- 1State Key Laboratory of Molecular Vaccinology and Molecular Diagnostics, School of Public Health, Xiamen University, Xiamen, China
- 2Center for Aging and Health Research, School of Public Health, Xiamen University, Xiamen, China
Objectives: We examined the magnitude and determinants of socioeconomic disparities in disability-free life expectancy and life expectancy at age 65 (DFLE65 and LE65) in China.
Methods: Data from Chinese Longitudinal Healthy Longevity Survey collected during 2011–2018 (8,184 participants aged ≥65) were used. Socioeconomic status (SES) was measured by economic status (ES), and education, respectively. Multistate Markov models and microsimulations were fitted to estimate DFLE65 and LE65.
Results: LE65 between high- and low-ES groups differed by 2.20 years for males and 2.04 years for females. The DFLE65 disparity in ES was 1.51 and 1.29 years for males and females, respectively. Not undergoing physical examinations, inadequate fruit/vegetable intake, and stress contributed to 35.10% and 57.36% of DFLE65 disparity in ES, as well as 26.36% and 42.65% of LE65 disparity for males and females, respectively. These disparities in education and ES were of a similar magnitude, while the above factors contributed little to education disparity.
Conclusion: Socioeconomic disparities in DFLE65 and LE65 existed in China. Physical examination, fruit/vegetable intake and stress partly explained these disparities.
Introduction
Currently, with a rapidly aging population, more than one billion (approximately 15% of the global population) adults are disabled worldwide [1]. The rate of disability is much higher among older adults [2]. Disability-free life expectancy (DFLE), as a supplement to life expectancy (LE), summarizing disability and mortality experiences, has become an important measure to monitor population health. Many previous studies from high-income countries (HICs) have shown that socioeconomic status (SES), including income, wealth, education and occupation, remains positively associated with LE and DFLE at old ages [3–8]. These socioeconomic inequities in health are inherently unjust and lead to significant financial cost to societies [9, 10]. Reducing such health inequities is a means to improve a whole population’s health and increase healthy aging [11].
As the largest low- and middle-income country (LMIC), China has also confirmed the existence of socioeconomic disparities in DFLE among older adults in 1992–1997 [8]. Since the start of the 21st century, with the accelerated aging, the number of care-dependent Chinese elderly individuals is expected to rise from 25.3 million in 2010 to 66 million in 2050 [12]. Additionally, social security and care systems based on the principles of equitable accessibility and use are undeveloped [13]. This may further aggravate DFLE inequities among older adults. The China-WHO Country Cooperation Strategy 2016–2020 also noted that health inequities will be a key challenge for China in the coming years [14].
However, a recent study from China, using education as an SES indicator, found that the disparity in DFLE at age 65 (DFLE65) between literate and illiterate adults was small (0.2 years for males and 0.0 years for females) [15]. Additionally, in contrast to recent studies from HICs [4–6], this study surprisingly found that the proportion of DFLE65 in the remaining life among illiterate individuals was higher than that among literate individuals [15]. Other recent studies found an education-mortality gradient among Chinese adults aged 65 years and over [16, 17]. These findings may suggest that there is an education disparity in LE (mortality) but not in DFLE among older Chinese adults. In other words, SES may have a relatively stronger effect on mortality than disability at old ages in China. This study did not estimate a 95% confidence interval (95% CI) for the difference in DFLE and its proportion. Whether this difference is statistically significant when further estimating its 95% CI and whether other SES indicators, such as economic status and occupation, show greater inequality of DFLE or provide additional insights compared to education remain unclear.
Estimating the effect of modifiable risk factors on socioeconomic disparities in DFLE and LE enables setting priorities and implementing policies with realistic targets to reduce these disparities. Many studies have shown that inadequate fruit/vegetable intake, smoking, stress, and inadequate healthcare utilization mainly contributed to the socioeconomic disparity in health [18–22]. However, the health indicators in these studies were mostly focused on LE, mortality, self-reported health, etc. To what extent socioeconomic disparity in DFLE can be reduced by reducing or eliminating these risk factors remains unknown. Do the contributions of these factors to these socioeconomic disparities vary by different measures of SES?
Therefore, we estimated the magnitude and potential determinants of socioeconomic disparities in DFLE and LE among older Chinese adults using different measures of SES.
Methods
Study Population
Data from the Chinese Longitudinal Healthy Longevity Survey (CLHLS) [23] were used. The CLHLS is a nationwide population-based longitudinal survey conducted in a sample of randomly selected counties and cities in 23 of the 31 provinces in China. More details about the survey design and data quality are available elsewhere [17, 23]. We used data collected during 2011–2018, which included 3 waves: 2011–2012, 2014, and 2017–2018. The baseline wave (2011–2012) comprised 9,679 participants aged 65 years and over. Individuals without any follow-up after baseline (n = 791), with a negative follow-up time (n = 180) or a missing disability status (n = 499), or without complete data concerning smoking status, fruit/vegetable intake, physical examination, and stress status (n = 25) were excluded from analyses (accounting for a total of 15.45%). Thus, the final analytical sample included 8,184 participants. The CLHLS was approved by the research ethics committees of Duke University and Peking University (IRB00001052–13074).
Socioeconomic Status
Economic status, educational attainment were used to characterize SES. Economic status was measured by the following question: “how do you rate your economic status compared with that of other local people?” and categorized as high (very rich or rich), intermediate (average), or low (poor or very poor). Educational attainment was categorized into low (no schooling), intermediate (1–6 years), and high (7 or more years) by the number of schooling years.
Occupational position was another common indicator being used to assess SES. Occupational position was divided into high (government, institutional and managerial personnel; professional and technical personnel; and military personnel), intermediate (clerks, service industry employees, and manual workers), and low (farmers and the unemployed) according to individuals’ major occupation before age 60. However, about 90% of females in the low occupation and about 81% of males in the low occupation. Occupation may be not much variability in this indicator to pick up exposure to occupational hazards and the like which is what one wants to assess when linking occupation with disability. Therefore, we only displayed the results and discussed the results in discussion, not draw conclusions.
Disability and Disability-Free Life Expectancy
We divided health state into three states: disability-free, disability, and mortality. Disability was defined as needing assistance in at least one of the activities of daily living (ADL): bathing, dressing, going to the toilet, indoor transferring, continence and feeding. DFLE was defined as expected life years without disability.
Demographic Characteristics and Risk Factors
Demographic characteristics and impact factors were assessed at every follow-up wave. Individual characteristics included gender (male/female), age, and region (urban/rural). Risk factors included smoking, inadequate fruit/vegetable intake, not undergoing physical examinations, and feeling stress. Smoking status was categorized as smoking and never smoked, with the former category including both currently and formerly smoking. Fruit/vegetable intake was measured with the following question: “Do you eat fresh fruits or vegetables?” It was categorized as daily/almost daily, occasionally, and rarely/no. Inadequate fruit/vegetable intake included “occasionally” and “rarely/no” intake categories. Not undergoing physical examinations was defined as participants not undergoing a regular physical examination once every year. Stress status was assessed with the following question: “Do you often feel fearful or anxious?” It was categorized as feeling stress (always or often) or no stress (sometimes, seldom, or never).
Statistical Analysis
First, logistic regression models were used to estimate the association of SES with risk factors. A multistate Markov model (MSM) [24] was fitted to estimate the hazard ratios (HRs) for the association of health transitions with SES and risk factors. We fitted 6 MSMs that allowed 4 transitions: from disability-free to disability (disability incidence), from disability-free to death, from disability to disability-free (recovery from disability), and from disability to death. The first model (model 1) adjusted for age, gender, and region. Subsequently, smoking status, fruit/vegetable intake, physical examination status and stress status were entered into model 1 separately as time-dependent covariates (models 2–5) and then simultaneously into model 1 (model 6). In these models, sex-age-region-specific transition probabilities were estimated.
Then, to calculate DFLE65and LE65, we used microsimulation [25] to simulate a cohort of 100,000 persons at age 65. In this 65-year-old cohort, the distribution of region and gender was sourced from the 2010 census data of China [26], and the gender-region-specific distribution of SES and disability was based on the observed prevalence of the CLHLS’s 2011–2012 wave. From age 65 to death, the health and survival trajectories of each individual in this cohort were governed by the transition probabilities output from the MSM model. The 95% CIs (from the 2.5th and 97.5th percentiles) were estimated by bootstrapping with 1,000 independent replications.
Finally, we set the prevalence of the risk factors to zero in all the SES groups (elimination scenario). We compared the results of the elimination scenario to the results of the current situation to quantify the effect of the risk factors on the socioeconomic disparities in DFLE and LE.
We used economic status and educational attainment as measures of SES to conduct the above analysis separately. These analyses were mainly performed using R software (version 3.5.1) and SAS version 9.4.
Results
Characteristics of the Study Population at Baseline
The baseline characteristics of the participants are shown in Table 1. The sample consisted of 3667 males and 4557 females. Of the participants, 19.72%, 26.64%, 28.98% and 24.66% were ages 65–74, 75–84, 84–95, and ≥95 years, respectively. More than half of the participants had low educational attainment (58.82%), only 10.51% had high educational attainment; 15.82% and 17.36% reported low and high economic status, respectively. There were 4349 deaths during 2011–2018. More than a quarter of the older adults were disabled at baseline (26.56%).
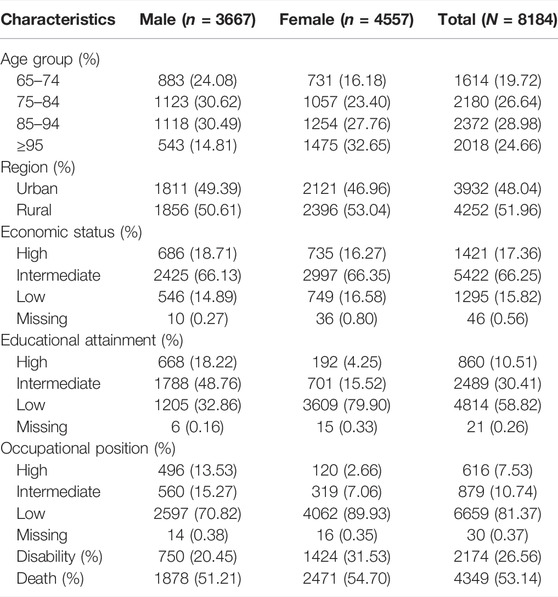
TABLE 1. Sample characteristics, disability, and death in the Chinese Longitudinal Healthy Longevity Survey, 2011–2018 (China, 2011–2018).
Socioeconomic Disparities in DFLE65 and LE65
Socioeconomic disparities in DFLE65 and LE65 are displayed in Table 2. A positive socioeconomic gradient was seen in LE65 as well as DFLE65 when using economic status and educational attainment as measures of SES. LE65 between the high and low economic status groups differed by 2.20 (95% CI, 1.10–3.41) years for males and 2.04 (1.01–3.29) years for females. The DFLE65 disparity in economic status was 1.51 (0.52–2.59) and 1.29 (0.19–2.52) years for males and females, respectively. Similarly, the LE65 disparity between the high- and low-education groups was 2.28 (1.11–3.46) years for males and 1.79 (0.66–3.07) years for females. For DFLE65, the education disparity was 1.88 (0.74–2.93) years for males and 1.32 (0.13–2.60) years for females. Overall, the proportion of DFLE65 was lower in the high-SES group than in the low-SES group using economic status and educational attainment as markers of SES, although the difference in education was not statistically significant.
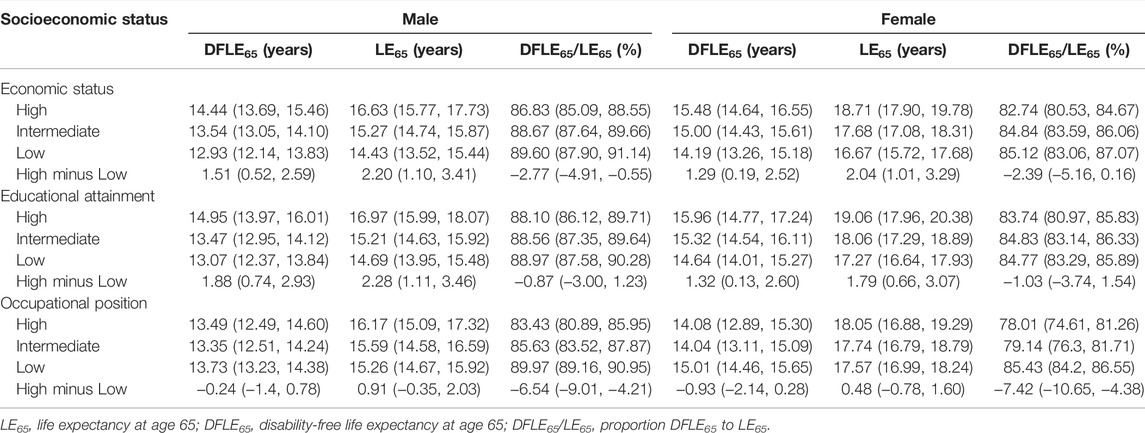
TABLE 2. Disability-free life expectancy and life expectancy with 95% confidence interval according to socioeconomic status for males and females, based on the Chinese Longitudinal Healthy Longevity Survey, 2011–2018 (China, 2011–2018).
Socioeconomic Disparities in Prevalence of Risk Factors
The prevalence of risk factors according to SES is presented in Figure 1. Economic status and educational attainment were negatively associated with the prevalence of inadequate fruit/vegetable intake (p < 0.05), whereas only economic status was negatively related to the prevalence of feeling stress (p < 0.001). Lower economic status and educational attainment were associated with higher prevalence of not undergoing physical examinations (p < 0.05). Overall, there was little socioeconomic difference in the prevalence of smoking.
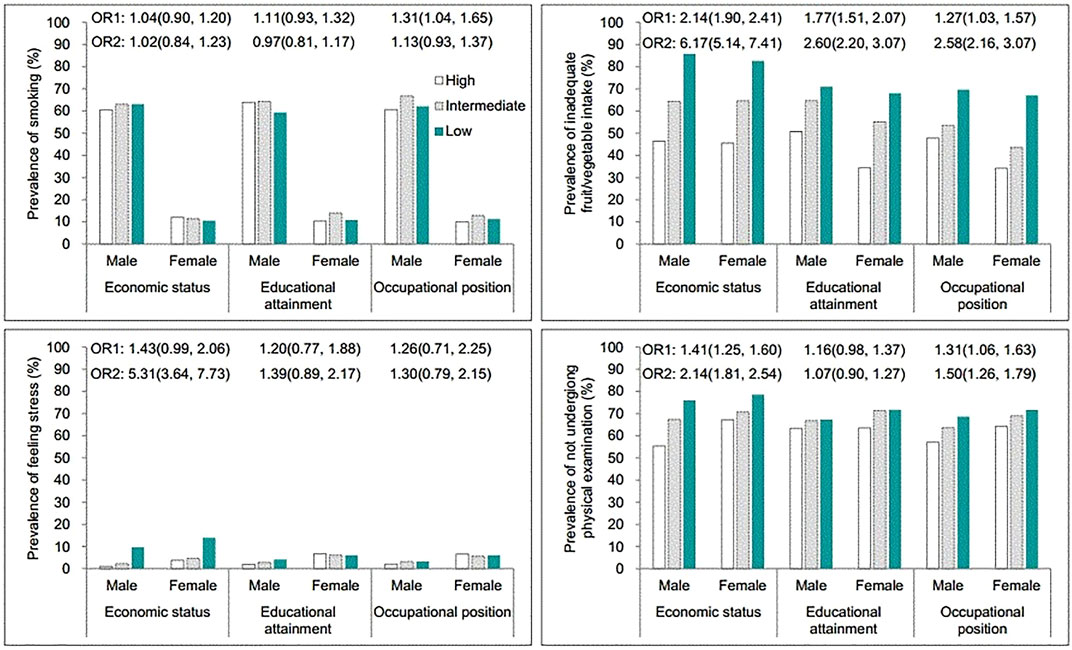
FIGURE 1. Prevalence of risk factors according to socioeconomic status for older males and females aged 65 years and over, based on the Chinese Longitudinal Healthy Longevity Survey, 2011–2018 (China, 2011–2018).
Association of Transitions With Risk Factors
Smoking was the risk factor for disability incidence (HR = 1.20, 95% CI = 1.01–1.43) (Table 3). For mortality from disability-free, not undergoing physical examination was the risk factor (HR = 1.25, 95% CI = 1.05–1.53). For mortality from disability, feeling stress, not undergoing physical examinations, the occasional and rare/no intake of fruit/vegetable were the risk factors, and the HRs (95% CI) were 1.27 (1.08–1.49), 1.11 (1.01–1.22), 1.15 (1.03–1.28), and 1.28 (1.16–1.43), respectively.
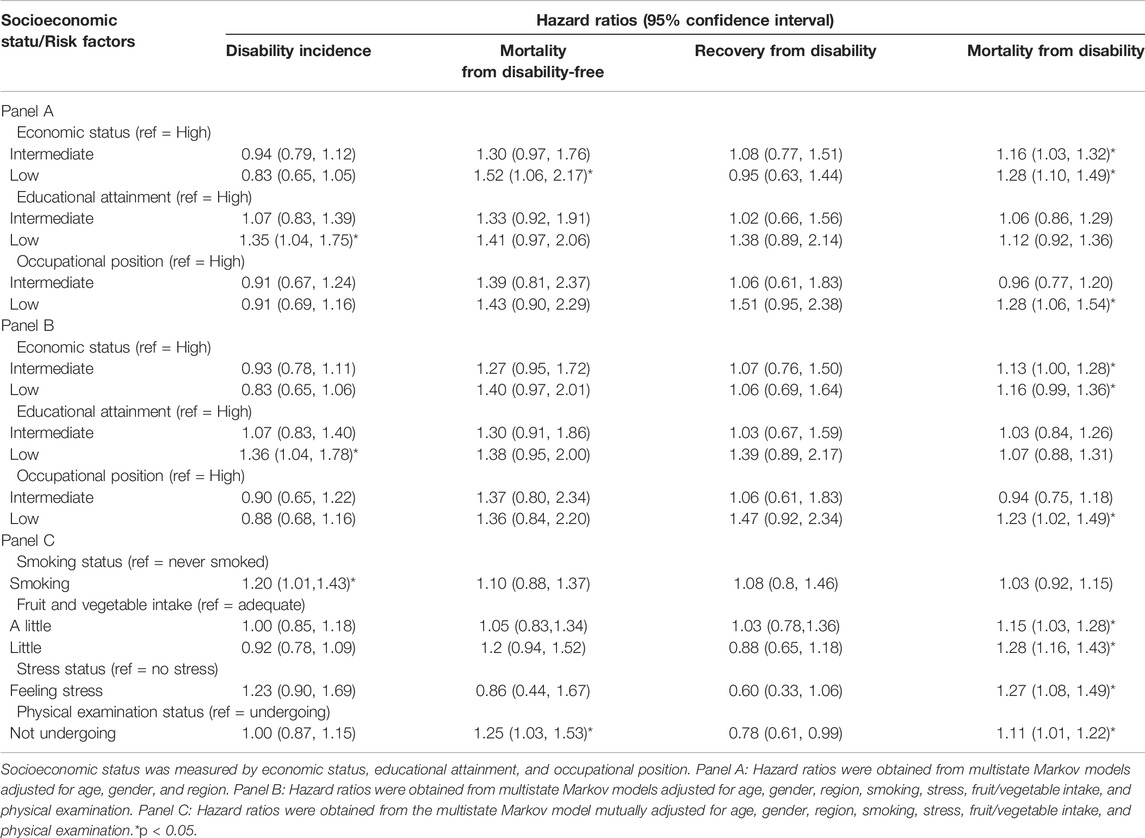
TABLE 3. The association of transitions with socioeconomic status and risk factors for older adults aged 65 years and over, based on the Chinese Longitudinal Healthy Longevity Survey, 2011–2018 (China, 2011–2018).
Association of Transitions With SES
The association of transitions with SES and the risk factors are shown in Table 3. For mortality from disability-free, the HR (95% CI) for low economic status (high as reference) was 1.52 (1.06–2.17) in the model adjusted for age, gender, and region. After adjusting smoking, fruit/vegetable intake, physical examination, and stress, the HR was attenuated to 1.40 (0.97–2.01). Similarly, for mortality from disability, after adjusting these factors, the HR for low economic status decreased from 1.28 (1.10–1.49) to 1.16 (0.99–1.36), and the HR for intermediate economic status slightly decreased from 1.16 (1.03–1.32) to 1.13 (1.00–1.28). The association of economic status with disability incidence and recovery had no statistical significance (p > 0.05).
Low education was only associated with disability incidence (HR = 1.36, 95% CI = 1.04–1.78). After adjusting for the above factors, the HRs changed little.
Contribution of Risk Factors to Socioeconomic Disparities in DFLE65 and LE65
The DFLE65 and LE65 disparities in economic status were substantially reduced by eliminating not undergoing physical examinations or inadequate fruit/vegetable intake and somewhat reduced by eliminating feeling stress, but these disparities changed little by eliminating smoking (Figure 2). For example, the DFLE65 disparity between males with high and low economic status was reduced from 1.51 (0.52–2.59) years to 1.08 (0.04–2.34) years by eliminating inadequate fruit/vegetable intake. That is, 28.48% of the DFLE65 disparity in economic status for males was attributed to inadequate fruit/vegetable intake. Inadequate fruit/vegetable intake, not undergoing physical examinations, and stress contributed in total to 35.10% of the DFLE65 disparity in economic status for males and 57.36% for females. The contribution from these factors to the LE65 disparity in economic status was 26.36% and 42.65% for males and females, respectively. However, the contribution of these risk factors to education disparities in DFLE65 and LE65 was small (approximately 10%) (Table 4).
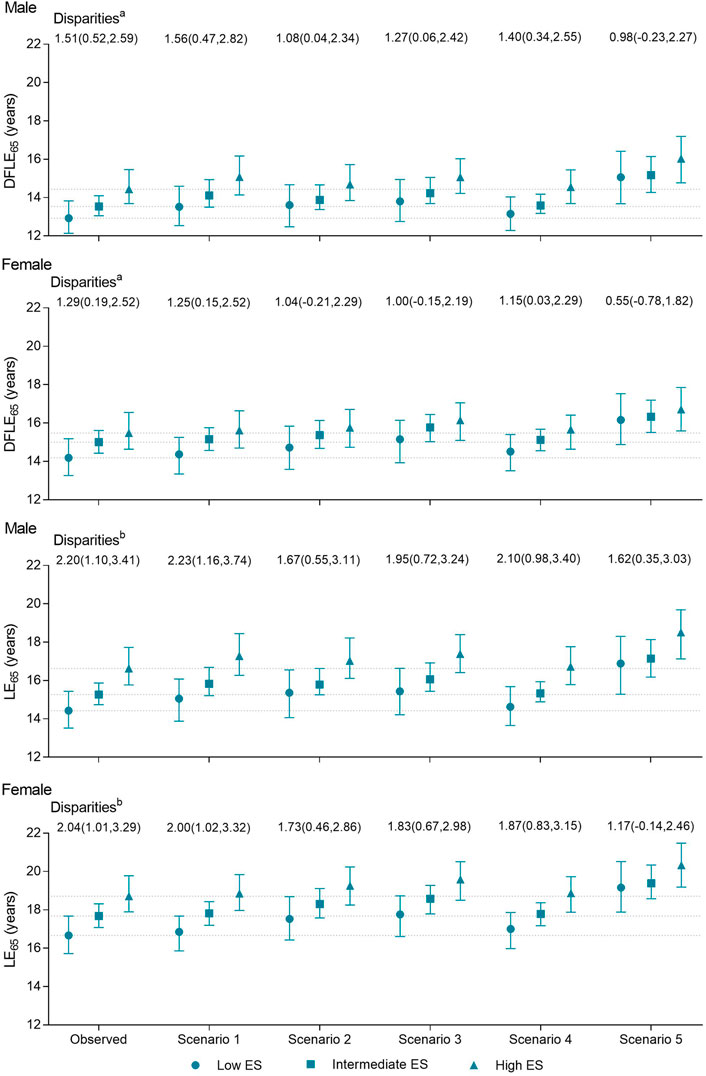
FIGURE 2. Observed and scenario socioeconomic disparities in disability-free life expectancy and life expectancy, based on the Chinese Longitudinal Healthy Longevity Survey, 2011–2018 (China, 2011–2018).
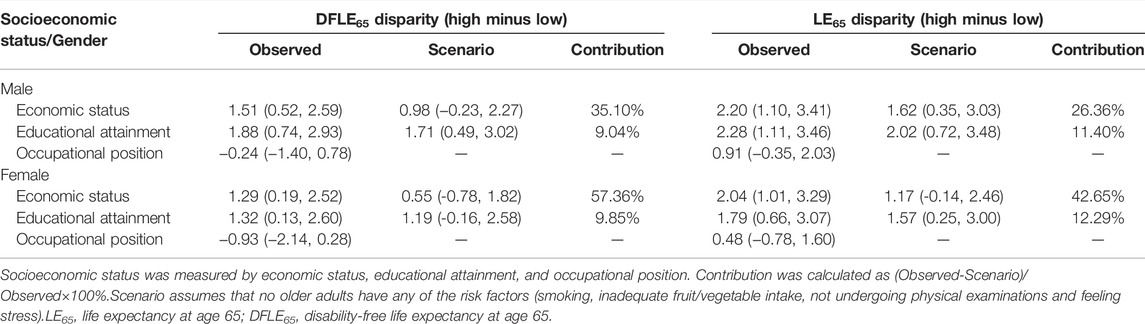
TABLE 4. Socioeconomic disparities in disability-free life expectancy and life expectancy with 95% confidence interval, based on the Chinese Longitudinal Healthy Longevity Survey, 2011–2018 (China, 2011–2018).
Discussion
The results yield 4 main findings. First, socioeconomic disparity in DFLE65 and LE65 existed in China in 2011–2018. The magnitudes of these disparities were similar when using economic status and education as markers of SES. Second, the socioeconomic disparity in LE65 was larger than that in DFLE65, and the higher-SES group had a lower proportion of DFLE65 to LE65. Third, economic status was negatively associated with mortality at old ages, but educational attainment was negatively related to disability incidence. Fourth, inadequate fruit/vegetable intake, not undergoing physical examinations and stress played important mediating roles in the DFLE65 and LE65 disparities in economic status but not in this disparity in education.
In contrast to another Chinese study (3-year follow-up) that found little DFLE65 disparity in education [15], our results showed a greater education-DFLE65 gradient. This inconsistency may be because our study had a longer follow-up time (7 years). Studies on socioeconomic disparities in DFLE and LE at old ages have been reported in other countries. In Denmark, the DFLE65 disparities between tertiary education and primary/lower secondary education were 2.9 and 3.4 years for males and females in 2014, respectively; for LE65, the disparities were 2.4 and 2.2 years [6]. The income disparities (highest and lowest income quintiles) in DFLE65 (6.1 years for males and 5.6 years for females) and LE65 (4.7 years for males and 3.3 years for females) seemed larger than the education disparities [4]. In England and the United States, the socioeconomic disparities in DFLE were larger for wealth than for education and occupation [3]. The disparities between the richest (in the top 33% of wealth) and poorest (in the bottom 33% of wealth) were 7–8 years at age 60 and 6–7 years at age 70 in 2002–2013 [3]. In 10 western European countries, the average education difference (lower secondary education or lower versus tertiary education) in DFLE65 was 4.6 years for males and 4.4 years for females; the average LE65 disparity was 3.0 years for males and 1.9 years for females in 1995–2001 [5]. In Japan, the education difference (≤9 years of formal education versus >9 years of formal education) in DFLE65 was 2.4–2.5 years, and in LE65 it was 2.1 years for people who were active at baseline in 1999–2009 [27]. Although our results cannot be directly compared with the results from these studies because of different measures of SES and disability, the magnitudes of DFLE65 and LE65 disparities in economic status and education in China appeared similar to those in Japan but smaller than those in western developed countries.
Unlike economic status and education, occupational position seemed to be negatively associated with DFLE65, although the association was not statistically significant. Furthermore, higher occupational position was related to higher disability prevalence (Supplementary Figure S1). There are two plausible explanations for these puzzling findings. First, most of the older adults with low occupational positions were farmers and still needed to do farm work to survive at older ages, which may contribute to better physical functioning. A study revealed that farm work was negatively related to dependency duration in late life [28]. Second, older adults with low occupational positions had higher mortality from disability. The mortality selection made older adults with low occupational positions pass away at early ages, and the people who survived to old ages had healthier physical functioning [29]. Occupational position was categorized into 3 groups (high, intermediate, low) with about 90% of females in the low occupation and about 81% of males in the low occupation, which may lead to an underestimation of the effect.
Consistent with another recent study from China [15], the proportion of DFLE65 was lower in the higher-education group. However, it lost statistical significance when we further estimated a 95% CI. However, we found that the proportion of DFLE65 was lower in the higher-SES group when using economic status as markers of SES and that the disparity in LE65 was larger than that in DFLE65. Moreover, economic status were associated with mortality but not with disability incidence and recovery (Table 3). These results suggested that SES had a stronger effect on mortality than disability at old ages in China. The lower mortality in the lower-SES group merits further study.
Additionally, economic status was negatively associated with disability prevalence at age 65 (Supplementary Figure S1). These results indicated that the effect of economic status on disability may mainly occur in early life rather than in late life. A Netherlands study showed that the average age at onset of disability in a low-SES group (62 years for males and 61 years for females) was younger than that in a high-SES group (76 years for males and 75 years for females) [30]. Implementing relevant policies targeting early-life disability and late-life mortality among those with low SES may greatly improve DFLE and reduce health inequities in later life.
Unlike in some countries where smoking has a great effect on health disparities [31–33], smoking had little effect on DFLE65 and LE65 disparities in SES in China. Consistent with previous studies, stress was an important mediator of the association between economic status and health [21, 34]. However, the effect of stress on the DFLE65 and LE65 disparities in economic status was small for its relatively lower prevalence among older adults. It was inadequate fruit/vegetable intake and not undergoing physical examinations that were the determinants of these disparities in economic status and should be given priority when making policies to reduce the inequities in DFLE and LE.
However, inadequate fruit/vegetable intake, not undergoing physical examinations and stress had little effect on the educational disparities. Unlike economic status, educational attainment seemed to affect DFLE65 and LE65 through different paths. Educational attainment was negatively associated with disability incidence but not mortality at old ages. A previous study of 7 LIMCs showed that approximately two-thirds of disabilities were attributed to chronic disease [35]. Another study of 20 HICs and LIMCs revealed that all-cause mortality and cardiovascular events were negatively associated with education but not with health [36]. These findings may suggest that educational attainment may affect DFLE65 and LE65 through chronic disease. In addition, the older adults with higher education may get a better job or better know the rules of cardiovascular prevention, such as reducing the consumption of salt or cigarette smoke or body weight, to have better health outcomes.
Strengths and Limitations
Our study provided updated results on the socioeconomic disparities in DFLE and LE among older adults using different measures of SES in the 2010s in an LMIC. Moreover, the SES and risk factors may consequently change with time. Therefore, we used repeated measures of SES and risk factors as time-dependent variables to estimate the effect of risk factors on this disparity. A study has suggested that the effect of risk factors will be underestimated if only risk factors are assessed at the first follow-up [33].
However, there are some limitations. First, risk factors were self-reported and categorized broadly on two or three levels, which potentially underestimated their effects. Second, we did not use income or wealth to assess SES due to its potential multiple sources (e.g., cumulative income throughout life, monetary assistance from family members and various sideline economic activities) and the difficulty of obtaining accurate accounts. Second, economic status was self-perceived and subjective. However, subjective SES, such as self-perceived economic status and adequacy of income, has also been used to assess the association of SES with health in previous studies [37–39]. Subjective SES has been thought to capture differences in wealth [40]. Furthermore, self-perceived economic status may have been more related to relative SES within the respondents’ region. Some of the high-SES people from rural areas, for instance, may have had lower absolute SES than intermediate-SES people from the cities. Local governments may find it effective to make scientific decisions targeted to relatively poor older adults within their regions. Third, losses to follow-up and from nonresponse may have biased our results. The excluded participants tended to live in rural areas and have lower economic status than the eligible participants, but the difference was small (Supplementary Table S1). Fourth, confounding factors that were unknown or not included in the analysis, such as moderate or heavy drinking, may have contributed to an overestimation of outcomes. However, it was difficult to obtain alcohol consumption in our data. Finally, the MSM model we fitted based on the Markov process assumed that the transitions between health statuses depended only on the present status, not on the sequence of events that preceded it and thus did not account for individual heterogeneity in disability status history.
In conclusion, DFLE65 and LE65 disparities existed in economic status and education. Moreover, the LE65 disparity was greater than the DFLE65 disparity. The mechanisms of the DFLE65 and LE65 disparities in economic status and education differed. Physical examinations, fruit/vegetable intake and stress had great effects on DFLE65 and LE65 disparities in economic status but not in education. Future policies should pay more attention to older adults’ economic status and education and use different interventions for different groups.
Ethics Statement
The studies involving human participants were reviewed and approved by the research ethics committees of Duke University and Peking University (IRB00001052–13074). The patients/participants provided their written informed consent to participate in this study.
Author Contributions
Concept and design: YZ, YH, and YF. Analysis of the data and drafting the manuscript: YZ. Critical revision of the manuscript: YH, YF, and YZ. All authors have seen and approved the final version.
Funding
This study was supported by a project of the National Natural Science Foundation of China (No. 81973144) and the Natural Science Foundation of Fujian Province (No. 2019J01038). The funder had no role in any of the work described in the manuscript.
Conflict of Interest
The authors declare that the research was conducted in the absence of any commercial or financial relationships that could be construed as a potential conflict of interest.
Supplementary Material
The Supplementary Material for this article can be found online at: https://www.ssph-journal.org/articles/10.3389/ijph.2022.1604242/full#supplementary-material
Supplementary Figure S1 | Disability prevalence across socioeconomic status (China, 2011–2018).
References
1.World Health Organization. Draft WHO Global Disability Action Plan 2014–2021: Better Health for All People with Disabilities. Geneva: World Health Organization (2014).
2.World Health Organization, The World Bank. World Report on Disability. Geneva: World Health Organisation (2015).
3. Paola, Z, David, BG, Sari, S, Ichiro, K, Martin, H, Marcel, G, et al. Socioeconomic Inequalities in Disability-free Life Expectancy in Older People from England and the United States: A Cross-National Population-Based Study. J Gerontol A Biol Sci Med Sci (2020) 75:906. doi:10.1093/gerona/glz266
4. Brønnum-Hansen, H, Foverskov, E, and Andersen, I. Income Inequality in Life Expectancy and Disability-free Life Expectancy in Denmark. J Epidemiol Community Health (2020) 75:145. doi:10.1136/jech-2020-214108
5. Majer, IM, Nusselder, WJ, Mackenbach, JP, and Kunst, AE. Socioeconomic Inequalities in Life and Health Expectancies Around Official Retirement Age in 10 Western-European Countries. J Epidemiol Community Health (2011) 65:972–9. doi:10.1136/jech.2010.111492
6. Brønnum-Hansen, H, Eriksen, ML, Andersen-Ranberg, K, and Jeune, B. Persistent Social Inequality in Life Expectancy and Disability-free Life Expectancy: Outlook for a Differential Pension Age in Denmark? Scand J Public Health (2017) 45:459–62. doi:10.1177/1403494816683591
7. Vanessa, Y, and Yasuhiko, S. Are There Education Differentials in Disability and Mortality Transitions and Active Life Expectancy Among Japanese Older Adults? Findings from a 10-Year Prospective Cohort Study. J Gerontol B Psychol Soc (2012) 67:343–53. doi:10.1093/geronb/gbs029
8. Kaneda, T, Zimmer, Z, and Tang, Z. Socioeconomic Status Differentials in Life and Active Life Expectancy Among Older Adults in Beijing. Disabil Rehabil (2005) 27:241–51. doi:10.1080/09638280400006481
9. Marmot, M, Friel, S, Bell, R, Houweling, TA, and Taylor, S. Closing the gap in a Generation: Health Equity through Action on the Social Determinants of Health. Lancet (2008) 372:1661–9. doi:10.1016/s0140-6736(08)61690-6
10. Mackenbach, JP, Meerding, WJ, and Kunst, AE. Economic Costs of Health Inequalities in the European Union. J Epidemiol Community Health (2011) 65:412–9. doi:10.1136/jech.2010.112680
11.World Health Organization. CHINA-WHO Country Cooperation Strategy 2016–2020. Geneva: World Health Organisation (2015).
12.World Health Organization. China Country Assessment Report on Ageing and Health. Geneva: World Health Organisation (2015).
13. Wang, XQ, and Chen, PJ. Population Ageing Challenges Health Care in China. Lancet (2014) 383:870. doi:10.1016/S0140-6736(14)60443-8
14.World Health Organization Regional Office for the Western Pacific. China-WHO Country Cooperation Strategy 2016–2020. WHO Regional Office for the Western Pacific (2016). Available from: https://apps.who.int/iris/handle/10665/206614.
15. Huang, G, Guo, F, and Chen, G. Educational Differences of Healthy Life Expectancy Among the Older Adults in China: A Multidimensional Examination Using the Multistate Life Table Method. Educ Gerontol (2019) 45:1–12. doi:10.1080/03601277.2019.1678719
16. Sudharsanan, N, Zhang, Y, Payne, CF, Dow, W, and Crimmins, E. Education and Adult Mortality in Middle-Income Countries: Surprising Gradients in Six Nationally-Representative Longitudinal Surveys. SSM Popul Health (2020) 12:100649. doi:10.1016/j.ssmph.2020.100649
17. Luo, Y, Zhang, Z, and Gu, D. Education and Mortality Among Older Adults in China. Soc Sci Med (2015) 127:134–42. doi:10.1016/j.socscimed.2014.09.039
18. Mäki, NE, Martikainen, PT, Eikemo, T, Menvielle, G, Lundberg, O, Östergren, O, et al. The Potential for Reducing Differences in Life Expectancy between Educational Groups in Five European Countries: the Effects of Obesity, Physical Inactivity and Smoking. J Epidemiol Community Health (2014) 68:635–40. doi:10.1136/jech-2013-203501
19. Stringhini, S. Association of Socioeconomic Position with Health Behaviors and Mortality. JAMA (2010) 303:1159–66. doi:10.1001/jama.2010.297
20. Aldabe, B, Anderson, R, Lyly-Yrjanainen, M, Parent-Thirion, A, Vermeylen, G, Kelleher, CC, et al. Contribution of Material, Occupational, and Psychosocial Factors in the Explanation of Social Inequalities in Health in 28 Countries in Europe. J Epidemiol Community Health (2011) 65:1123–31. doi:10.1136/jech.2009.102517
21. Adler, NE, and Stewart, J. Health Disparities across the Lifespan: Meaning, Methods, and Mechanisms. Ann N Y Acad Sci (2010) 1186:5–23. doi:10.1111/j.1749-6632.2009.05337.x
22. Baars, AE, Rubio-Valverde, JR, Hu, Y, Bopp, M, Brønnum-Hansen, H, Kalediene, R, et al. Fruit and Vegetable Consumption and its Contribution to Inequalities in Life Expectancy and Disability-free Life Expectancy in Ten European Countries. Int J Public Health (2019) 64:861–72. doi:10.1007/s00038-019-01253-w
23.Duke University. Chinese Longitudinal Healthy Longevity Survey (CLHLS). [Internet]. Beijing: Duke University Center for the Study of Aging and Human Development (1998). Available from: https://sites.duke.edu/centerforaging/programs/chinese-longitudinal-healthy-longevity-survey-clhls/.
24. Hou, C, Lin, Y, Ren, M, Liu, M, Ma, Y, Li, H, et al. Cognitive Functioning Transitions, Health Expectancies, and Inequalities Among Elderly People in China: A Nationwide Longitudinal Study. Int J Geriatr Psychiatry (2018) 33:1–10. doi:10.1002/gps.4966
25. Cai, L, Hayward, M, Saito, Y, Lubitz, J, Hagedorn, A, and Crimmins, E. Estimation of Multi-State Life Table Functions and Their Variability from Complex Survey Data Using the SPACE Program. DemRes (2010) 22:129–58. doi:10.4054/demres.2010.22.6
26.Population Census Office of the State Council of PRC, Department of Population and Employment Statistics National Bureau of Statistics Ministry of PRC. Tabulation on 2010 Population Census of the People's Republic of China. Beijing: China Statistics Press (2010).
27. Yong, V, and Saito, Y. Are There Education Differentials in Disability and Mortality Transitions and Active Life Expectancy Among Japanese Older Adults? Findings from a 10-year Prospective Cohort Study. J Gerontol Ser B: Psychol Sci Soc Sci (2012) 67B:343–53. doi:10.1093/geronb/gbs029
28. Haruyama, K, Yokomichi, H, and Yamagata, Z. Farm Working Experience Could Reduce Late-Life Dependency Duration Among Japanese Older Adults: The Yamanashi Healthy-Active Life Expectancy Cohort Study Based on the STROBE Guidelines. Medicine (Baltimore) (2020) 99:e22248–7. doi:10.1097/MD.0000000000022248
29. Manton, KG, and Stallard, E. Methods for Evaluating the Heterogeneity of Aging Processes in Human Populations Using Vital Statistics Data: Explaining the Black/white Mortality Crossover by a Model of Mortality Selection. Hum Biol (1981) 53:47–67.
30. Knoops, K, and van den Brakel, M. Rijke mensen leven lang en gezond. TVGW (2010) 88:17–24. doi:10.1007/bf03089530
31. Östergren, O, Martikainen, P, Tarkiainen, L, Elstad, JI, and Brønnum-Hansen, H. Contribution of Smoking and Alcohol Consumption to Income Differences in Life Expectancy: Evidence Using Danish, Finnish, Norwegian and Swedish Register Data. J Epidemiol Community Health (2019) 73:334–9. doi:10.1136/jech-2018-211640
32. Stringhini, S, Dugravot, A, Shipley, M, Goldberg, M, Zins, M, Kivimäki, M, et al. Health Behaviours, Socioeconomic Status, and Mortality: Further Analyses of the British Whitehall II and the French GAZEL Prospective Cohorts. Plos Med (2011) 8:e1000419. doi:10.1371/journal.pmed.1000419
33. Stringhini, S, Sabia, S, Shipley, M, Brunner, E, Nabi, H, Kivimaki, M, et al. Association of Socioeconomic Position with Health Behaviors and Mortality. JAMA (2010) 303:1159–66. doi:10.1001/jama.2010.297
34. Lantz, PM, House, JS, Mero, RP, and Williams, DR. Stress, Life Events, and Socioeconomic Disparities in Health: Results from the Americans' Changing Lives Study. J Health Soc Behav (2005) 46:274–88. doi:10.1177/002214650504600305
35. Sousa, RM, Ferri, CP, Acosta, D, Albanese, E, Guerra, M, Huang, Y, et al. Contribution of Chronic Diseases to Disability in Elderly People in Countries with Low and Middle Incomes: A 10/66 Dementia Research Group Population-Based Survey. Lancet (2009) 374:1821–30. doi:10.1016/s0140-6736(09)61829-8
36. Rosengren, A, Smyth, A, Rangarajan, S, Ramasundarahettige, C, Bangdiwala, SI, AlHabib, KF, et al. Socioeconomic Status and Risk of Cardiovascular Disease in 20 Low-Income, Middle-Income, and High-Income Countries: The Prospective Urban Rural Epidemiologic (PURE) Study. Lancet Glob Health (2019) 7:e748–e760. doi:10.1016/s2214-109x(19)30045-2
37. Feng, D, Ji, L, and Xu, L. Effect of Subjective Economic Status on Psychological Distress Among Farmers and Non-farmers of Rural China. Aust J Rural Health (2015) 23:215–20. doi:10.1111/ajr.12187
38. Karvonen, S, and Rahkonen, O. Subjective Social Status and Health in Young People. Sociol Health Illness (2011) 33:372–83. doi:10.1111/j.1467-9566.2010.01285.x
39. Zhou, W, Chen, R, Hopkins, A, Wang, Y, Tang, J, Chen, X, et al. Association between Socioeconomic Status and Incident Stroke in China. J Epidemiol Community Health (2020) 74:519. doi:10.1136/jech-2019-213515
Keywords: older adults, occupation, education, life expectancy, disability-free life expectancy, socioeconomic disparities, economic status
Citation: Zhan Y, Han Y and Fang Y (2022) Socioeconomic Disparities in Disability-Free Life Expectancy and Life Expectancy Among Older Chinese Adults From a 7-Year Prospective Cohort Study. Int J Public Health 67:1604242. doi: 10.3389/ijph.2022.1604242
Received: 12 May 2021; Accepted: 25 May 2022;
Published: 07 July 2022.
Edited by:
Salvatore Panico, University of Naples Federico II, ItalyReviewed by:
Pietro Amedeo Modesti, University of Florence, ItalyCopyright © 2022 Zhan, Han and Fang. This is an open-access article distributed under the terms of the Creative Commons Attribution License (CC BY). The use, distribution or reproduction in other forums is permitted, provided the original author(s) and the copyright owner(s) are credited and that the original publication in this journal is cited, in accordance with accepted academic practice. No use, distribution or reproduction is permitted which does not comply with these terms.
*Correspondence: Ya Fang, fangya@xmu.edu.cn