- 1Guizhou Centre for Disease Control and Prevention, Guiyang, China
- 2Department of Biostatistics, School of Public Health, Fudan University, Shanghai, China
- 3Key Laboratory of Public Health and Safety of the Ministry of Education, Fudan University, Shanghai, China
- 4Key Lab of Health Technology Assessment, Ministry of Health, Fudan University, Shanghai, China
- 5Department of Epidemiology, School of Public Health, Fudan University, Shanghai, China
Objectives: Studies have linked obesity to an increased risk of hypertension, but the optimal adiposity indicators to predict hypertension remains controversial. We comprehensively explored the correlation between body mass index, waist circumference (WC), waist-to-height ratio (WHtR), long-term weight and WC change, and hypertension in an adult population in Southwest China.
Methods: We studied 9,280 participants from 48 townships of 12 districts with a follow-up of 10 years in the Guizhou Population Health Cohort Study. We used Pearson’s correlation coefficients combined with Dunn and Clark’s z test and Zou’s confidence interval test, receiver operating characteristic (ROC) analyses, and multivariate Cox proportional hazards regressions adjusting for demographic characteristics, lifestyle habits, disease history, and lipid information of participants.
Results: Baseline central adiposity indicators (WC and WHtR) had closer associations with hypertension than BMI, and long-term WC change was more predictive of hypertension compared with weight change in the studied population.
Conclusion: Central adiposity indicators maintain a stronger association with the risk of hypertension, hinting at the importance of WC management in the precaution of hypertension.
Introduction
Hypertension is a major cause of premature death worldwide [1]. The high prevalence and burden of hypertension, which affects the health and living qualities of 1.13 billion people worldwide [2], is a serious concern [3–5]. Two-thirds of adults aged 30–79 years with hypertension worldwide live in low- and middle-income countries, mainly due to increased risk factors in those populations in recent decades [1–3, 6]. Reducing modifiable risk factors, including being overweight or obese, is among the best ways to prevent hypertension and related diseases and organ damage, and avoiding dietary and behavioral risk factors is doubly essential for those with unmodifiable or hereditary risk factors [7].
Evidence has demonstrated the association between obesity and an increased risk of hypertension [8–12]. Data from NHANES indicated that obesity is an important predictor of elevated blood pressure [8]. Although the association between hypertension and obesity has been confirmed, the best anthropometric index of obesity that predicts or associates strongly with hypertension remains controversial. Some studies have observed that waist circumference (WC) maintained a stronger association with hypertension than body mass index (BMI) [13–15], while other studies found that BMI best predicted hypertension [16, 17]. Additionally, the Korean Genome and Epidemiology Study indicated that the central obesity indices like WC and waist-height ratio (WHtR) were better than BMI for the prediction of hypertension in middle-aged Korean people [18]. And there is evidence indicating WHtR had the best performance for discriminating hypertension [19–21]. Besides, a meta-analysis done exclusively on the Caucasian population concluded that compared to WHtR, WC was more associated with hypertension [13]. Moreover, few studies assessed the predictive abilities of long-term weight or WC change for hypertension. It is necessary to compare the effects of long-term weight or WC change on hypertension as it accumulated over time.
Therefore, the main objective of this study was to compare the association of commonly used baseline adiposity indicators (BMI, WC, and WHtR) and long-term adiposity changes (weight and WC change from baseline to follow-up) with hypertension in an adult population in Southwest China, and to comprehensively assess the predictive power of adiposity indicators for hypertension.
Methods
Study Design and Population
The Guizhou Population Health Cohort Study (GPHCS) is a community-based prospective cohort study in Guizhou Province, China. A total of 9,280 adult residents in 48 townships of 12 districts in Guizhou Province were recruited from 2010 to 2012 using the multi-stage proportional stratified cluster sampling method. Inclusion criteria were [1]: 18 years of age or older [2]; living in the study area with no plans to move out [3]; completing the survey questionnaire and taking blood samples; and [4] signing a written informed consent. This study was approved by the Institutional Review Board of the Guizhou Provincial Center for Disease Control and Prevention (No. S2017-02). All subjects provided written informed consent at the time of enrollment into the cohort.
All participants were followed up through repeat surveys between 2016 and 2020 to collect information on major chronic conditions and vital status. All deaths were confirmed through the Death Registration Information System and the Basic Public Health Services System. In our analysis, we excluded 1) subjects who had a history of hypertension at baseline (n = 2,132), 2) subjects who were lost to follow-up (n = 1,117), 3) subjects with missing hypertension data at follow-up (n = 406), and 4) subjects with missing height or weight information at baseline (n = 12). Finally, a total of 5,613 participants were included in our analysis.
Anthropometric Indicators of Obesity
In this study, we investigate anthropometric indices of obesity including body mass index (BMI), waist circumference (WC), waist-height ratio (WHtR), weight change, and WC change. Participants’ standing height, body weight, and WC were measured by trained investigators, both at baseline and follow-up. Standing height was measured using a portable stadiometer to the nearest 0.1 cm and body weight was measured using digital weight scales to the nearest 0.1 kg. WC was measured at the midpoint between the lowest rib margin and the iliac crest to the nearest 0.1 cm. BMI was calculated as body weight (kg) divided by the square of height (m2). BMI was evaluated using either per standard deviation (SD) increase or divided into four categories (low normal weight <22.0; high normal weight 22.0–23.9; overweight 24.0–27.9; and obese ≥28.0 kg/m2) according to the Chinese BMI classification criteria [22]. WC was evaluated using either per SD increase or divided into two categories (normal WC < 85 cm for women and <90 cm for men; central obesity ≥85 cm for women and ≥90 cm for men) [23]. WHtR was calculated as WC (cm) divided by height (cm) and evaluated by either per SD increase or divided into two categories (low WHtR <0.5 and high WHtR ≥0.5) [24]. The amount of weight change in participants was calculated by subtracting the weight recorded at baseline from the weight recorded at the follow-up minus and evaluated by either per SD increase or divided into four categories according to its distribution (loss of >2 kg, loss of ≤2 to gain of <2 kg, gain of ≥2 to gain of <6 kg, and gain of ≥6 kg). The amount of WC change in participants was calculated by subtracting the WC recorded at baseline from the WC recorded at the follow-up minus and evaluated by either per SD increase or divided into four categories according to its distribution (loss of >3 cm, loss of ≤3 to gain of <3 cm, gain of ≥3 to gain of <9 cm, and gain of ≥9 cm).
Hypertension
Participants were diagnosed with hypertension if they met either of the following two criteria [1]: self-reported diagnosis of hypertension or anti-hypertensive treatment by physicians; or [2] systolic blood pressure (SBP) ≥140 mmHg and/or diastolic blood pressure (DBP) ≥90 mmHg [25]. The blood pressure was measured with the same type of electronic sphygmomanometer and was accurate at 0.1 mmHg. If the difference between the three measurements did not exceed 10 mmHg, the average of the three measurements was taken as the final reading; if the difference between the three measurements was large, the average of the two similar measurements was taken as the final reading; if only one measurement was taken, the final reading was taken directly.
Covariates
All participants were interviewed face-to-face and completed a structured questionnaire that provided information relating to participants’ demographic characteristics (sex, age, area, ethnicity, marital status, and occupation), lifestyle habits (smoking status, alcohol use, and exercise), and history of diabetes. Participants were diagnosed with Type 2 diabetes mellitus (T2DM) if they met any of the following criteria [1]: self-reported diagnosis of diabetes or anti-diabetic treatment by physicians [2]; fasting plasma glucose (FPG) of ≥7.0 mmol/L [3]; oral glucose tolerance test (OGTT) result of ≥11.1 mmol/L; or [4] hemoglobin A1c (HbA1c) of ≥6.5% [26]. Venous blood samples were collected after an overnight fast of at least 8 h to measure total cholesterol (TC), triglycerides (TG), high-density lipoprotein cholesterol (HDL-C), and low-density lipoprotein cholesterol (LDL-C).
Statistical Analysis
Continuous variables were represented by mean (SD) and categorical variables by percentages. The Student’s t-test for continuous variables and the Chi-square test for categorical variables were done to compare the differences between new hypertension cases and non-hypertension subjects. The follow-up person-years (PYs) were calculated from the date of enrollment in the cohort to the date of hypertension diagnosis, death, or follow-up, whichever occurred first.
Associations between anthropometric measures and hypertension were first measured by Pearson’s correlation coefficients and the corresponding 95% confidence intervals. Pearson’s correlation coefficients were classified into four categories: very strong (0.90–1.00), strong (0.70–0.89), moderate (0.40–0.69), weak (0.10–0.39), and negligible (0–0.10) [27], to present the strength of the association. To control for the possibility of an observed difference between two correlations occurring simply by chance, a test of significance is necessary. Hittner, May, and Silver’s modification of Dunn and Clark’s z test and Zou’s confidence interval test were utilized to compare two overlapping correlations in dependent groups [28].
Receiver Operating Characteristic (ROC) analyses were then used to compare differences in the prediction of hypertension across anthropometric measures [29–31]. The area under the ROC curve (AUC) and the corresponding 95% confidence intervals for half of the follow-up person-years were determined by the time-dependent ROC curve. In addition, time-AUC curves of various anthropometric measures were utilized to compare the AUC at each time point.
Finally, multivariate Cox proportional hazards regression model was performed to estimate the association between hypertension and baseline general adiposity indicator (BMI), central adiposity indicators (WC and WHtR), and long-term adiposity changes (weight and WC change from baseline to follow-up). We fitted three separate models of the association between each obesity indicator and hypertension and tested the anthropometric measures individually in separate Cox models [1]: Model 1: adjusted for age (as continuous) and sex [2]; Model 2: Model 1 plus area (urban, rural), ethnicity (ethnic minorities, the Han nationality), marriage (married, unmarried, other), occupation (farmer, unemployed, and retired, other), smoking status (current smoker, non-current smoker), alcohol use (yes or no), exercise (yes or no), and history of diabetes (yes or no) [3]; Model 3: Model 2 plus SBP, total cholesterol, triglycerides, HDL-C value, LDL-C value, and baseline BMI value (in the analyses of weight change and WC change). The adjusted hazard ratios (HRs) were presented with 95% confidence intervals (CIs). The proportional hazards assumption in Cox regression models was tested using Schoenfeld residuals, and no evidence of non-proportionality was observed. In addition, we fitted multivariate Cox models with restricted cubic splines with 4 knots at the 5th, 35th, 65th, and 95th percentiles to test for linearity in the association between anthropometric indices of obesity and incident hypertension and found no evidence of nonlinearity (Supplementary Table S1). We also calculated the C-index to evaluate the model performance of these Cox models adjusted for the covariates described above.
The robustness of our findings was assessed in multiple sensitivity analyses. First, we re-estimated the association between obesity indicators and the risk of hypertension after excluding the participants who followed up in less than 1 year. Second, we calculated E-values to explore the possibility of unmeasured confounding between obesity indicators and hypertension risk [32, 33]. The E-value quantifies the required magnitude of unmeasurable confounders that may negate the currently obtained correlation between obesity indicators and the risk of hypertension.
All analyses were two-tailed and p < 0.05 was considered to indicate statistical significance. All analyses were conducted in R software (version 4.1.0).
Results
Baseline Characteristics
The basic characteristics of the study population are presented in Supplementary Table S2. A total of 5,613 participants were included in the study, and 1,214 (21.6%) were diagnosed with hypertension at follow-up. The mean age of the study population was 42.02 ± 14.17 years and most were women. Participants were more likely to be married, to be farmers, to be exercise population, and had a lower proportion of ethnic minority. Only 6.3% of subjects had a history of diabetes, and a small number of subjects were current drinkers and smokers. Compared with participants who remained free of hypertension, new hypertension cases were older and had a significantly higher mean BMI, WC, WHtR, SBP, and TG.
In addition, we also found that of the 9,280 participants, 1,117 (12.04%) were lost to follow-up. Compared to the complete follow-up group, subjects lost to follow-up were older at baseline and had statistically significant differences in mean BMI, SBP, TC, and LDL. The detailed results of the comparison were shown in Supplementary Table S3.
Correlations Coefficients
The Pearson’s correlation coefficients between the various anthropometric measures with blood pressure are displayed in Figure 1 and Supplementary Table S4. Baseline BMI, WC, and WHtR were positively and weakly correlated with blood pressure (p < 0.001), among which WC correlated with blood pressure most closely (both SBP and DBP were considered). Weight change and WC change from baseline to follow-up were negatively correlated with blood pressure (p < 0.001). Compared with WC change, Weight change had a higher but negligible correlation with SBP and a lower correlation with DBP. We further compared these associations and found that the correlation between DBP and WC was significantly higher than the other two associations, and WC change had a significantly larger negative effect on DBP compared with weight change. Moreover, the correlation with blood pressure (both SBP and DBP) was significantly higher for WC than for long-term adiposity changes (weight change and WC change from baseline to follow-up). The detailed results of the association comparison were listed in Table 1.
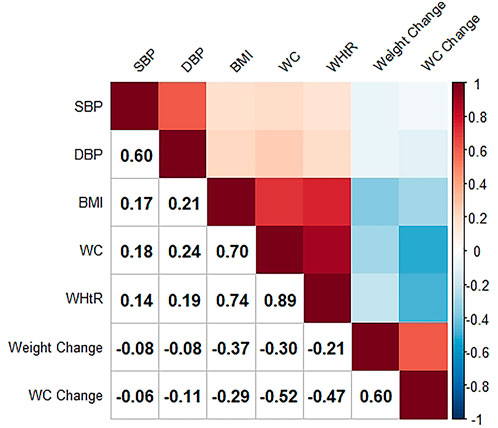
FIGURE 1. Intercorrelations between anthropometric indices and blood pressure (The Guizhou population health cohort study, China, 2010–2012). SBP, systolic blood pressure; DBP, diastolic blood pressure; BMI, body mass index; WC, waist circumference; WHtR, waist-to-height ratio.
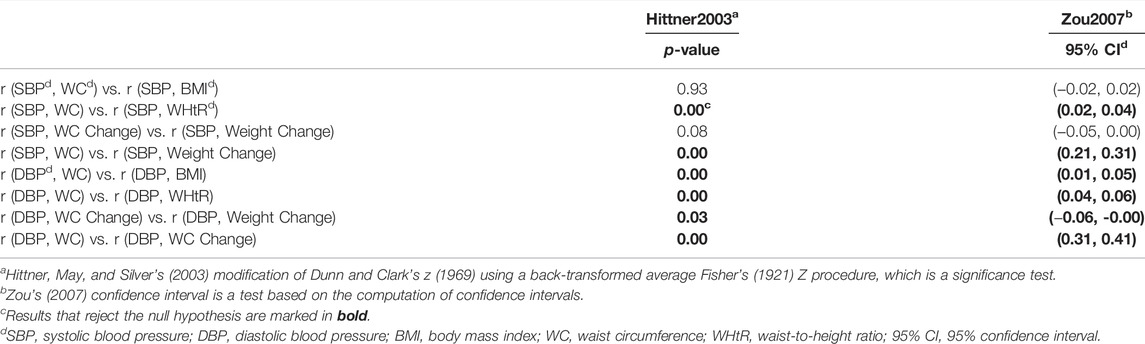
TABLE 1. Test results of comparisons of two overlapping correlations in dependent groups (The Guizhou population health cohort study, China, 2010–2012).
Receiver Operating Characteristic Analyses
The time-dependent ROC curves for half of the follow-up person-years and AUCs of various anthropometric indices for hypertension were shown in Figure 2 and Supplementary Table S5. We found WHtR had the largest AUC for hypertension, but the differences in the AUC for the baseline adiposity indicators were small with overlapping 95% confidence intervals. However, we still found the AUC for WC change from baseline to follow-up was significantly higher than weight change. In addition, we further compared the AUC of WHtR to WC change at half of the follow-up person-years and found a greater AUC for WHtR compared to WC change, but the results were not significant due to overlapping 95% confidence intervals. Detailed information was shown in Supplementary Table S5 and Supplementary Figure S1.
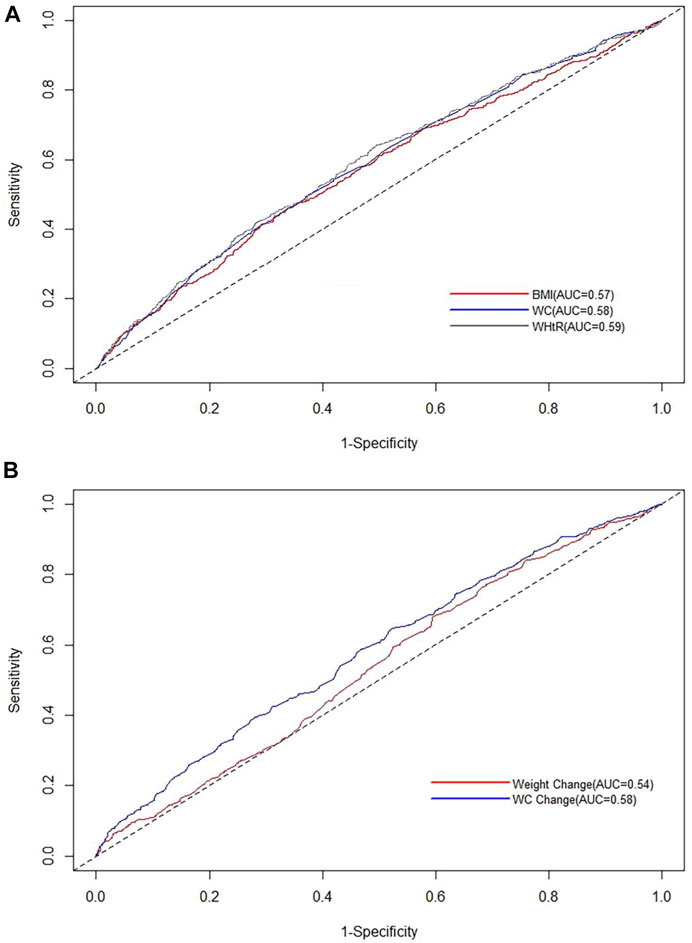
FIGURE 2. Time-dependent receiver operating characteristic curves for half of the follow-up person-years of various anthropometric indices for hypertension (The Guizhou population health cohort study, China, 2010–2012). (A) Time-dependent receiver operating characteristic curves for half of the follow-up person-years of baseline body mass index, waist circumference, and waist-to-height ratio for hypertension; (B) time-dependent receiver operating characteristic curves for half of the follow-up person-years of weight change and waist circumference change from baseline to follow-up for hypertension. BMI, body mass index; WC, waist circumference; WHtR, waist-to-height ratio.
The time-AUC curves between each anthropometric measure and hypertension were displayed in Figure 3. Time-AUC curves showed that WHtR had the slightly largest AUC among the three anthropometric indices, while the AUC of WC change from baseline to follow-up for hypertension was significantly higher than the AUC of weight change when the follow-up time exceeds 6.5 years. And the effect of WC change on hypertension was stronger than that of weight change as it accumulated over time. However, the differences in the AUC for baseline WHtR, BMI, and WC were not statistically significant with overlapping 95% confidence intervals. Additionally, we found WC change from baseline to follow-up had a greater AUC than WHtR after approximately 6.7 years of follow-up, but the results were not significant due to overlapping 95% confidence intervals. Time-AUC curves of WHtR and WC change were placed in Supplementary Figure S2.
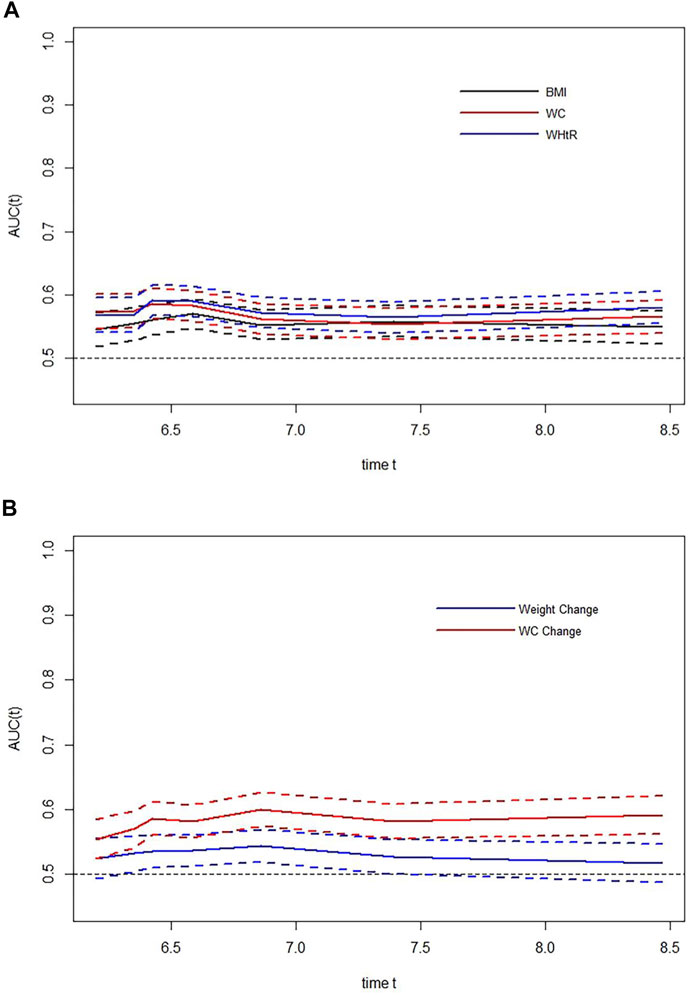
FIGURE 3. Time-dependent areas under curves of various anthropometric indices for hypertension (The Guizhou population health cohort study, China, 2010–2012). (A) Time-dependent areas under curves of baseline body mass index, waist circumference, and waist-to-height ratio for hypertension; (B) time-dependent areas under curves of weight change and waist circumference change from baseline to follow-up for hypertension. BMI, body mass index; WC, waist circumference; WHtR, waist-to-height ratio; AUC, areas under the receiver operating characteristic curves.
Hazard Ratios of Hypertension by Anthropometric Measures
As shown in Table 2, baseline BMI, WC, and WHtR were associated with incident hypertension in the study population. Compared with the normal weight subjects (BMI: 22.0–23.9 kg/m2), obese participants (BMI: ≥28.0 kg/m2) had a statistically increased risk of hypertension, with fully adjusted HRs of 1.50 (95% CI: 1.16–1.93). Central obesity (WC ≥ 85/90 cm) increased the risk of hypertension in participants by 33% (HR = 1.33, 95% CI: 1.12–1.57), after adjustment for potential covariates. Participants with high WHtR (≥0.5) had a 25% increased risk of hypertension compared to subjects with low WHtR (<0.5). For per SD increase in BMI, WC, and WHtR, the risk of hypertension increased by 10%, 15%, and 14%, respectively, in Model 3. We found that WC had the highest HRs for hypertension among all the anthropometric measures. However, HRs were not considered significantly different from each other due to the overlapping 95% confidence intervals.
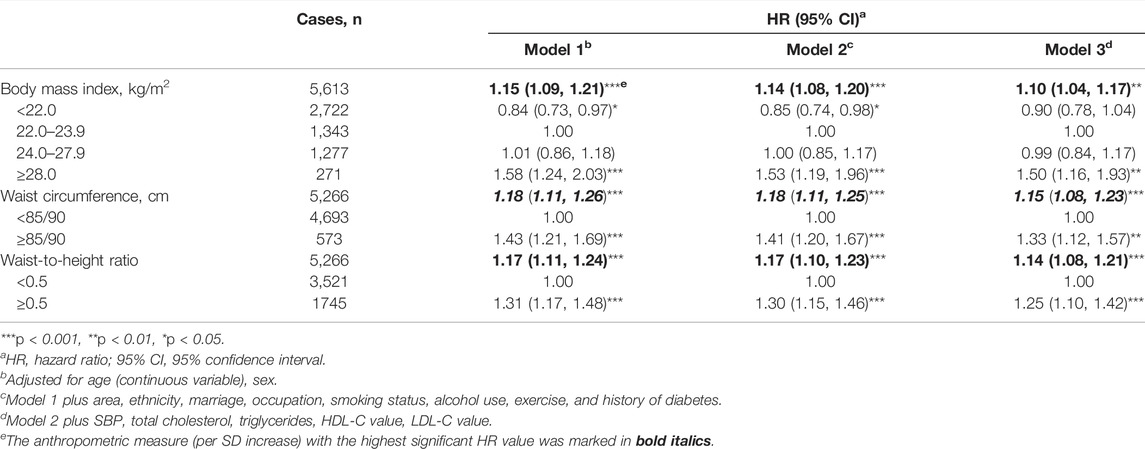
TABLE 2. Hazard ratios (95% confidence intervals) of hypertension associated with baseline body mass index, waist circumference, and waist-to-height ratio (The Guizhou population health cohort study, China, 2010–2012).
We found that weight change and WC change from baseline to follow-up had a statistically increased risk of hypertension after adjustment for major covariates and baseline BMI, with fully adjusted HRs of 1.27 (95% CI: 1.19–1.35) and 1.43 (95% CI: 1.34–1.52), per SD increase (Table 3). Participants with a weight gain of ≥2 to gain of <6 kg or a weight gain of ≥6 kg had significant increased HRs for incident hypertension compared with participants who maintained baseline weight (±2 kg), which were 1.40 (95% CI: 1.16–1.70) and 1.56 (95% CI: 1.29–1.87), respectively, in Model 3. Compared with participants who maintained baseline WC (±3 cm), those with a WC gain of ≥3 to gain of <9 cm or a WC gain of ≥9 cm experienced an increased risk of hypertension with fully adjusted HRs of 1.37 (95% CI: 1.11–1.69) and 1.83 (95% CI: 1.51–2.21), respectively, in Model 3. We found that the HRs of hypertension were higher for each SD increase of long-term WC change than for each SD increase of long-term weight change. Finally, although the 95% confidence intervals were slightly overlapped, there is a trend that the effect of WC change on hypertension was larger than that of weight change.
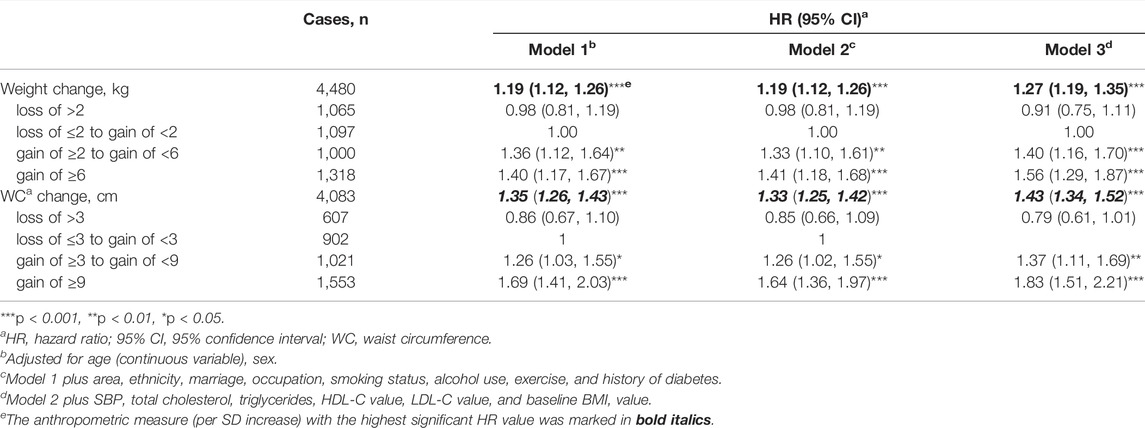
TABLE 3. Hazard ratios (95% confidence intervals) of hypertension associated with weight change and waist circumference change from baseline to follow-up (The Guizhou population health cohort study, China, 2010–2012).
As shown in Supplementary Table S6, we found that generally, C-indices of the models using the central obesity indicators were a little bit higher than those using the general obesity indicators, indicating that the model performance using the central obesity indicators was superior to that using the general obesity indicators. This finding further highlights the importance of WC management in the precaution of hypertension.
Sensitivity Analysis
In the sensitivity analysis conducted to assess the robustness of our results, the effect estimates of baseline BMI, WC, WHtR, weight change, and WC change from baseline to the follow-up on hypertension did not change substantially after excluding subjects diagnosed with hypertension within one year of entry into the cohort (Supplementary Table S7).
We also generated E-values to assess the sensitivity to unmeasured confounding [34]. E-values were higher than the HRs of the covariates, and unmeasurable confounders could not completely negate the association effects observed in our current study. See Supplementary Tables S8–S10 for other details.
Discussion
This study compared the association of general adiposity indicators (baseline BMI and long-term weight change) and central adiposity indicators (baseline WC, WHtR, and long-term WC change) with hypertension based on the first 10-year prospective cohort study of adults in Southwest China, and comprehensively assessed the predictive power of adiposity indicators for hypertension. Based on the results of correlation coefficients, estimated AUCs, and HR values, we found that baseline central adiposity indicators (WC and WHtR) showed a closer association with hypertension. Moreover, our results indicated that WC change from baseline to follow-up was a better predictor of hypertension than weight change in the studied population. It is important to note that these findings are still potentially biased and need to be confirmed by further studies.
Previous studies have demonstrated the association of adiposity indicators such as BMI, WC, and WHtR with hypertension and its predictive power for hypertension, but the best adiposity indicators have not reached consensus [13, 16–19, 35–39]. In view of the potential bias, the present study reported that central obesity indicators showed the best performance for predicting hypertension, that was baseline obesity indicators (WC and WHtR) have been proposed to have a closer association with hypertension than BMI [13, 18, 19, 38], and WC change presented better predictive power for hypertension than weight change. However, some studies concluded that BMI, WC, and WHtR could all be considered comparable in their association with hypertension [35–37]. A few other studies have suggested that BMI best predicted hypertension [16, 17]. In our studied population, we found that central obesity indicators performed better in predicting hypertension based on the results of correlation coefficients, AUC, and HR estimates. This may be because ectopic fat deposition triggers pathological metabolic responses that subsequently increase the risk of metabolic disease [40]. Excessive visceral fat distribution is accompanied by several alterations in hormonal, inflammatory and endothelial levels, and these alterations induce stimulation of several other mechanisms through increased insulin resistance, RAAS and sympathetic nervous system stimulation, impaired stress and chemoreflex cardiovascular control, endothelial dysfunction and increased sodium retention, leading to an increase in blood pressure levels [9]. Visceral fat distribution is influenced in part by genetic factors, which can also lead to elevated blood pressure levels in obese individuals (e.g., tumor necrosis factor-α, β3-adrenergic receptor, G-protein β3 subunit) [41].
Another potential explanation for the stronger association between central obesity indicators and hypertension could be the differences in the definitions of obesity by central obesity indicators versus general obesity indicators. Although BMI is the most widely used indicator of obesity, many studies have shown that BMI does not consider body fat distribution and there is some doubt concerning its ability to predict the accurate risk of hypertension [18, 42–44]. In contrast with BMI, WC, and WHtR are used as surrogate markers for body fat centralization [24, 39, 45]. Moreover, studies have shown that weight change is an indicator to assess changes in total fat mass, while WC change provides the measure of changes in body fat distribution. Most of the change in WC was attributable to changes in subcutaneous abdominal fat compared to weight change [46]. Central distribution of body fat is strongly associated with hypertension [39, 47, 48], and thus central obesity indicators performed better in predicting hypertension.
In addition, further comparisons of baseline to change measures in central obesity indicators based on correlation coefficients and AUC estimates were conducted to identify which indicators were more associated with hypertension. In general, although potential bias remains, we found that baseline central adiposity indicators (WC and WHtR) presented closer associations with hypertension than long-term WC change from baseline to follow-up. Yet after approximately 6.7 years of follow-up, the WC change had better predictive power for hypertension than baseline WHtR. This may be due to the accumulation of effects over time. And the study suggested that in contrast to baseline indices, long-term adiposity change may better capture the effects of excess fat because it affects individual differences in frame size and lean mass that are difficult to measure in population studies [49].
Strengths of this study include the availability of the first 10-year well-characterized population-based cohort in southwest China, whose relatively low loss to follow-up limited the potential bias of risk estimates. To our knowledge, this study is the first report to compare the predictive power of long-term weight change or waist circumference change on hypertension in southwest China. Our results provided a scientific basis for the prevention and control of hypertension development by changing poor lifestyles and suggested that more attention should be paid to waist circumference and central obesity. The present study also has some notable limitations. Firstly, only baseline information for most covariates was used in the regression analysis, which may cause residual confounding if those covariates may vary over time. Secondly, although the current regression analysis adjusted for the major potential confounders, residual confounding due to other confounding factors, such as dietary factors, cannot be excluded. Thirdly, the time of onset of hypertension in this cohort may be inaccurate because it was followed up only once. Fourthly, we could not account for the relationship between some typical obesity indicators (hip circumference, waist-to-hip ratio, and others) and the risk of hypertension due to the lack of data. Fifthly, the selection bias in lost to follow-up can lead to potential bias in our analysis and weaken the association between the obesity indicators and hypertension. Finally, the fact that our study was conducted only in Guizhou province limits the generalizability of our results to southwest China. And more studies should be done before any conclusions can be drawn.
Ethics Statement
The studies involving human participants were reviewed and approved by the Institutional Review Board (or Ethics Committee) of the center of disease control and prevention of Guizhou Province (No. S2017-02). The patients/participants provided their written informed consent to participate in this study.
Author Contributions
TL, JZ, YyW, CF, and KX had a major role in the acquisition of data. YwW and KX performed the statistical analysis. TL and YwW prepared the initial manuscript draft with input from KX. KX and NG polished the manuscript. All authors contributed to manuscript revision, read, and approved the submitted version.
Funding
This work was supported by Guizhou Province Science and Technology Support Program (grant number: Qiankehe [2018]2819).
Conflict of Interest
The authors declare that the research was conducted in the absence of any commercial or financial relationships that could be construed as a potential conflict of interest.
Supplementary Material
The Supplementary Material for this article can be found online at: https://www.ssph-journal.org/articles/10.3389/ijph.2022.1605305/full#supplementary-material
References
1.WHO. Hypertension. Key Facts (2022). Available at: https://www.who.int/news-room/fact-sheets/detail/hypertension (Accessed August 5, 2022).
2.WHO. Hypertension. Overview (2022). Available at: https://www.who.int/health-topics/hypertension#tab=tab_1 (Accessed August 5, 2022).
3. Mills, KT, Bundy, JD, Kelly, TN, Reed, JE, Kearney, PM, Reynolds, K, et al. Global Disparities of Hypertension Prevalence and Control: A Systematic Analysis of Population-Based Studies from 90 Countries. Circulation (2016) 134(6):441–50. doi:10.1161/circulationaha.115.018912
4. Dorans, KS, Mills, KT, Liu, Y, and He, J. Trends in Prevalence and Control of Hypertension According to the 2017 American College of Cardiology/American Heart Association (ACC/AHA) Guideline. J Am Heart Assoc (2018) 7(11):e008888. doi:10.1161/jaha.118.008888
5. Zhang, M, Wu, J, Zhang, X, Hu, CH, Zhao, ZP, Li, C, et al. Prevalence and Control of Hypertension in Adults in China, 2018]. Zhonghua Liu Xing Bing Xue Za Zhi (2021) 42(10):1780–9. doi:10.3760/cma.j.cn112338-20210508-00379
6. Mills, KT, Stefanescu, A, and He, J. The Global Epidemiology of Hypertension. Nat Rev Nephrol (2020) 16(4):223–37. doi:10.1038/s41581-019-0244-2
7.WHO. Hypertension. Treatment (2022). Available at: https://www.who.int/health-topics/hypertension#tab=tab_3 (Accessed August 5, 2022).
8. Wang, Y, and Wang, QJ. The Prevalence of Prehypertension and Hypertension Among US Adults According to the New Joint National Committee Guidelines: New Challenges of the Old Problem. Arch Intern Med (2004) 164(19):2126–34. doi:10.1001/archinte.164.19.2126
9. Seravalle, G, and Grassi, G. Obesity and Hypertension. Pharmacol Res (2017) 122:1–7. doi:10.1016/j.phrs.2017.05.013
10. Ruilope, LM, Nunes Filho, ACB, Nadruz, W, Rodríguez Rosales, FF, and Verdejo-Paris, J. Obesity and Hypertension in Latin America: Current Perspectives. Hipertens Riesgo Vasc (2018) 35(2):70–6. doi:10.1016/j.hipert.2017.12.004
11. Landsberg, L, Aronne, LJ, Beilin, LJ, Burke, V, Igel, LI, Lloyd-Jones, D, et al. Obesity-related Hypertension: Pathogenesis, Cardiovascular Risk, and Treatment: a Position Paper of the Obesity Society and the American Society of Hypertension. J Clin Hypertens (Greenwich) (2013) 15(1):14–33. doi:10.1111/jch.12049
12. Dixon, JB. The Effect of Obesity on Health Outcomes. Mol Cel Endocrinol (2010) 316(2):104–8. doi:10.1016/j.mce.2009.07.008
13. van Dijk, SB, Takken, T, Prinsen, EC, and Wittink, H. Different Anthropometric Adiposity Measures and Their Association with Cardiovascular Disease Risk Factors: a Meta-Analysis. Neth Heart J (2012) 20(5):208–18. doi:10.1007/s12471-011-0237-7
14. Menke, A, Muntner, P, Wildman, RP, Reynolds, K, and He, J. Measures of Adiposity and Cardiovascular Disease Risk Factors. Obesity (Silver Spring) (2007) 15(3):785–95. doi:10.1038/oby.2007.593
15. Janssen, I, Katzmarzyk, PT, and Ross, R. Waist Circumference and Not Body Mass index Explains Obesity-Related Health Risk. Am J Clin Nutr (2004) 79(3):379–84. doi:10.1093/ajcn/79.3.379
16. Schneider, HJ, Klotsche, J, Stalla, GK, and Wittchen, HU. Obesity and Risk of Myocardial Infarction: the INTERHEART Study. Lancet (2006) 367(9516):1052. doi:10.1016/s0140-6736(06)68462-6
17. Bunout, D, Rueda, E, Aicardi, V, Hidalgo, C, and Kauffmann, R. Influence of Body Fat and its Distribution on Cardiovascular Risk Factors in Healthy Subjects. Rev Med Chil (1994) 122(2):123–32.
18. Lee, JW, Lim, NK, Baek, TH, Park, SH, and Park, HY. Anthropometric Indices as Predictors of Hypertension Among Men and Women Aged 40-69 Years in the Korean Population: the Korean Genome and Epidemiology Study. BMC Public Health (2015) 15:140. doi:10.1186/s12889-015-1471-5
19. Zhang, Y, Gu, Y, Wang, N, Zhao, Q, Ng, N, Wang, R, et al. Association between Anthropometric Indicators of Obesity and Cardiovascular Risk Factors Among Adults in Shanghai, China. BMC Public Health (2019) 19(1):1035. doi:10.1186/s12889-019-7366-0
20. Hu, L, Hu, G, Huang, X, Zhou, W, You, C, Li, J, et al. Different Adiposity Indices and Their Associations with Hypertension Among Chinese Population from Jiangxi Province. BMC Cardiovasc Disord (2020) 20(1):115. doi:10.1186/s12872-020-01388-2
21. Deng, G, Yin, L, Liu, W, Liu, X, Xiang, Q, Qian, Z, et al. Associations of Anthropometric Adiposity Indexes with Hypertension Risk: A Systematic Review and Meta-Analysis Including PURE-China. Medicine (Baltimore) (2018) 97(48):e13262. doi:10.1097/md.0000000000013262
22. Zhou, BF. Predictive Values of Body Mass index and Waist Circumference for Risk Factors of Certain Related Diseases in Chinese Adults-Sstudy on Optimal Cut-Off Points of Body Mass index and Waist Circumference in Chinese Adults. Biomed Environ Sci (2002) 15(1):83–96.
23. Sun, H, Zheng, M, Wu, S, Chen, M, Cai, J, and Yang, X. Waist Circumference and Incidence of Hypertension in Chinese Adults : Observations from the Kailuan Study. Herz (2017) 42(7):677–83. doi:10.1007/s00059-016-4501-x
24. Browning, LM, Hsieh, SD, and Ashwell, M. A Systematic Review of Waist-To-Height Ratio as a Screening Tool for the Prediction of Cardiovascular Disease and Diabetes: 0·5 Could Be a Suitable Global Boundary Value. Nutr Res Rev (2010) 23(2):247–69. doi:10.1017/s0954422410000144
25. Unger, T, Borghi, C, Charchar, F, Khan, NA, Poulter, NR, Prabhakaran, D, et al. 2020 International Society of Hypertension Global Hypertension Practice Guidelines. Hypertension (2020) 75(6):1334–57. doi:10.1161/hypertensionaha.120.15026
26. Association2, AD. 2. Classification and Diagnosis of Diabetes: Standards of Medical Care in Diabetes-2020. Diabetes Care (2020) 43(1):S14–s31. doi:10.2337/dc20-S002
27. Schober, P, Boer, C, and Schwarte, LA. Correlation Coefficients: Appropriate Use and Interpretation. Anesth Analg (2018) 126(5):1763–8. doi:10.1213/ane.0000000000002864
28. Diedenhofen, B, and Musch, J. Cocor: a Comprehensive Solution for the Statistical Comparison of Correlations. PLoS One (2015) 10(3):e0121945. doi:10.1371/journal.pone.0121945
29. Uno, H, Cai, T, Tian, L, and Wei, LJ. Evaluating Prediction Rules for T-Year Survivors with Censored Regression Models. J Am Stat Assoc (2007) 102(478):527–37. doi:10.1198/016214507000000149
30. Hung, H, and Chiang, C-T. Estimation Methods for Time-dependent AUC Models with Survival Data. Can J Statistics-Revue Canadienne De Statistique (2010) 38(1):8–26.
31. Blanche, P, Dartigues, JF, and Jacqmin-Gadda, H. Estimating and Comparing Time-dependent Areas under Receiver Operating Characteristic Curves for Censored Event Times with Competing Risks. Stat Med (2013) 32(30):5381–97. doi:10.1002/sim.5958
32. VanderWeele, TJ, and Ding, P. Sensitivity Analysis in Observational Research: Introducing the E-Value. Ann Intern Med (2017) 167(4):268–74. doi:10.7326/m16-2607
33. Haneuse, S, VanderWeele, TJ, and Arterburn, D. Using the E-Value to Assess the Potential Effect of Unmeasured Confounding in Observational Studies. Jama (2019) 321(6):602–3. doi:10.1001/jama.2018.21554
34. Aminian, A, Zajichek, A, Arterburn, DE, Wolski, KE, Brethauer, SA, Schauer, PR, et al. Association of Metabolic Surgery with Major Adverse Cardiovascular Outcomes in Patients with Type 2 Diabetes and Obesity. Jama (2019) 322(13):1271–82. doi:10.1001/jama.2019.14231
35. Paniagua, L, Lohsoonthorn, V, Lertmaharit, S, Jiamjarasrangsi, W, and Williams, MA. Comparison of Waist Circumference, Body Mass Index, Percent Body Fat and Other Measure of Adiposity in Identifying Cardiovascular Disease Risks Among Thai Adults. Obes Res Clin Pract (2008) 2(3):1–23. doi:10.1016/j.orcp.2008.05.003
36. Nyamdorj, R, Qiao, Q, Söderberg, S, Pitkäniemi, J, Zimmet, P, Shaw, J, et al. Comparison of Body Mass index with Waist Circumference, Waist-To-Hip Ratio, and Waist-To-Stature Ratio as a Predictor of Hypertension Incidence in Mauritius. J Hypertens (2008) 26(5):866–70. doi:10.1097/HJH.0b013e3282f624b7
37.Obesity in Asia Collaboration. Is central Obesity a Better Discriminator of the Risk of Hypertension Than Body Mass index in Ethnically Diverse Populations? J Hypertens (2008) 26(2):169–77. doi:10.1097/HJH.0b013e3282f16ad3
38. Tseng, CH, Chong, CK, Chan, TT, Bai, CH, You, SL, Chiou, HY, et al. Optimal Anthropometric Factor Cutoffs for Hyperglycemia, Hypertension and Dyslipidemia for the Taiwanese Population. Atherosclerosis (2010) 210(2):585–9. doi:10.1016/j.atherosclerosis.2009.12.015
39. Zhou, Z, Hu, D, and Chen, J. Association between Obesity Indices and Blood Pressure or Hypertension: Which index Is the Best? Public Health Nutr (2009) 12(8):1061–71. doi:10.1017/s1368980008003601
40. Piché, ME, Poirier, P, Lemieux, I, and Després, JP. Overview of Epidemiology and Contribution of Obesity and Body Fat Distribution to Cardiovascular Disease: An Update. Prog Cardiovasc Dis (2018) 61(2):103–13. doi:10.1016/j.pcad.2018.06.004
41. Pausova, Z, Jomphe, M, Houde, L, Vezina, H, Orlov, SN, Gossard, F, et al. A Genealogical Study of Essential Hypertension with and without Obesity in French Canadians. Obes Res (2002) 10(6):463–70. doi:10.1038/oby.2002.64
42. Nuttall, FQ. Body Mass Index: Obesity, BMI, and Health: A Critical Review. Nutr Today (2015) 50(3):117–28. doi:10.1097/nt.0000000000000092
43. Lee, CM, Huxley, RR, Wildman, RP, and Woodward, M. Indices of Abdominal Obesity Are Better Discriminators of Cardiovascular Risk Factors Than BMI: a Meta-Analysis. J Clin Epidemiol (2008) 61(7):646–53. doi:10.1016/j.jclinepi.2007.08.012
44. Dong, J, Ni, YQ, Chu, X, Liu, YQ, Liu, GX, Zhao, J, et al. Association between the Abdominal Obesity Anthropometric Indicators and Metabolic Disorders in a Chinese Population. Public Health (2016) 131:3–10. doi:10.1016/j.puhe.2015.08.001
45. Ko, GT, Chan, JC, Woo, J, Lau, E, Yeung, VT, Chow, CC, et al. Simple Anthropometric Indexes and Cardiovascular Risk Factors in Chinese. Int J Obes Relat Metab Disord (1997) 21(11):995–1001. doi:10.1038/sj.ijo.0800508
46. Cornier, MA, Després, JP, Davis, N, Grossniklaus, DA, Klein, S, Lamarche, B, et al. Assessing Adiposity: a Scientific Statement from the American Heart Association. Circulation (2011) 124(18):1996–2019. doi:10.1161/CIR.0b013e318233bc6a
47. Li, WC, Chen, IC, Chang, YC, Loke, SS, Wang, SH, and Hsiao, KY. Waist-to-height Ratio, Waist Circumference, and Body Mass index as Indices of Cardiometabolic Risk Among 36, 642 Taiwanese Adults. Eur J Nutr (2013) 52(1):57–65. doi:10.1007/s00394-011-0286-0
48. Hsieh, SD, and Yoshinaga, H. Waist/height Ratio as a Simple and Useful Predictor of Coronary Heart Disease Risk Factors in Women. Intern Med (1995) 34(12):1147–52. doi:10.2169/internalmedicine.34.1147
Keywords: risk factors, cohort study, obesity, hypertension, anthropometric indices
Citation: Liu T, Wang Y, Gao N, Zhou J, Wang Y, Fu C and Xu K (2022) Central Adiposity Indicators Maintain a Stronger Association With the Risk of Hypertension: A Prospective Cohort Study in Southwest China. Int J Public Health 67:1605305. doi: 10.3389/ijph.2022.1605305
Received: 08 August 2022; Accepted: 23 September 2022;
Published: 05 October 2022.
Edited by:
Paolo Chiodini, University of Campania Luigi Vanvitelli, ItalyReviewed by:
Fabrizio Pasanisi, University of Naples Federico II, ItalyVittorio Simeon, Università della Campania Luigi Vanvitelli, Italy
Copyright © 2022 Liu, Wang, Gao, Zhou, Wang, Fu and Xu. This is an open-access article distributed under the terms of the Creative Commons Attribution License (CC BY). The use, distribution or reproduction in other forums is permitted, provided the original author(s) and the copyright owner(s) are credited and that the original publication in this journal is cited, in accordance with accepted academic practice. No use, distribution or reproduction is permitted which does not comply with these terms.
*Correspondence: Tao Liu, liutaombs@163.com; Kelin Xu, kelinxu12@fudan.edu.cn
†These authors have contributed equally to this work and share first authorship