- 1German Institute for Economic Research (DIW), Berlin, Germany
- 2Berlin Graduate School of Social Sciences, Humboldt University of Berlin, Berlin, Germany
- 3Department of Nursing Science, University of Trier, Trier, Germany
Objectives: Our research provides competing hypotheses and empirical evidence how associations between objectively social isolation and subjective loneliness differ between host populations, migrants, and refugees.
Methods: The analysis uses data of 25,171 participants from a random sample of the German population (SOEP v.35). We estimate regression models for the host population, migrants, and refugees and test five hypotheses on the association between social isolation and loneliness using a Bayesian approach in a multiverse framework.
Results: We find the strongest relative support for an increased need for social inclusion among refugees, indicated by a higher Bayes factor compared to the hosts and migrants. However, all theoretically developed hypotheses perform poorly in explaining the major pattern in our data: The association of social isolation and loneliness is persistently lower for migrants (0.15 SD−0.29 SD), with similar sizes of associations for refugees and the host population (0.38 SD−0.67 SD).
Conclusion: The migration history must be actively considered in health service provision and support programs to better cater to the needs of the different groups.
Introduction
Loneliness has been called a pandemic of modern times [1, 2], constituting a severe problem for modern societies. It has been associated with an increased risk of developing mental health problems [3–5] and can exacerbate existing vulnerabilities to other health outcomes [6]. Several societal trends have been proposed as explanations for increased risks of loneliness in recent decades, among them global mobility [7]. In 2019, 272 million migrants lived outside of their home country [8]. At the same time, UNHCR counted 25.9 million refugees, 41 million internally displaced persons, and another 3.5 million asylum seekers [9].
Loneliness is defined as the subjective feeling of disconnection from social interactions in everyday life [10]. It is the cognitive evaluation of the objective absence of social networks and support. The objective aspect of the definition of loneliness, absence of a social support network, is often referred to as social isolation [11]. Although social isolation and loneliness are often used interchangeably [12], they are not identical [13]. While social isolation does not per se invoke feelings of loneliness [11], it is an important predictor of loneliness throughout the entire life [14, 15]. It is therefore important to know under which conditions social isolation works as a strong predictor for loneliness and hence identify more susceptible groups. Following Diderichsen et al., we use the term differential exposure denoting the differences in incidences of social isolation and loneliness among the groups under investigation. Further, we refer to the differences in the strength of the association of social isolation with loneliness between the groups as differential susceptibility to social isolation [16].
Migrants are more often at risk of greater exposure to social isolation than host populations, as their networks in the new environment need to be (re-) established [17, 18]. Additionally, they are prone to experiencing higher rates of loneliness due to cultural differences and language barriers [19]. Whether comparable trends to exposure to social isolation and loneliness exist for refugees has yet to be established. In the current study, we not only study these groups’ risks of suffering from objective and subjective social network deprivation: The economic, legal, and social differences in context motivate our investigation of the question whether—under the condition of social isolation—migrants, refugees, and the host population exhibit differing susceptibilities to social isolation. Answering this question can guide interventions and their prioritization in this area.
We propose competing hypotheses for differential susceptibility to social isolation. These hypotheses imply that host population, migrants, and refugees differ in reaction to the lack of social networks and support given their different circumstances (Table 1). Loneliness is often referred to as an evolutionary warning signal of the body, indicating deviations from a norm of social interaction. Hence, susceptibility to social isolation should not differ substantively based on context [20, 21]. Yet, as we propose context moderation, we expect the maximum difference between the associations among the host population, migrants, and refugees to be above a substantive threshold—in H1, 0.20 standard deviations (for a more detailed discussion of how we selected this value, see Supplementary Material S4).
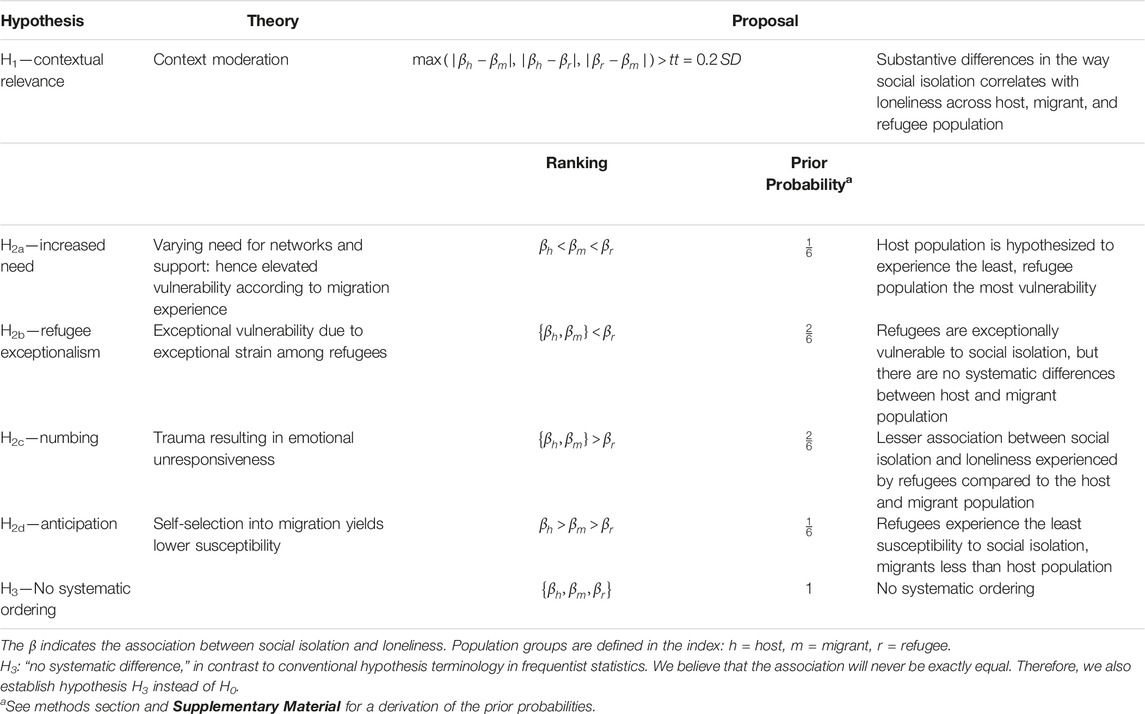
TABLE 1. Hypotheses on different degrees of vulnerability to social isolation with respect to loneliness. Random sample of the host population, migrants and refugees in Germany, 2016/2017 (SOEP v.35).
H2 advances a set of competing rankings of differential susceptibility between the host population, migrants, and refugees, founded in migration context and experiences: In detail, H2a proposes that the highest need for social support and inclusion exists for refugees and to a lesser extent for migrants. Their circumstances make them particularly susceptible to social isolation [22]. H2b hypothesizes an exceptional susceptibility in refugees, evinced in an elevated association between social isolation and loneliness compared to migrants and the host population [23]. H2c expects an emotionally numb response among refugees resulting from traumatic displacement experiences. This would lead to a smaller association between social isolation and loneliness compared to the levels of sensibility in the host and migrant population [24, 25]. H2d postulates that social isolation is anticipated by migrants, and even more so by refugees, through self-selection into migration, reducing their susceptibility to social isolation [26, 27]. H3 is the baseline hypothesis in this analysis, suggesting no systematic differences in susceptibility between groups.
We use one of the few available data sets which includes comparable and harmonized data for refugees, migrants, and a host population: the German Socio-Economic Panel Study (SOEP, v.35, N = 25,171). We use a Bayesian Evaluation of Informative Hypotheses (BEIH) framework to evaluate the hypotheses [28, 29], testing the robustness of our results in a multiverse framework [30, 31].
Methods
Study Population
We use 2016 and 2017 data from the SOEP v.35 [32]. The household survey is a stratified random sample of the German population with recent booster samples for migrants and refugees (DOI: 10.5684/soep-core.v35). Notably, the IAB-BAMF-SOEP refugee survey enabling the analysis of many cases of recently arrived refugees to Germany between 2013 and 2016. The SOEP draws its refugee samples from the Central Register of Foreign Nationals. Hence, the refugee survey is a random sample from a clearly defined population. This is a clear advantage, as most refugee surveys are based on highly targeted, clinical, or convenience samples.
Migration Status
We group individuals into [1] Germans and second-generation migrants–labelled host population (n = 16,658) [2], first generation migrants (n = 3790) and [3] refugees (or similar protected) who have arrived since 2013 as part of the IAB-BAMF-SOEP survey (n = 4723). We define the host population as those born as German nationals or those born in the Federal Republic of Germany as of 1949. Further, we define refugees in Germany as those having applied for asylum, regardless of the outcome of their application.
Loneliness
We use the three-item version of the UCLA loneliness scale as our measure for subjective loneliness [33]. Items are rated on 5-point scales (0 = “never”, 1 = “rarely”, 2 = “sometimes”, 3 = “often”, 4 = “very often”). Based on this scale, we test two different outcome measures of loneliness [1]: a simple summary score and [2] the factor score from a confirmatory factor analysis of the three items. Migrants and host population were surveyed on the three items in 2017, refugees in 2016, leading us to transmit the 2016 information to 2017. Measurement invariance tests across groups can be found in the supplementary material (Supplementary Figures S1, S2 and Supplementary Tables S1, S2).
Social Isolation
We measure social isolation as a composite index, across three domains consisting of several indicators [34]:
1. The size of the support network (SS), measured as the number of individuals given by respondents as social support in three categories. The SOEP reports the social support (SS) for refugees in 2017 and for the host population and other migrants in 2016. Hence, we transmit the 2016 information for SOEP participants to 2017.
2. Living and partnership arrangements (LA): a) having a spouse and b) presence of other household members.
3. Frequency of attending social activities (SA): a) church, b) cultural activities, c) cinema/disco, d) sports, e) arts.
For each indicator and domain, and for the total calculation of domains, we test two alternative cut-off points: one that allows for more and another allowing for less substitution within and between domains (N = 16) (see Supplementary Material S3). Substitution refers to the assumption that a lack in one dimension can compensated by another.
Covariates
We control for age groups and gender as well as education, residency in rural and urban areas, and residency in former East or West Germany (Supplementary Material S2).
Statistical Analysis
The central parameters that represent the quantities of interest from our hypotheses are the coefficients (
We use the BEIH framework (see Supplementary Material S8), designed for a comparative evaluation of competing hypotheses (Ht) [35]. The general estimation procedure used for the posterior distribution of the parameters is the Integrated Nested Laplace Approximation (INLA) [36]. The key feature of the BEIH method is to compare the observed support
Recent research proposes that studies based on secondary data report all plausible specifications of their data coding and sample definitions [30, 31]. This reduces the probability of reporting findings specific to certain idiosyncratic decisions in the process of the data analysis [38]. Based on the definition of social isolation and the different cut-off points presented, in addition to alterations in sample definition and coding, we report all plausible specifications or codings in a multiverse framework (Supplementary Material S3–S5).
Results
Descriptive statistics of loneliness are provided in Table 2. The factor score of loneliness is smallest for the host population (M = -0.17, SD = 0.85), and larger for migrants (M = −0.03, SD = 0.97) and refugees (M = 0.55, SD = 1.24). The magnitude of the differences is even more intuitive when observing the summary score between the groups, ranging from a M = 2.88 (SD = 2.22) in the host population to a M = 4.81 (SD = 3.23) for refugees. The dispersion is larger in the refugee population compared to the host population and migrants. Supplementary Table S7 lays out further descriptive statistics.

TABLE 2. Descriptive statistics of the two dependent variables of interest: a factor score of loneliness and the sum score—by subgroup. Random sample of the host population, migrants and refugees in Germany, 2016/2017 (SOEP v.35).
Notably, the prevalence of social isolation depends on the choice of cut-off points (Figure 1). The less we allow for substitution among and between dimensions, the more people count as socially isolated. Under full substitution (coding 1111), less than 1% of the sample are categorized as socially isolated. When only partial substitution is allowed (coding 0000), social isolation becomes as high as 30% among the group of refugees, and 15% for host population and migrants. Disallowing full substitutability in the domain of social activities most strongly increases the prevalence of social isolation. Overall, refugees are more socially isolated than migrants and the host population. Host population and migrants do not differ greatly regarding the prevalence of social isolation.
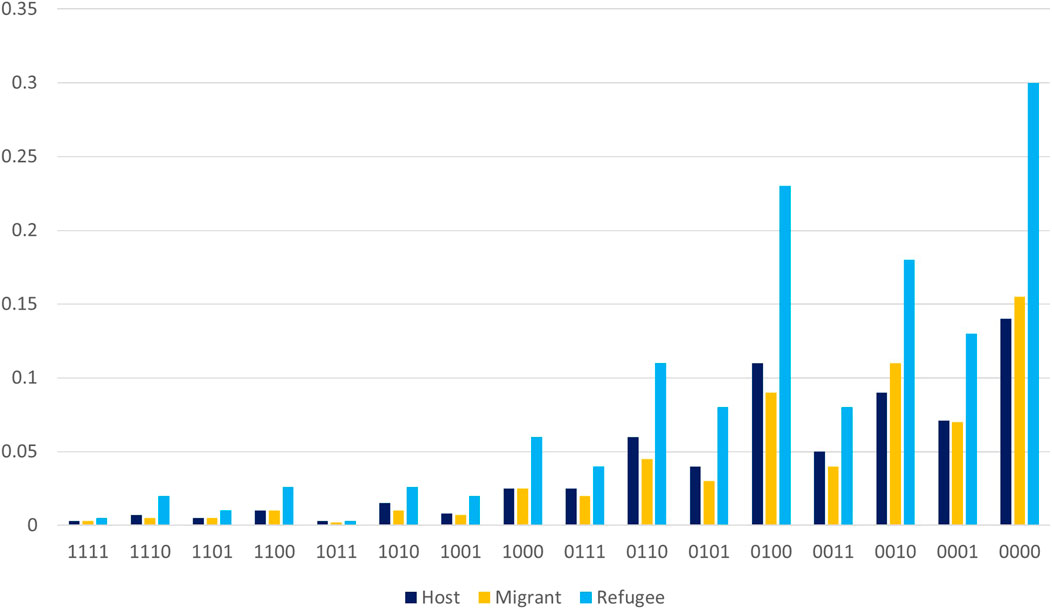
FIGURE 1. Prevalence of social isolation by migration status over different codings of social isolation. Random sample of the host population, migrants and refugees in Germany, 2016/2017 (SOEP v.35). aX-Axis: Coding choice of the social isolation indicator: The 4 digits for each isolation indicator on the X-axis describe the degree of substitution allowed in each step of the generation of the indicator for social isolation (see equations 1 and 2 in the Supplementary Material S4 (p.18)). Y-Axis: prevalence of social isolation given the choice of the social isolation indicator. bThe first digit represents the coding for the degree of substitution across dimensions (
Results from the BEIH analysis are presented in Figure 2, containing two sets of results over the 16 codings of social isolation on the Y-axis in terms of SD. On the left, the posterior mean and 95%-credible interval of the regression coefficients of social isolation are plotted (averaged across specifications in Supplementary Figures S7, S8—for a list with detailed posterior means and credible intervals consult Supplementary Table S8). On the right, the table reports the BF and PMP. The darker the box, the higher the PMP for the hypotheses corresponding to the coding of social isolation. We also would like to note the lowest absolute number of observations counted as socially isolated in some of the codings. They naturally lead to a smaller number of cases per cell in the analysis, in some cases with fewer than 50 observations per cell, marked in grey. We consider codings with fewer than 50 cases as unreliable for interpretation and focus on the results from models with sufficient cases of social isolation in all three groups.
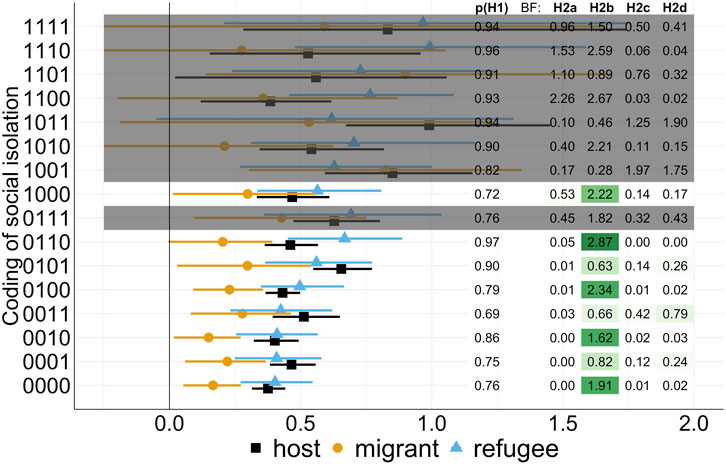
FIGURE 2. Regression results and relative support for the hypotheses. Random sample of the host population, migrants and refugees in Germany, 2016/2017 (SOEP v.35). aThe table compares posterior model probabilities (colour) and Bayes Factor (numerics) to the credible intervals derived from analysis across the different codings of the social isolation indicator. bThe X-axis refers to effect sizes while the Y-axis describes different choices of codings of the social isolation indicator in the multiverse analysis (see also Supplementary Figures S4A,B). cThe 4 digits of each isolation indicator describe the degree of substitution allowed in each step of the generation of the indicator for social isolation. The first digit represents the coding for the degree of substitution across dimensions (
Regardless of the coding of social isolation, we see a substantial association of social isolation with loneliness in all three groups (Figure 2, Supplementary Table S8). Still, the posterior mean of the migrant population is persistently lower than that of the host population and that of refugees. It ranges between 0.149 [SI Coding: 0010, 95%-CI: 0.017; 0.271] and 0.298 [SI Coding: 1000, 95%-CI 0.014; 0.558]. The posterior means of hosts and refugees are larger and similar in size, with an effect size of 0.50 SD and ranging from 0.376 [SI Coding: 0000, 95%-CI: 0.315; 0.442] to 0.655 [SI Coding: 0101, 95%-CI: 0.548; 0.772] for the host population and for refugees from 0.403 [SI Coding: 0000, 95%-CI: 0.271; 0.546] to 0.668 [SI Coding: 0110, 95%-CI: 0.452; 0.888]. This means being socially isolated is associated with about a 0.20 SD higher loneliness score for migrants and about a 0.50 SD higher score of loneliness for the host population and refugees in Germany. These comparisons are always made with respect to those individuals who are not socially isolated within their respective group.
The first column from the left of the table in Figure 2 reflects the evaluation of hypothesis
Figure 2 also shows the BF from the evaluation of the competing hypotheses H2a-d. The PMP for hypothesis H2b (refugee exceptionalism) is largest compared to the other four hypotheses, as indicated by the darker green background of the BFs. The BF, however, remains below one in three of the codings with sufficient variation on the independent variable of interest. Hence, despite being favored relative to other hypotheses, it is less supported by our data than would be expected by chance alone, which is a poor absolute performance.
In the other cases, H2b receives the highest BF, with 2.89 times more support for the hypothesis than expected by chance in the SI Coding: 0110 (Figure 2). Hence, we find most support for the hypothesis that the association between social isolation and loneliness is larger for refugees than for the host population and migrants. H2b, the increased need hypothesis, comes closest to the data pattern.
This result of the evaluation is at odds with the observations of posterior means and the group level credible intervals. A focus on this output shows that the association between social isolation and loneliness is lower for migrants and about equal for refugees and the host population across different social isolation codings. This phenomenon is an indication that we yet have not correctly identified the most suitable hypothesis given the data.
Discussion
We hypothesized migration status shaping the susceptibility to social isolation. Among the set of hypotheses, we saw that the “refugee exceptionalism” hypothesis H2b received most support relative to the other hypotheses in the data. The finding that the association between social isolation and loneliness is, overall, weaker for migrants than for the host and refugee groups conflicts with the results of our formal procedure for testing hypotheses. This contradiction indicates that the set of hypotheses did not include the most relevant proposition about the relative strength of association between social isolation and loneliness. The hypothesis that migrants do indeed show a lesser susceptibility to social isolation should be tested in future studies on independent samples.
Looking for an explanation for this finding, we turn to the composition of the migrant group and to alternative hypotheses we equally postulated. The weaker association between social isolation and loneliness for migrants can be attributed to positive self-selection and anticipation (H2d). Migrants might more readily accept their social circumstances, as they actively chose their destination based on social network considerations [26]. Meanwhile, refugees have less choice of destination. Their self-selection to migrate is less linked to a well-functioning diaspora than to a need to survive.
One puzzle is the similar pattern of susceptibility to social isolation among refugees and the host population. Deviations from social norms are perhaps more awkward for individuals from the host population, who compare themselves to members of the host community whom they should ostensibly resemble [20]. Meanwhile, a migrant in the same age group is aware of his or her situation and can evaluate it in a positive light. This sensitivity to social deviation from the norm in the host population would then be equal in strength compared to the susceptibility previously postulated for the refugee population.
Overall, refugees show the highest exposure to social isolation and loneliness. Given that they also have a high susceptibility to social isolation, they can be regarded as the most vulnerable group in this analysis, leaving aside their capacity to respond to the exposure [16].
One limitation of our analysis is that data did not allow us to test the underlying mechanisms directly. This also makes it difficult to assess why the data showed a clear but unexpected result in migrants being less susceptible to social isolation. Further research needs to examine the proposed mechanisms separately. We assume the external validity of results, as the prevailing mechanisms should not differ by country. However, the refugee policies of host countries can influence self-selection, for instance through visa sponsorship. Despite the large data set used, a more precise differentiation within the migrant and refugee group is not implemented. It is possible that country of origin, reason for migration, or duration of stay explain how social isolation are linked to loneliness in varying degrees. Last, we argued that social isolation is deprivation in social contacts across domains. While our data cover three domains and several indicators, there are aspects we do not measure, for example, closeness of the social network or integration into transnational networks [39]. More specific data would be necessary for the last two considerations to be further explored.
As established in previous research, loneliness is particularly strongly linked to negative mental health outcomes. This cost to individuals and society should be avoided where possible [2, 13]. Our study suggests that interventions to lessen the risks of mental ill-health can start with the prevention of social isolation. The groups at stake might be best stimulated in different ways in terms of environmental prevention. Nevertheless, the present analysis cannot infer direct policy guidance. Particularly interesting would be a closer examination of whether a substitution effect among different social activities exists. This is just one way forward in studying the association between social isolation and loneliness in the context of migration.
Editors Note
This manuscript was accepted without revision, after an informal review by one co-editor in chief. For eleven months we were unable to find reviewers. Please read our editorial about the reviewer crisis: https://www.ssph-journal.org/articles/10.3389/phrs.2022.1605407/full.
Ethics Statement
Ethical review and approval was not required for the study on human participants in accordance with the local legislation and institutional requirements. Written informed consent for participation was not required for this study in accordance with the national legislation and the institutional requirements.
Author Contributions
All authors LL, HK, and AT contributed equally to the stages of theory and concept development as well as to the interpreting stage. LL and HK conducted the data preparation, LL provided the descriptive statistics while HK implemented the main analysis. LL compiled a first draft manuscript. All authors engaged in re-writing and approved the final version of the manuscript.
Funding
The research was supported by the Deutsche Forschungsgemeinschaft (DFG, German Research Foundation) [415809395, 427279591, and 40965412]; and by the Leibniz Association [K205/2016].
Conflict of Interest
The authors declare that they do not have any conflicts of interest.
Supplementary Material
The Supplementary Material for this article can be found online at: https://www.ssph-journal.org/articles/10.3389/ijph.2022.1604576/full#supplementary-material
References
1. Cacioppo, JT, and Cacioppo, S. Loneliness in the Modern Age: An Evolutionary Theory of Loneliness (ETL). In: Advances in Experimental Social Psychology. Academic Press (2018). p. 127.
2. Holt-Lunstad, J. The Potential Public Health Relevance of Social Isolation and Loneliness: Prevalence, Epidemiology, and Risk Factors. Public Pol Aging Rep (2017) 27(4):127–30. doi:10.1093/ppar/prx030
3. Thurston, RC, and Kubzansky, LD. Women, Loneliness, and Incident Coronary Heart Disease. Psychosom Med (2009) 71(8):836–42. doi:10.1097/PSY.0b013e3181b40efc
4. Lee, EE, Depp, C, Palmer, BW, Glorioso, D, Daly, R, Liu, J, et al. High Prevalence and Adverse Health Effects of Loneliness in Community-Dwelling Adults across the Lifespan: Role of Wisdom as a Protective Factor. Int Psychogeriatr (2019) 31(10):1447–62. doi:10.1017/S1041610218002120
5. Jessen, MAB, Pallesen, AVJ, Kriegbaum, M, and Kristiansen, M. The Association between Loneliness and Health – a Survey-Based Study Among Middle-Aged and Older Adults in Denmark. Aging Ment Health (2018) 22(10):1338–43. doi:10.1080/13607863.2017.1348480
6. Tsur, N, Stein, JY, Levin, Y, Siegel, A, and Solomon, Z. Loneliness and Subjective Physical Health Among War Veterans: Long Term Reciprocal Effects. Soc Sci Med (2019) 234(112373):112373. doi:10.1016/j.socscimed.2019.112373
7. Djundeva, M, and Ellwardt, L. Social Support Networks and Loneliness of Polish Migrants in the Netherlands. J Ethn Migr Stud (2019) 46:1281. [Internet]Available from: https://www.tandfonline.com/action/journalInformation?journalCode=cjms20.
8.United Nations Population Division. International Migration Stock (2019). [cited 2020 Mar 18]. Available from: https://www.un.org/en/development/desa/population/migration/data/estimates2/estimates19.asp.
9.UNHCR. Figures at a Glance (2009). Available from: https://www.unhcr.org/figures-at-a-glance.html.
10. de Jong Gierveld, J, van Tilburg, T, and Dykstra, PA. Loneliness and Social Isolation. In: D Perlman, and A Vangelisti, editors. The Cambridge Handbook of Personal Relationships. Cambridge, UK: Cambridge University Press (2006). 485.
11. Victor, C, Scambler, S, Bond, J, and Bowling, A. Being Alone in Later Life: Loneliness, Social Isolation and Living Alone. Rev Clin Gerontol (2000) 10(4):407–17. doi:10.1017/s0959259800104101
12. Malcolm, M, Frost, H, and Cowie, J. Loneliness and Social Isolation Causal Association with Health-Related Lifestyle Risk in Older Adults: A Systematic Review and Meta-Analysis Protocol. Syst Rev (2019) 8(1):48. doi:10.1186/s13643-019-0968-x
13. Beutel, ME, Klein, EM, Brähler, E, Reiner, I, Jünger, C, Michal, M, et al. Loneliness in the General Population: Prevalence, Determinants and Relations to Mental Health. BMC Psychiatry (2017) 17:97. doi:10.1186/s12888-017-1262-x
14. Soest, Tvon, Luhmann, M, Hansen, T, and Gerstorf, D. Development of Loneliness in Midlife and Old Age: Its Nature and Correlates. J Pers Soc Psychol (2020) 118(2):388–406. doi:10.1037/pspp0000219
15. Luhmann, M, and Hawkley, L. Age Differences in Loneliness from Late Adolescence to Oldest Old Age. Dev Psychol (2016) 52(6):943–59. doi:10.1037/dev0000117
16. Diderichsen, F, Hallqvist, J, and Whitehead, M. Differential Vulnerability and Susceptibility: How to Make Use of Recent Development in Our Understanding of Mediation and Interaction to Tackle Health Inequalities. Int J Epidemiol (2019) 48:268–74. doi:10.1093/ije/dyy167
17. Koelet, S, and de Valk, HAG. Social Networks and Feelings of Social Loneliness after Migration: The Case of European Migrants with a Native Partner in Belgium. Ethnicities (2016) 16(4):610–30. doi:10.1177/1468796816638398
18. Ten Kate, RLF, Bilecen, B, Steverink, N, and Castle, NG. A Closer Look at Loneliness: Why Do First-Generation Migrants Feel More Lonely Than Their Native Dutch Counterparts? Gerontologist (2020) 60(2):291–301. doi:10.1093/geront/gnz192
19. Lim, MH, Eres, R, and Vasan, S. Understanding Loneliness in the Twenty-First century: an Update on Correlates, Risk Factors, and Potential Solutions. Soc Psychiatry Psychiatr Epidemiol (2020) 55(7):793–810. doi:10.1007/s00127-020-01889-7
20. Cacioppo, JT, and Patrick, W. Loneliness : Human Nature and the Need for Social Connection. W. W. Norton (2008). 317.
21. Cacioppo, S, Bangee, M, Balogh, S, Cardenas-Iniguez, C, Qualter, P, and Cacioppo, JT. Loneliness and Implicit Attention to Social Threat: A High-Performance Electrical Neuroimaging Study. Cogn Neurosci. Available from: http://www.ncbi.nlm.nih.gov/pubmed/26274315.
22. Porter, M, and Haslam, N. Predisplacement and Postdisplacement Factors Associated with Mental Health of Refugees and Internally Displaced Persons: A Meta-Analysis. JAMA (2005) 294(5):602–12. doi:10.1001/jama.294.5.602
23. Walther, L, Kröger, H, Tibubos, AN, Ta, TMT, Von Scheve, C, Schupp, J, et al. Psychological Distress Among Refugees in Germany: A Cross-Sectional Analysis of Individual and Contextual Risk Factors and Potential Consequences for Integration Using a Nationally Representative Survey, 10. BMJ Open. BMJ Publishing Group (2020). e033658.
24. Spahic-Mihajlovic, A, Crayton, JW, and Neafsey, EJ. Selective Numbing and Hyperarousal in Male and Female Bosnian Refugees with PTSD. J Anxiety Disord (2005) 19(4):383–402. doi:10.1016/j.janxdis.2004.03.004
25. Tibubos, AN, Grammes, J, Beutel, ME, Michal, M, Schmutzer, G, and Brähler, E. Emotion Regulation Strategies Moderate the Relationship of Fatigue with Depersonalization and Derealization Symptoms. J Affect Disord (2018) 227:571–9. doi:10.1016/j.jad.2017.11.079
26. Boyd, M. Family and Personal Networks in International Migration: Recent Developments and New Agendas. Int Migr Rev (1989) 23(3):638–70. doi:10.2307/2546433
27. Silventoinen, K, Hammar, N, Hedlund, E, Koskenvuo, M, Ronnemaa, T, and Kaprio, J. Selective International Migration by Social Position, Health Behaviour and Personality. Eur J Public Health (2007) 18(2):150–5. doi:10.1093/eurpub/ckm052
28. Klugkist, I, and Mulder, J. Bayesian Estimation for Inequality Constrained Analysis of Variance. In: H Hoijtink, I Klugkist, and PA Boelen, editors. Bayesian Evaluation of Informative Hypotheses. New York: Springer (2008). 27.
29. van de Schoot, R, Verhoeven, M, and Hoijtink, H. Bayesian Evaluation of Informative Hypotheses in SEM Using Mplus: A Black bear story. Eur J Dev Psychol (2013) 10(1):81–98. doi:10.1080/17405629.2012.732719
30. Simonsohn, U, Simmons, JP, and Nelson, LD. Specification Curve: Descriptive and Inferential Statistics on All Reasonable Specifications. Available SSRN 2694998. 2019.
31. Steegen, S, Tuerlinckx, F, Gelman, A, and Vanpaemel, W. Increasing Transparency through a Multiverse Analysis. Perspect Psychol Sci (2016) 11(5):702–12. doi:10.1177/1745691616658637
32. Goebel, J, Grabka, MM, Liebig, S, Kroh, M, Richter, D, Schröder, C, et al. The German Socio-Economic Panel (SOEP). In: Jahrbücher für Nationalökonomie und Statistik, 239. De Gruyter Oldenbourg (2019). 345.
33. Hughes, ME, Waite, LJ, Hawkley, LC, and Cacioppo, JT A Short Scale for Measuring Loneliness in Large Surveys: Results from Two Population-Based Studies. Res Aging (2004) 26. 655.
34. Cornwell, EY, and Waite, LJ. Social Disconnectedness, Perceived Isolation, and Health Among Older Adults. J Health Soc Behav (2009) 50(1):31–48. doi:10.1177/002214650905000103
35. Gelman, A, Carlin, JB, Stern, HS, and Rubin, DB. Bayesian Data Analysis. 3rd ed. CRC Press (2014).
36. Martins, TG, Simpson, D, Lindgren, F, and Rue, H. Bayesian Computing with INLA: New Features. Comput Stat Data Anal (2013) 67:68–83. doi:10.1016/j.csda.2013.04.014
37. Hoijtink, H, Klugkist, I, and Boelen, PA. Bayesian Evaluation of Informative Hypotheses. Springer (2008). 361.
38. Orben, A, Dienlin, T, and Przybylski, AK. Social media’s Enduring Effect on Adolescent Life Satisfaction. Proc Natl Acad Sci U S A (2019) 116(21):10226–8. doi:10.1073/pnas.1902058116
Keywords: social isolation, loneliness, mental health, migration, refugees
Citation: Löbel L-M, Kröger H and Tibubos AN (2022) How Migration Status Shapes Susceptibility of Individuals’ Loneliness to Social Isolation. Int J Public Health 67:1604576. doi: 10.3389/ijph.2022.1604576
Received: 01 November 2021; Accepted: 18 November 2022;
Published: 06 December 2022.
Edited by:
Nino Kuenzli, Swiss Tropical and Public Health Institute (Swiss TPH), SwitzerlandCopyright © 2022 Löbel, Kröger and Tibubos. This is an open-access article distributed under the terms of the Creative Commons Attribution License (CC BY). The use, distribution or reproduction in other forums is permitted, provided the original author(s) and the copyright owner(s) are credited and that the original publication in this journal is cited, in accordance with accepted academic practice. No use, distribution or reproduction is permitted which does not comply with these terms.
*Correspondence: Lea-Maria Löbel, bGxvZWJlbEBkaXcuZGU=