2008.00481.x
7. Santiago, S, Zazpe, I, Cuervo, M, and Martínez, JA. Perinatal and Parental Determinants of Childhood Overweight in 6-12 Years Old Children. Nutr Hosp (2012) 27:599–605. doi:10.1590/S0212-16112012000200037
8. Rito, AI, Buoncristiano, M, Spinelli, A, Salanave, B, Kunešová, M, Hejgaard, T, et al. Association Between Characteristics at Birth, Breastfeeding and Obesity in 22 Countries: The WHO European Childhood Obesity Surveillance Initiative - COSI 2015/2017. Obes Facts (2019) 12:226–43. doi:10.1159/000500425
9. Weng, SF, Redsell, SA, Swift, JA, Yang, M, and Glazebrook, CP. Systematic Review and Meta-Analyses of Risk Factors for Childhood Overweight Identifiable During Infancy. Arch Dis Child (2012) 97:1019–26. doi:10.1136/archdischild-2012-302263
10. Deng, X, He, M, He, D, Zhu, Y, Zhang, Z, and Niu, W. Sleep Duration and Obesity in Children and Adolescents: Evidence From an Updated and Dose-Response Meta-Analysis. Sleep Med (2021) 78:169–81. doi:10.1016/j.sleep.2020.12.027
11. Lee, EY, and Yoon, K-H. Epidemic Obesity in Children and Adolescents: Risk Factors and Prevention. Front Med (2018) 12:658–66. doi:10.1007/s11684-018-0640-1
12. Iguacel, I, Michels, N, Ahrens, W, Bammann, K, Eiben, G, Fernández-Alvira, JM, et al. Prospective Associations Between Socioeconomically Disadvantaged Groups and Metabolic Syndrome Risk in European Children. Results From the IDEFICS Study. Int J Cardiol (2018) 272:333–40. doi:10.1016/j.ijcard.2018.07.053
13. Iguacel, I, Börnhorst, C, Michels, N, Breidenassel, C, Dallongeville, J, González-Gross, M, et al. Socioeconomically Disadvantaged Groups and Metabolic Syndrome in European Adolescents: The HELENA Study. J Adolesc Health (2021) 68:146–54. doi:10.1016/j.jadohealth.2020.05.027
14. Iguacel, I, Fernández-Alvira, JM, Ahrens, W, Bammann, K, Gwozdz, W, Lissner, L, et al. Prospective Associations Between Social Vulnerabilities and Children's Weight Status. Results From the IDEFICS Study. Int J Obes (Lond) (2018) 42:1691–703. doi:10.1038/s41366-018-0199-6
15. Brug, J, van Stralen, MM, Te Velde, SJ, Chinapaw, MJM, De Bourdeaudhuij, I, Lien, N, et al. Differences in Weight Status and Energy-Balance Related Behaviors Among Schoolchildren Across Europe: The ENERGY-Project. PLoS One (2012) 7:e34742. doi:10.1371/journal.pone.0034742
16. Brown, T, Moore, TH, Hooper, L, Gao, Y, Zayegh, A, Ijaz, S, et al. Interventions for Preventing Obesity in Children. Cochrane Database Syst Rev (2019) 7:Cd001871. doi:10.1002/14651858.CD001871.pub4
17. Ells, LJ, Rees, K, Brown, T, Mead, E, Al-Khudairy, L, Azevedo, L, et al. Interventions for Treating Children and Adolescents With Overweight and Obesity: An Overview of Cochrane Reviews. Int J Obes (Lond) (2018) 42:1823–33. doi:10.1038/s41366-018-0230-y
18. Bürgi, F, Niederer, I, Schindler, C, Bodenmann, P, Marques-Vidal, P, Kriemler, S, et al. Effect of a Lifestyle Intervention on Adiposity and Fitness in Socially Disadvantaged Subgroups of Preschoolers: A Cluster-Randomized Trial (Ballabeina). Prev Med (2012) 54:335–40. doi:10.1016/j.ypmed.2012.02.007
19. Plachta-Danielzik, S, Pust, S, Asbeck, I, Czerwinski-Mast, M, Langnäse, K, Fischer, C, et al. Four-Year Follow-Up of School-Based Intervention on Overweight Children: The KOPS Study. Silver Spring) (2007) 15:3159–69. doi:10.1038/oby.2007.376
20. Ahrens, W, Siani, A, Adan, R, De Henauw, S, Eiben, G, Gwozdz, W, et al. Cohort Profile: The Transition From Childhood to Adolescence in European Children-How I.Family Extends the IDEFICS Cohort. Int J Epidemiol (2017) 46:1394–5j. doi:10.1093/ije/dyw317
21. Ahrens, W, Bammann, K, De Henauw, S, Halford, J, Palou, A, Pigeot, I, et al. Understanding and Preventing Childhood Obesity and Related Disorders--IDEFICS: A European Multilevel Epidemiological Approach. Nutr Metab Cardiovasc Dis (2006) 16:302–8. doi:10.1016/j.numecd.2006.01.011
22. Cole, TJ, and Lobstein, T. Extended International (IOTF) Body Mass Index Cut-Offs for Thinness, Overweight and Obesity. Pediatr Obes (2012) 7:284–94. doi:10.1111/j.2047-6310.2012.00064.x
23. United Nations Educational Scientific and Cultural Organization (UNESCO). International Standard Classification of Education, ISCED 2011. Canada: UNESCO Institute for Statistics (2012).
24. Ravens-Sieberer, U, and Bullinger, M. Kindl-R English Questionnaire for Measuring Health-Related Quality of Life in Children and Adolescents. Revised Version Manual (2000). Ulrike Ravens-Sieberer & Monika Bullinger. Available from: https://www.kindl.org/english/questionnaires/implementation-manual/.
25. Bullinger, M, Brutt, AL, Erhart, M, and Ravens-Sieberer, U, BELLA Study Group. Psychometric Properties of the KINDL-R Questionnaire: Results of the BELLA Study. Eur Child Adolesc Psychiatry (2008) 17(1):125–32. doi:10.1007/s00787-008-1014-z
26. Tilling, K, Macdonald-Wallis, C, Lawlor, DA, Hughes, RA, and Howe, LD. Modelling Childhood Growth Using Fractional Polynomials and Linear Splines. Ann Nutr Metab (2014) 65:129–38. doi:10.1159/000362695
27. Wen, X, Kleinman, K, Gillman, MW, Rifas-Shiman, SL, and Taveras, EM. Childhood Body Mass Index Trajectories: Modeling, Characterizing, Pairwise Correlations and Socio-Demographic Predictors of Trajectory Characteristics. BMC Med Res Methodol (2012) 12:38. doi:10.1186/1471-2288-12-38
28. Ayala-Marín, AM, Iguacel, I, Miguel-Etayo, P, and Moreno, LA. Consideration of Social Disadvantages for Understanding and Preventing Obesity in Children. Front Public Health (2020) 8:423. doi:10.3389/fpubh.2020.00423
29. Ziauddeen, N, Huang, JY, Taylor, E, Roderick, PJ, Godfrey, KM, and Alwan, NA. Interpregnancy Weight Gain and Childhood Obesity: Analysis of a UK Population-Based Cohort. Int J Obes (Lond) (2022) 46:211–9. doi:10.1038/s41366-021-00979-z
30. Larque, E, Labayen, I, Flodmark, CE, Lissau, I, Czernin, S, Moreno, LA, et al. From Conception to Infancy - Early Risk Factors for Childhood Obesity. Nat Rev Endocrinol (2019) 15:456–78. doi:10.1038/s41574-019-0219-1
31. Leonard, SA, Rasmussen, KM, King, JC, and Abrams, B. Trajectories of Maternal Weight From Before Pregnancy Through Postpartum and Associations With Childhood Obesity. Am J Clin Nutr (2017) 106:1295–301. doi:10.3945/ajcn.117.158683
32. Laverty, AA, Hone, T, Goodman, A, Kelly, Y, and Millett, C. Associations of Active Travel With Adiposity Among Children and Socioeconomic Differentials: A Longitudinal Study. BMJ Open (2021) 11:e036041. doi:10.1136/bmjopen-2019-036041
33. Tahir, MJ, Willett, W, and Forman, MR. The Association of Television Viewing in Childhood With Overweight and Obesity Throughout the Life Course. Am J Epidemiol (2019) 188:282–93. doi:10.1093/aje/kwy236
34. Snell, EK, Adam, EK, and Duncan, GJ. Sleep and the Body Mass Index and Overweight Status of Children and Adolescents. Child Develop (2007) 78:309–23. doi:10.1111/j.1467-8624.2007.00999.x
35. Nianogo, RA, Wang, MC, Wang, A, Nobari, TZ, Crespi, CM, Whaley, SE, et al. Projecting the Impact of Hypothetical Early Life Interventions on Adiposity in Children Living in Low-Income Households. Pediatr Obes (2017) 12:398–405. doi:10.1111/ijpo.12157
36. Reinaerts, E, de Nooijer, J, and de Vries, NK. Parental Versus Child Reporting of Fruit and Vegetable Consumption. Int J Behav Nutr Phys Activity (2007) 4:33. doi:10.1186/1479-5868-4-33
37. Börnhorst, C, Huybrechts, I, Ahrens, W, Eiben, G, Michels, N, Pala, V, et al. Prevalence and Determinants of Misreporting Among European Children in Proxy-Reported 24 H Dietary Recalls. Br J Nutr (2013) 109:1257–65. doi:10.1017/S0007114512003194
38. Forrestal, SG. Energy Intake Misreporting Among Children and Adolescents: A Literature Review. Matern Child Nutr (2011) 7:112–27. doi:10.1111/j.1740-8709.2010.00270.x
39. Bogl, LH, Mehlig, K, Ahrens, W, Gwozdz, W, de Henauw, S, Molnár, D, et al. Like Me, Like You - Relative Importance of Peers and Siblings on Children's Fast Food Consumption and Screen Time But Not Sports Club Participation Depends on Age. Int J Behav Nutr Phys Act (2020) 17:50. doi:10.1186/s12966-020-00953-4
40. Olafsdottir, S, Berg, C, Eiben, G, Lanfer, A, Reisch, L, Ahrens, W, et al. Young Children's Screen Activities, Sweet Drink Consumption and Anthropometry: Results From a Prospective European Study. Eur J Clin Nutr (2014) 68:223–8. doi:10.1038/ejcn.2013.234
41. Lissner, L, Lanfer, A, Gwozdz, W, Olafsdottir, S, Eiben, G, Moreno, LA, et al. Television Habits in Relation to Overweight, Diet and Taste Preferences in European Children: The IDEFICS Study. J Epidemiol (2012) 27:705–15. doi:10.1007/s10654-012-9718-2
42. Whiting, S, Buoncristiano, M, Gelius, P, Abu-Omar, K, Pattison, M, Hyska, J, et al. Physical Activity, Screen Time, and Sleep Duration of Children Aged 6-9 Years in 25 Countries: An Analysis Within the WHO European Childhood Obesity Surveillance Initiative (COSI) 2015-2017. Obes Facts (2021) 14:32–44. doi:10.1159/000511263
43. Duffey, KJ, Huybrechts, I, Mouratidou, T, Libuda, L, Kersting, M, De Vriendt, T, et al. Beverage Consumption Among European Adolescents in the HELENA Study. Eur J Clin Nutr (2012) 66:244–52. doi:10.1038/ejcn.2011.166
44. Marshall, TA, Curtis, AM, Cavanaugh, JE, Warren, JJ, and Levy, SM. Child and Adolescent Sugar-Sweetened Beverage Intakes Are Longitudinally Associated With Higher Body Mass Index Z Scores in a Birth Cohort Followed 17 Years. J Acad Nutr Diet (2019) 119:425–34. doi:10.1016/j.jand.2018.11.003
45. Russo, RG, Northridge, ME, Wu, B, and Yi, SS. Characterizing Sugar-Sweetened Beverage Consumption for US Children and Adolescents by Race/Ethnicity. J Racial Ethn Health Disparities (2020) 7:1100–16. doi:10.1007/s40615-020-00733-7
46. Kaman, A, Ottová-Jordan, V, Bilz, L, Sudeck, G, Moor, I, and Ravens-Sieberer, U. Subjective Health and Well-Being of Children and Adolescents in Germany - Cross-Sectional Results of the 2017/18 HBSC Study. J Health Monit (2020) 5:7–20. doi:10.25646/6899
47. Briody, J. Parental Unemployment During the Great Recession and Childhood Adiposity. Soc Sci Med (2021) 275:113798. doi:10.1016/j.socscimed.2021.113798
48. Iguacel, I, Gasch-Gallén, Á, Ayala-Marín, AM, De Miguel-Etayo, P, and Moreno, LA. Social Vulnerabilities as Risk Factor of Childhood Obesity Development and Their Role in Prevention Programs. Int J Obes (Lond) (2021) 45:1–11. doi:10.1038/s41366-020-00697-y
49. Labree, W, van de Mheen, D, Rutten, F, Rodenburg, G, Koopmans, G, and Foets, M. Differences in Overweight and Obesity Among Children From Migrant and Native Origin: The Role of Physical Activity, Dietary Intake, and Sleep Duration. PLOS ONE (2015) 10:e0123672. doi:10.1371/journal.pone.0123672
50. Hilpert, M, Brockmeier, K, Dordel, S, Koch, B, Weiß, V, Ferrari, N, et al. Sociocultural Influence on Obesity and Lifestyle in Children: A Study of Daily Activities, Leisure Time Behavior, Motor Skills, and Weight Status. Obes Facts (2017) 10:168–78. doi:10.1159/000464105
51. Hankey, MS, and Miyazaki, Y. Multilevel Analysis of BMI Growth Trajectories of US School Children: Features and Risk Factors. SSM Popul Health (2019) 8:100455. doi:10.1016/j.ssmph.2019.100455
52. Chen, AY, and Escarce, JJ. Family Structure and Childhood Obesity: An Analysis Through 8th Grade. Matern Child Health J (2014) 18:1772–7. doi:10.1007/s10995-013-1422-7
53. Datar, A. The More the Heavier? Family Size and Childhood Obesity in the U.S. Soc Sci Med (2017) 180:143–51. doi:10.1016/j.socscimed.2017.03.035
54. Jacob, CM, Hardy-Johnson, PL, Inskip, HM, Morris, T, Parsons, CM, Barrett, M, et al. A Systematic Review and Meta-Analysis of School-Based Interventions With Health Education to Reduce Body Mass Index in Adolescents Aged 10 to 19 Years. Int J Behav Nutr Phys Act (2021) 18:1. doi:10.1186/s12966-020-01065-9
55. Svendsen, MT, Bak, CK, Sørensen, K, Pelikan, J, Riddersholm, SJ, Skals, RK, et al. Associations of Health Literacy With Socioeconomic Position, Health Risk Behavior, and Health Status: A Large National Population-Based Survey Among Danish Adults. BMC Public Health (2020) 20:565. doi:10.1186/s12889-020-08498-8
56. de Buhr, E, and Tannen, A. Parental Health Literacy and Health Knowledge, Behaviours and Outcomes in Children: A Cross-Sectional Survey. BMC Public Health (2020) 20:1096. doi:10.1186/s12889-020-08881-5
Keywords: body mass index, childhood overweight/obesity, IDEFICS/I.Family cohort, overweight/obesity risk factors, parental education
Citation: Börnhorst C, Ahrens W, De Henauw S, Hunsberger M, Molnár D, Moreno LA, Russo P, Schreuder A, Sina E, Tornaritis M, Vandevijvere S, Veidebaum T, Vrijkotte T, Wijnant K and Wolters M (2023) Age-Specific Quantification of Overweight/Obesity Risk Factors From Infancy to Adolescence and Differences by Educational Level of Parents. Int J Public Health 68:1605798. doi: 10.3389/ijph.2023.1605798
Received: 19 January 2023; Accepted: 26 October 2023;
Published: 15 November 2023.
Edited by:
Mariachiara Di Cesare, University of Essex, United KingdomReviewed by:
Joanna Mazur, University of Zielona Góra, PolandSara JalaliFarahani, University of Essex, United Kingdom
Copyright © 2023 Börnhorst, Ahrens, De Henauw, Hunsberger, Molnár, Moreno, Russo, Schreuder, Sina, Tornaritis, Vandevijvere, Veidebaum, Vrijkotte, Wijnant and Wolters. This is an open-access article distributed under the terms of the Creative Commons Attribution License (CC BY). The use, distribution or reproduction in other forums is permitted, provided the original author(s) and the copyright owner(s) are credited and that the original publication in this journal is cited, in accordance with accepted academic practice. No use, distribution or reproduction is permitted which does not comply with these terms.
*Correspondence: Claudia Börnhorst, Ym9lcm5AbGVpYm5pei1iaXBzLmRl